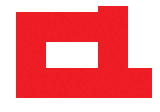
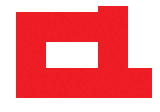
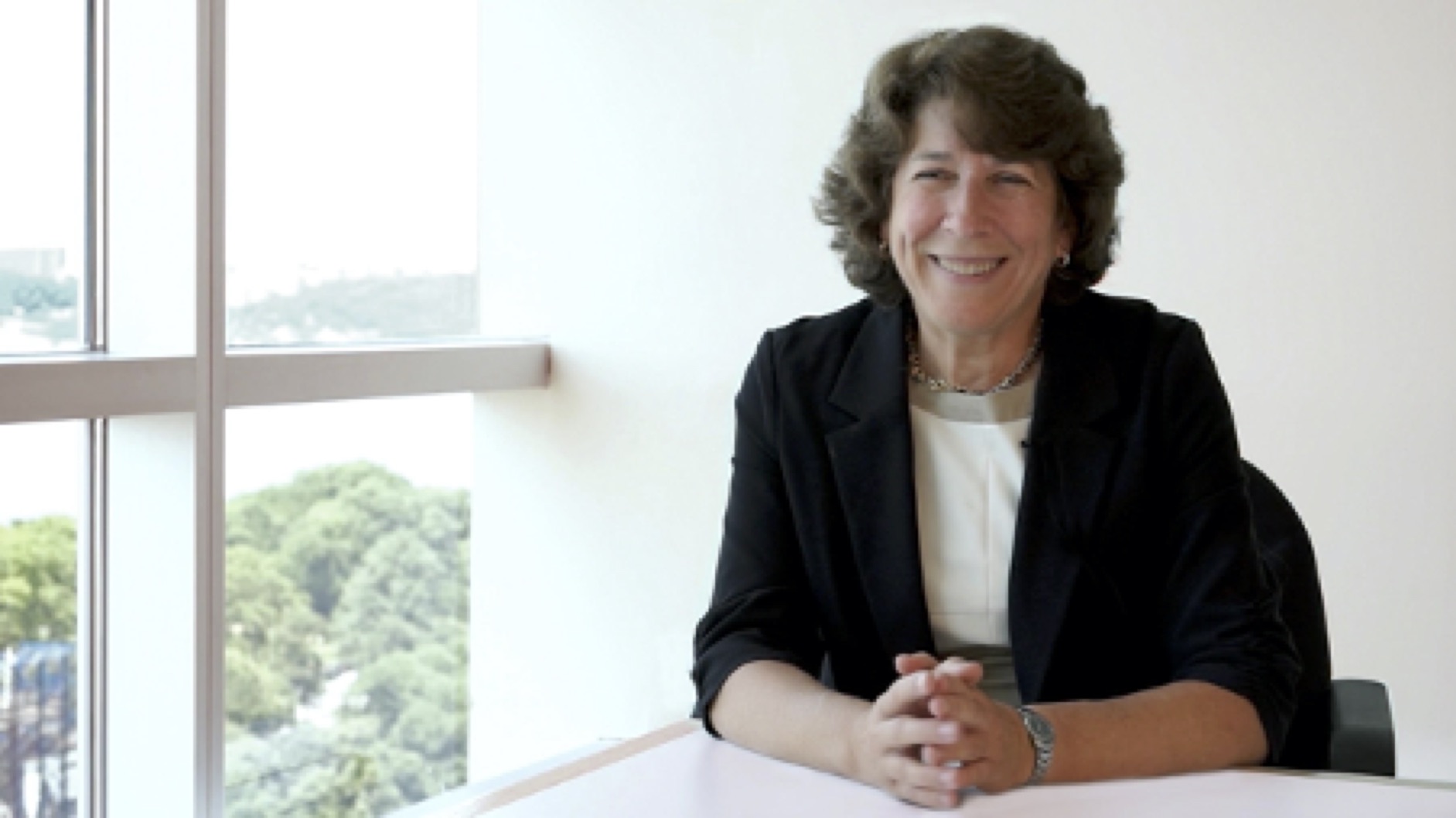
In recent years, we have seen tremendous advances in the field of natural language processing through the use of neural networks. In fact, they have done so well, that they have almost succeeded in rewriting the field as we knew it. In this talk, I examine the state of the field and its link to the past, with a focus on language generation of many forms. I ask where neural networks have been particularly successful, where approaches from the past might still be valuable, and where we need to turn in the future if we are to go beyond our current success. To answer these questions, this talk will feature clips from a series of interviews I carried out with experts in the field.
