Relation-Aware Collaborative Learning for Unified Aspect-Based Sentiment Analysis
Zhuang Chen, Tieyun Qian
Sentiment Analysis, Stylistic Analysis, and Argument Mining Long Paper
Session 6B: Jul 7
(06:00-07:00 GMT)
Session 7B: Jul 7
(09:00-10:00 GMT)
Abstract:
Aspect-based sentiment analysis (ABSA) involves three subtasks, i.e., aspect term extraction, opinion term extraction, and aspect-level sentiment classification. Most existing studies focused on one of these subtasks only. Several recent researches made successful attempts to solve the complete ABSA problem with a unified framework. However, the interactive relations among three subtasks are still under-exploited. We argue that such relations encode collaborative signals between different subtasks. For example, when the opinion term is ``delicious'', the aspect term must be ``food'' rather than ``place''. In order to fully exploit these relations, we propose a Relation-Aware Collaborative Learning (RACL) framework which allows the subtasks to work coordinately via the multi-task learning and relation propagation mechanisms in a stacked multi-layer network. Extensive experiments on three real-world datasets demonstrate that RACL significantly outperforms the state-of-the-art methods for the complete ABSA task.
You can open the
pre-recorded video
in a separate window.
NOTE: The SlidesLive video may display a random order of the authors.
The correct author list is shown at the top of this webpage.
Similar Papers
SpanMlt: A Span-based Multi-Task Learning Framework for Pair-wise Aspect and Opinion Terms Extraction
He Zhao, Longtao Huang, Rong Zhang, Quan Lu, Hui Xue,
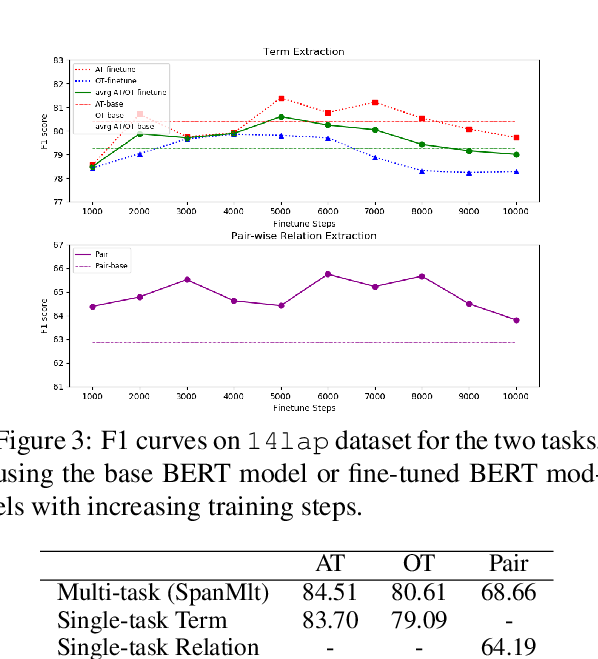
Modelling Context and Syntactical Features for Aspect-based Sentiment Analysis
Minh Hieu Phan, Philip O. Ogunbona,
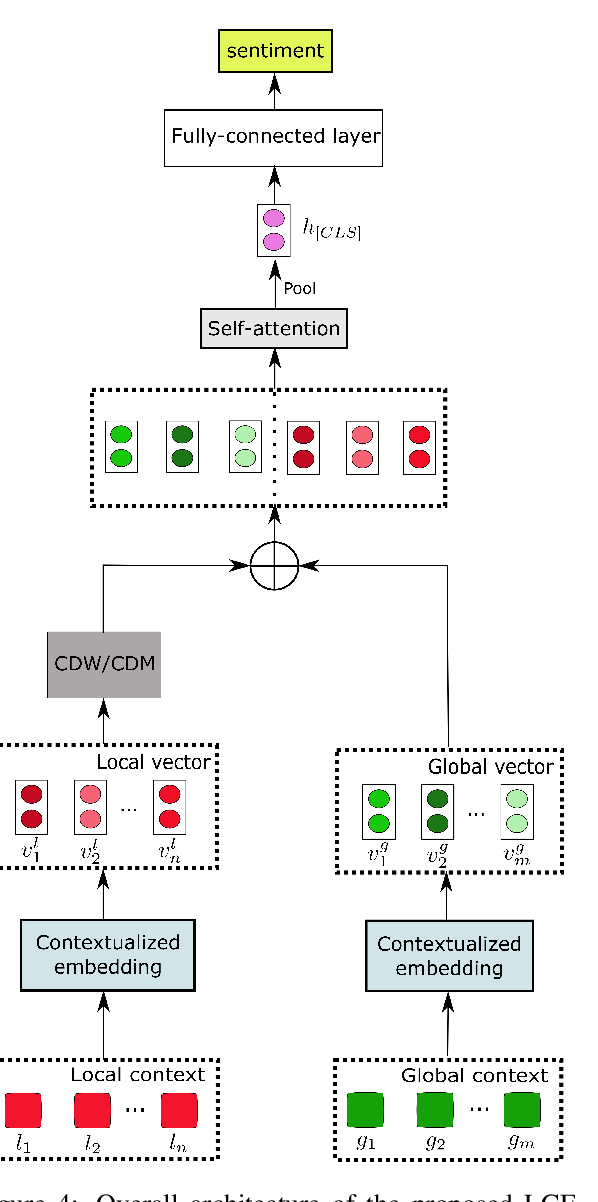
Conditional Augmentation for Aspect Term Extraction via Masked Sequence-to-Sequence Generation
Kun Li, Chengbo Chen, Xiaojun Quan, Qing Ling, Yan Song,
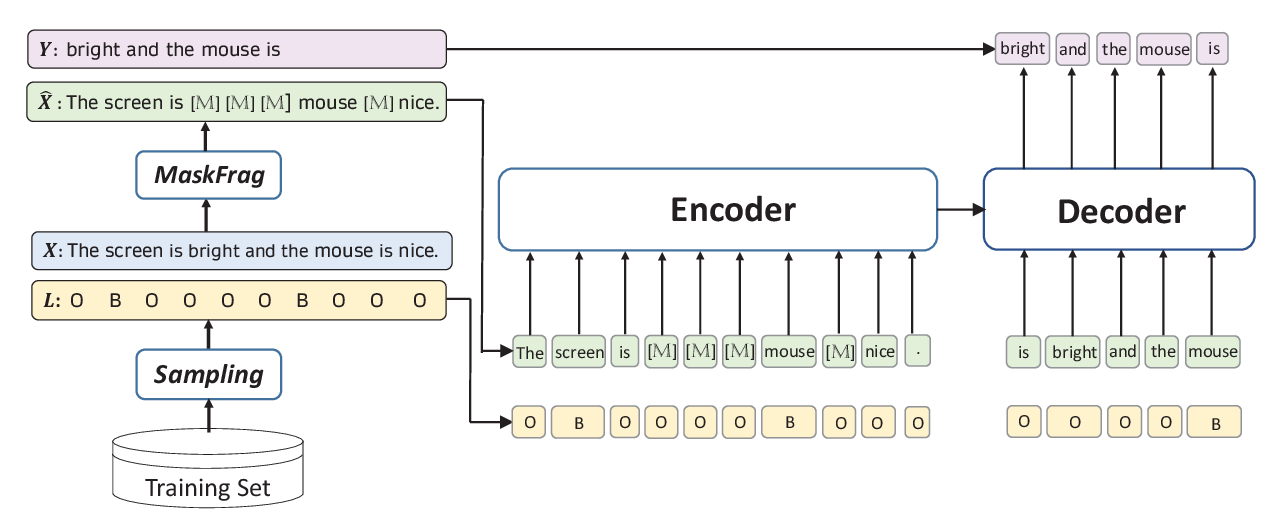
Relational Graph Attention Network for Aspect-based Sentiment Analysis
Kai Wang, Weizhou Shen, Yunyi Yang, Xiaojun Quan, Rui Wang,
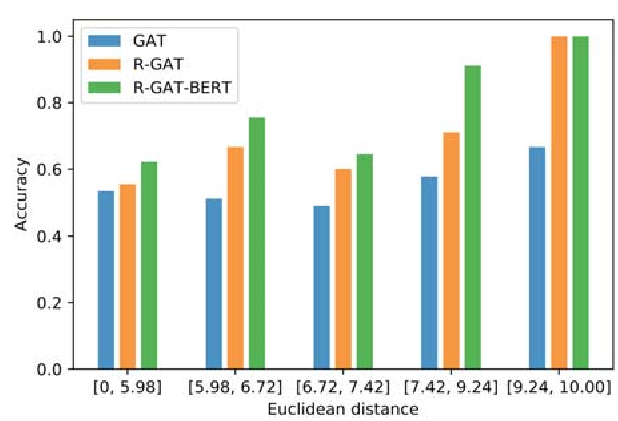