Learning to Ask More: Semi-Autoregressive Sequential Question Generation under Dual-Graph Interaction
Zi Chai, Xiaojun Wan
Generation Long Paper
Session 1A: Jul 6
(05:00-06:00 GMT)
Session 2A: Jul 6
(08:00-09:00 GMT)
Abstract:
Traditional Question Generation (TQG) aims to generate a question given an input passage and an answer. When there is a sequence of answers, we can perform Sequential Question Generation (SQG) to produce a series of interconnected questions. Since the frequently occurred information omission and coreference between questions, SQG is rather challenging. Prior works regarded SQG as a dialog generation task and recurrently produced each question. However, they suffered from problems caused by error cascades and could only capture limited context dependencies. To this end, we generate questions in a semi-autoregressive way. Our model divides questions into different groups and generates each group of them in parallel. During this process, it builds two graphs focusing on information from passages, answers respectively and performs dual-graph interaction to get information for generation. Besides, we design an answer-aware attention mechanism and the coarse-to-fine generation scenario. Experiments on our new dataset containing 81.9K questions show that our model substantially outperforms prior works.
You can open the
pre-recorded video
in a separate window.
NOTE: The SlidesLive video may display a random order of the authors.
The correct author list is shown at the top of this webpage.
Similar Papers
Syn-QG: Syntactic and Shallow Semantic Rules for Question Generation
Kaustubh Dhole, Christopher D. Manning,
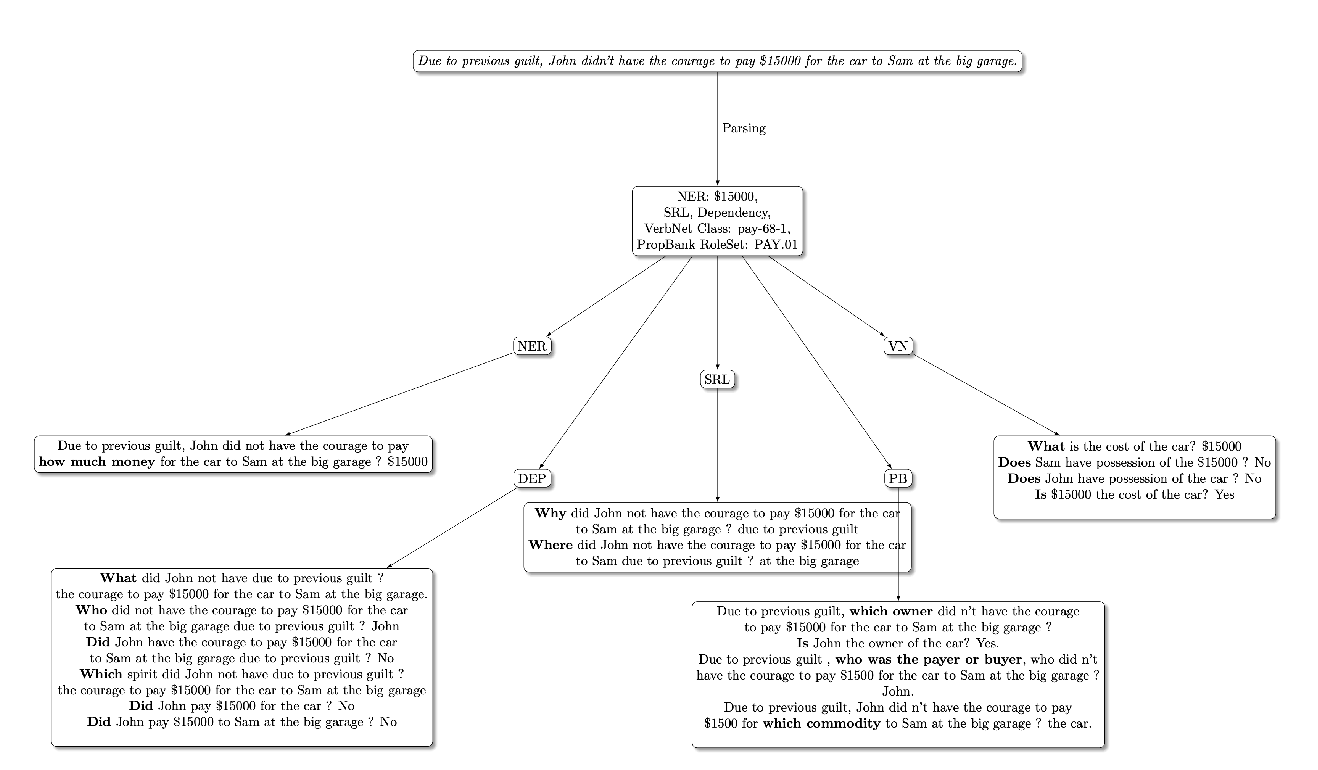
Two Birds, One Stone: A Simple, Unified Model for Text Generation from Structured and Unstructured Data
Hamidreza Shahidi, Ming Li, Jimmy Lin,
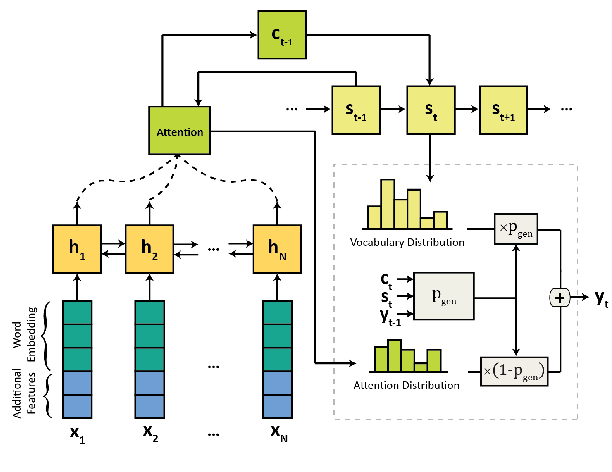
Crossing Variational Autoencoders for Answer Retrieval
Wenhao Yu, Lingfei Wu, Qingkai Zeng, Shu Tao, Yu Deng, Meng Jiang,
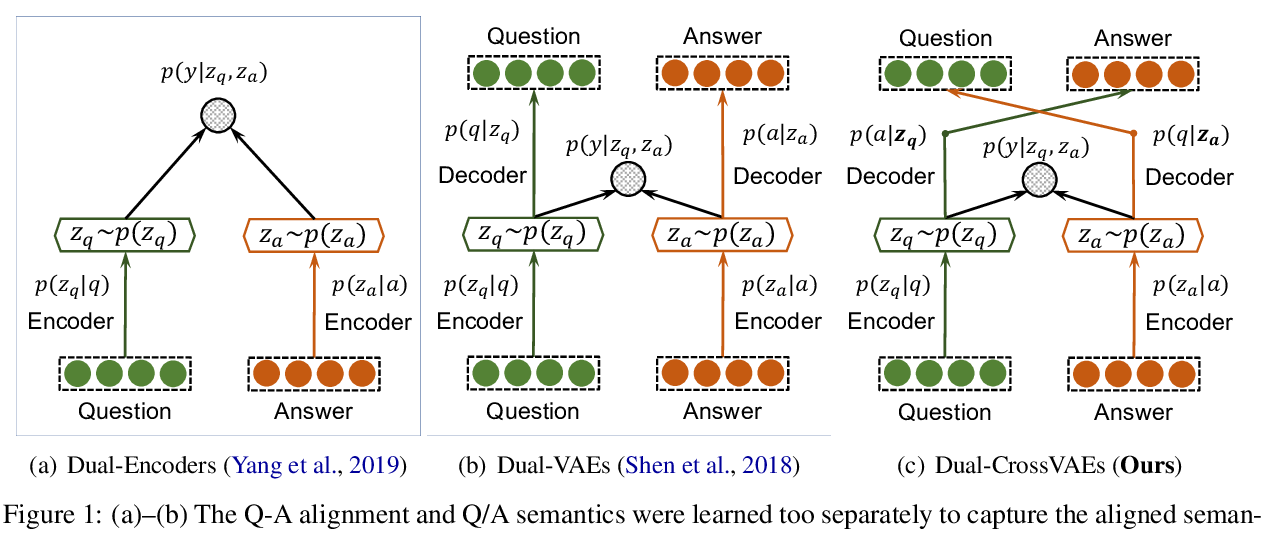
Exploring the Role of Context to Distinguish Rhetorical and Information-Seeking Questions
Yuan Zhuang, Ellen Riloff,
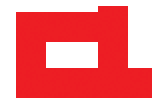