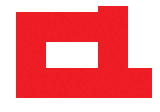
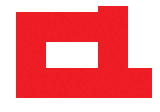
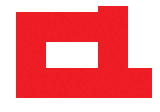
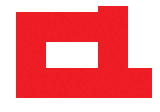
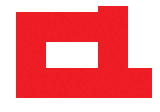
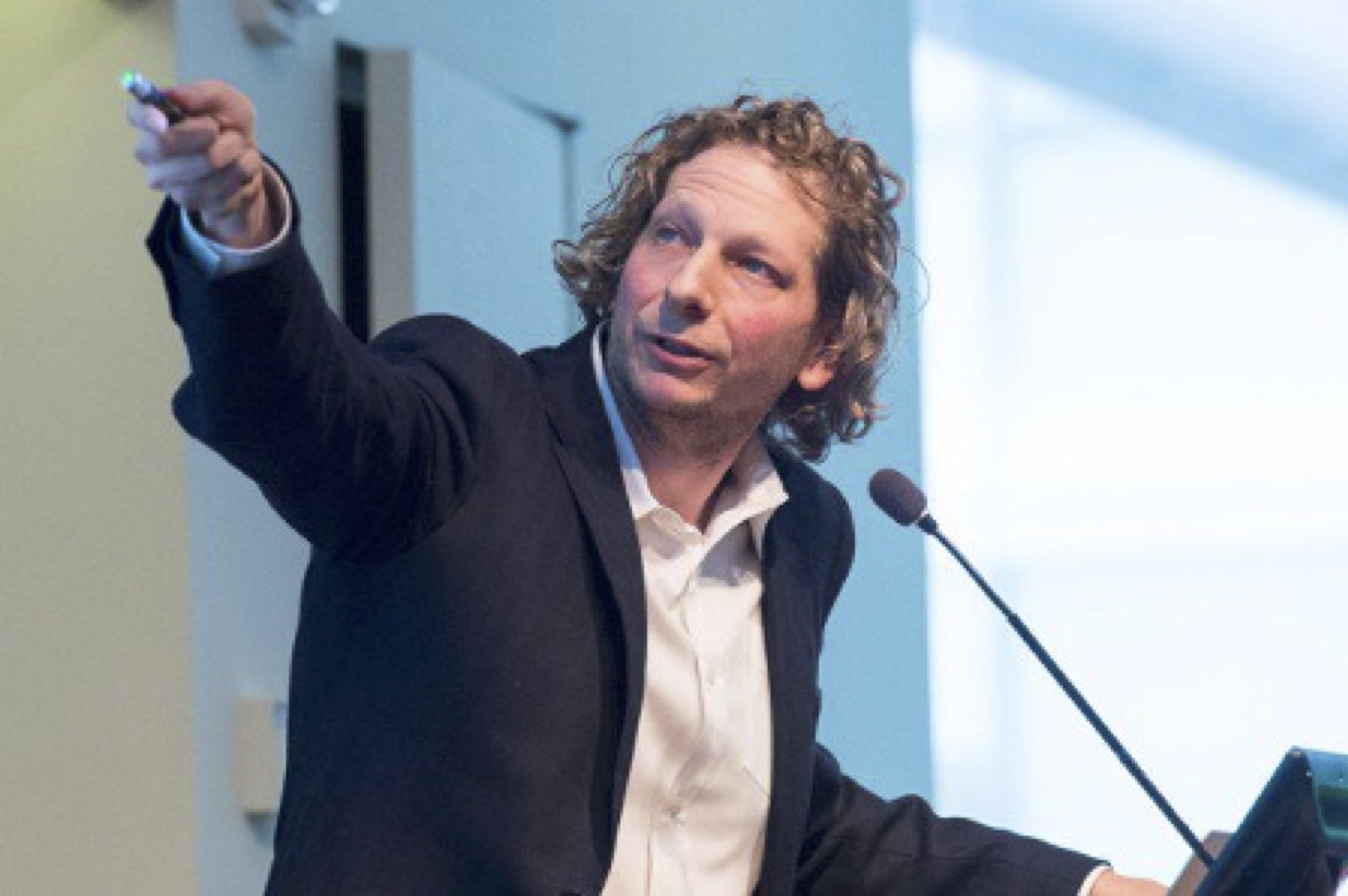
Humans learn language building on more basic conceptual and computational resources that we can already see precursors of in infancy. These include capacities for causal reasoning, symbolic rule formation, rapid abstraction, and commonsense representations of events in terms of objects, agents and their interactions. I will talk about steps towards capturing these abilities in engineering terms, using tools from hierarchical Bayesian models, probabilistic programs, program induction, and neuro-symbolic architectures. I will show examples of how these tools have been applied in both cognitive science and AI contexts, and point to ways they might be useful in building more human-like language, learning and reasoning in machines.
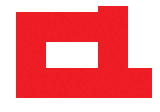
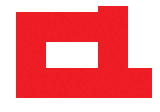
- EMNLP 2020: Bonnie Webber, Trevor Cohn, Yulan He, Yang Liu
- AACL 2020: Kam-Fai Wong, Kevin Knight, Hua Wu
- COLING 2020: Donia Scott, Nuria Bel, Chengqing Zong
- EACL 2021: Paola Merlo, Jorg Tiedemann, Reut Tsarfaty
- NAACL 2021: Kristina Toutanova, Luke Zettlemoyer, Anna Rumshisky, Saif Mohammad
- ACL 2021: Chengqing Zong, Thepchai Supnithi
- ACL 2023 Call for Bids: Tim Baldwin
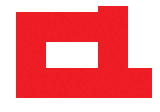