Keyphrase Generation for Scientific Document Retrieval
Florian Boudin, Ygor Gallina, Akiko Aizawa
Information Retrieval and Text Mining Short Paper
Session 2A: Jul 6
(08:00-09:00 GMT)
Session 3A: Jul 6
(12:00-13:00 GMT)
Abstract:
Sequence-to-sequence models have lead to significant progress in keyphrase generation, but it remains unknown whether they are reliable enough to be beneficial for document retrieval. This study provides empirical evidence that such models can significantly improve retrieval performance, and introduces a new extrinsic evaluation framework that allows for a better understanding of the limitations of keyphrase generation models. Using this framework, we point out and discuss the difficulties encountered with supplementing documents with -not present in text- keyphrases, and generalizing models across domains. Our code is available at https://github.com/boudinfl/ir-using-kg
You can open the
pre-recorded video
in a separate window.
NOTE: The SlidesLive video may display a random order of the authors.
The correct author list is shown at the top of this webpage.
Similar Papers
EVIDENCEMINER: Textual Evidence Discovery for Life Sciences
Xuan Wang, Yingjun Guan, Weili Liu, Aabhas Chauhan, Enyi Jiang, Qi Li, David Liem, Dibakar Sigdel, John Caufield, Peipei Ping, Jiawei Han,
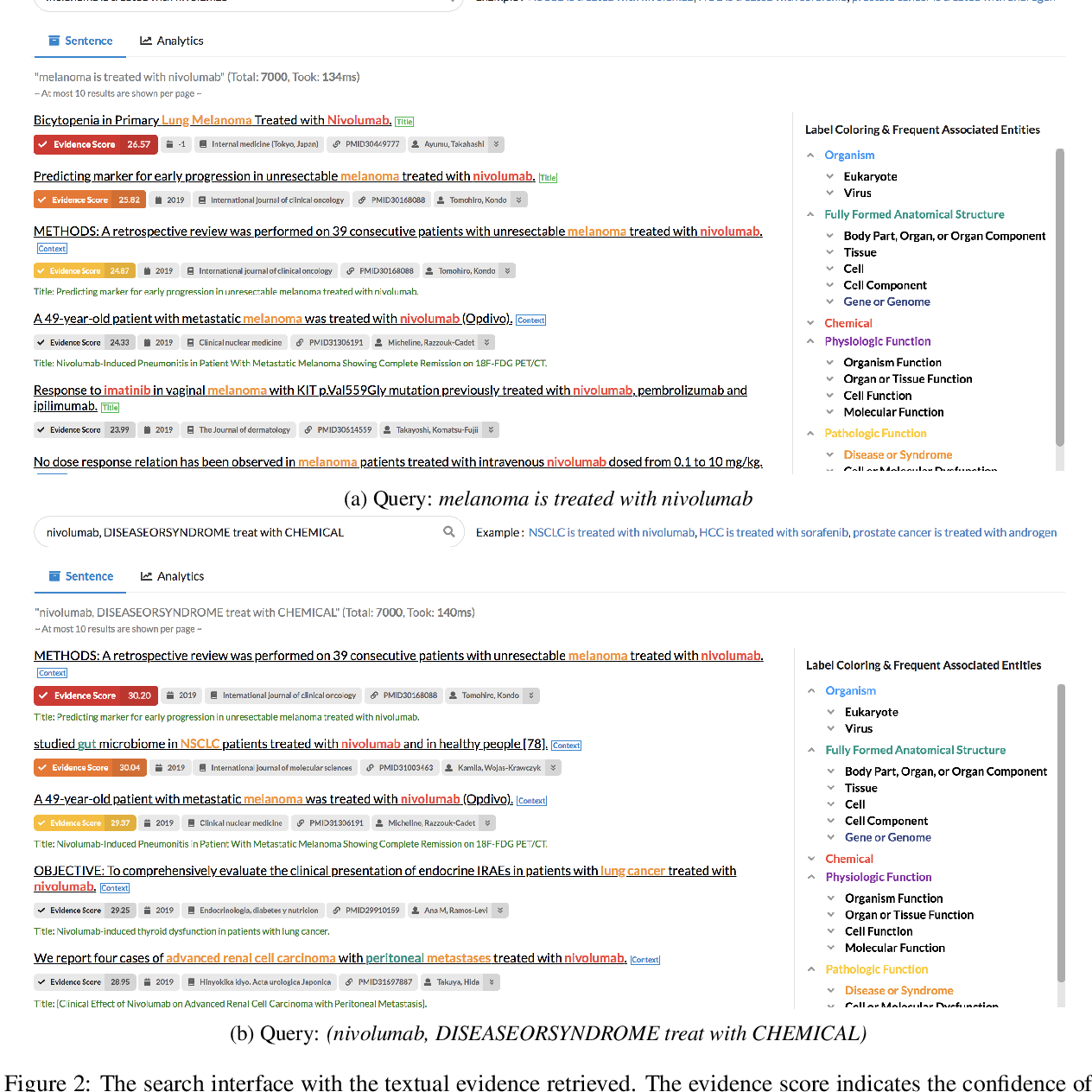
Expertise Style Transfer: A New Task Towards Better Communication between Experts and Laymen
Yixin Cao, Ruihao Shui, Liangming Pan, Min-Yen Kan, Zhiyuan Liu, Tat-Seng Chua,
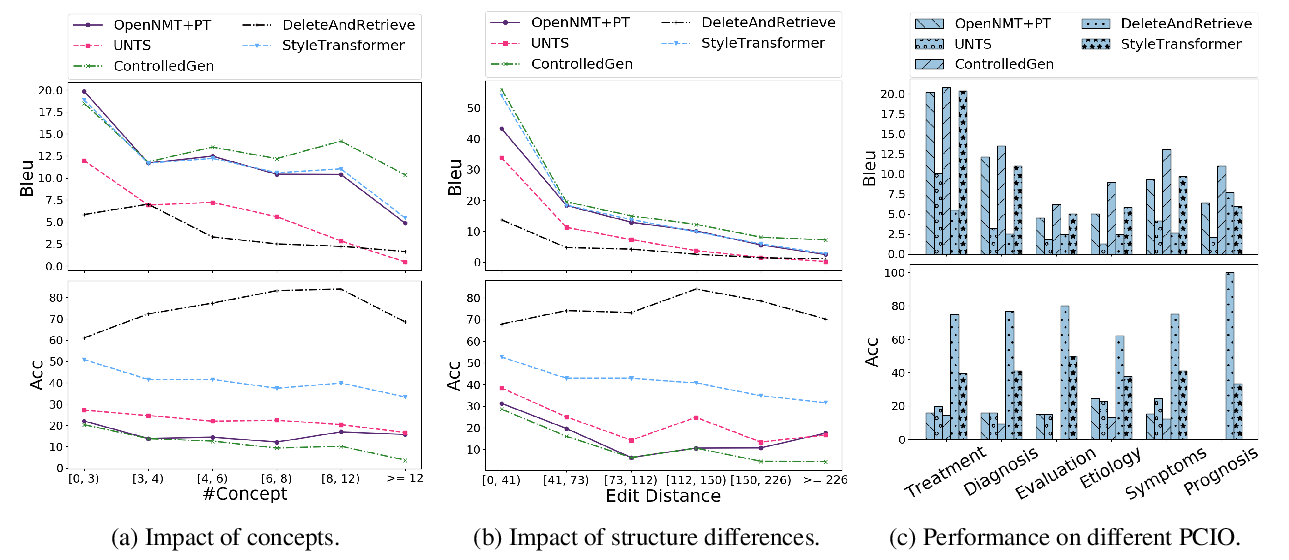
Incorporating External Knowledge through Pre-training for Natural Language to Code Generation
Frank F. Xu, Zhengbao Jiang, Pengcheng Yin, Bogdan Vasilescu, Graham Neubig,
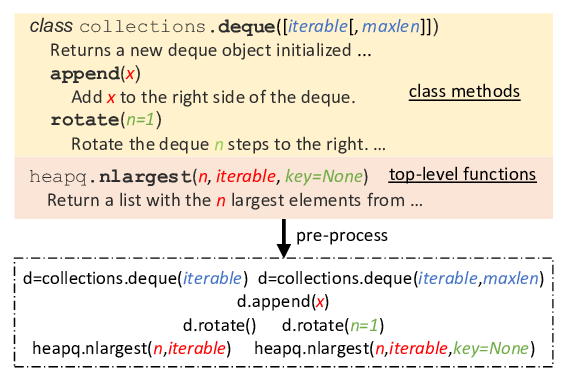
Personalized PageRank with Syntagmatic Information for Multilingual Word Sense Disambiguation
Federico Scozzafava, Marco Maru, Fabrizio Brignone, Giovanni Torrisi, Roberto Navigli,
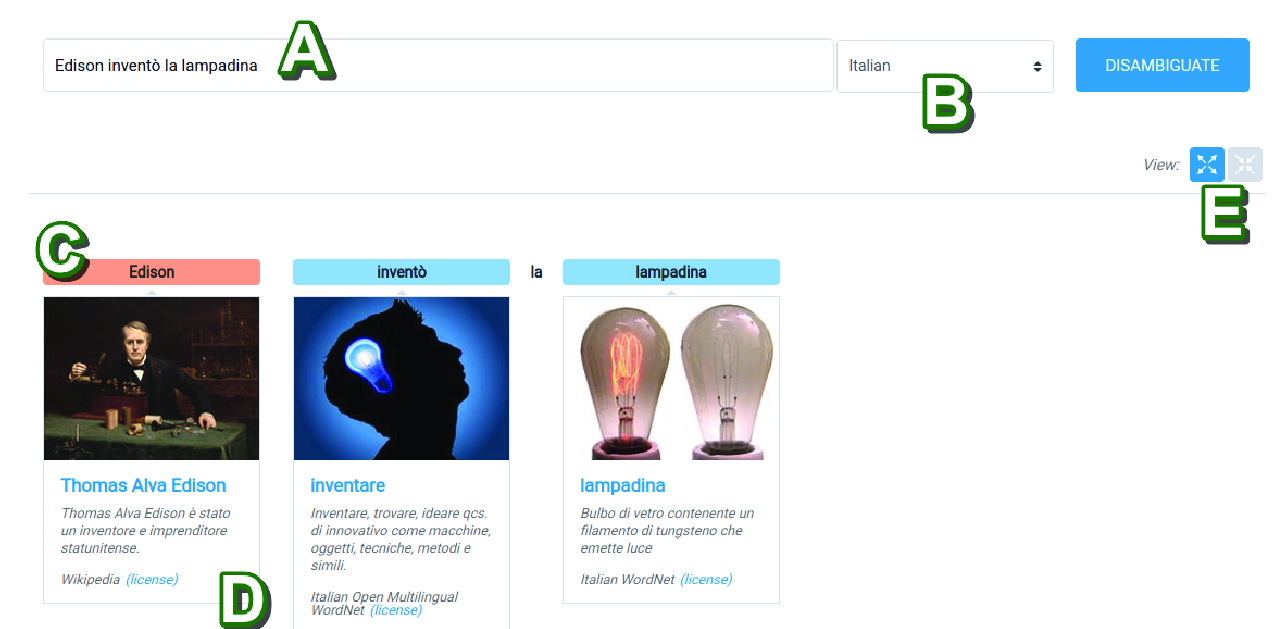