Predicting the Topical Stance and Political Leaning of Media using Tweets
Peter Stefanov, Kareem Darwish, Atanas Atanasov, Preslav Nakov
Computational Social Science and Social Media Long Paper
Session 1B: Jul 6
(06:00-07:00 GMT)
Session 2A: Jul 6
(08:00-09:00 GMT)
Abstract:
Discovering the stances of media outlets and influential people on current, debatable topics is important for social statisticians and policy makers. Many supervised solutions exist for determining viewpoints, but manually annotating training data is costly. In this paper, we propose a cascaded method that uses unsupervised learning to ascertain the stance of Twitter users with respect to a polarizing topic by leveraging their retweet behavior; then, it uses supervised learning based on user labels to characterize both the general political leaning of online media and of popular Twitter users, as well as their stance with respect to the target polarizing topic. We evaluate the model by comparing its predictions to gold labels from the Media Bias/Fact Check website, achieving 82.6% accuracy.
You can open the
pre-recorded video
in a separate window.
NOTE: The SlidesLive video may display a random order of the authors.
The correct author list is shown at the top of this webpage.
Similar Papers
Neural Temporal Opinion Modelling for Opinion Prediction on Twitter
Lixing Zhu, Yulan He, Deyu Zhou,
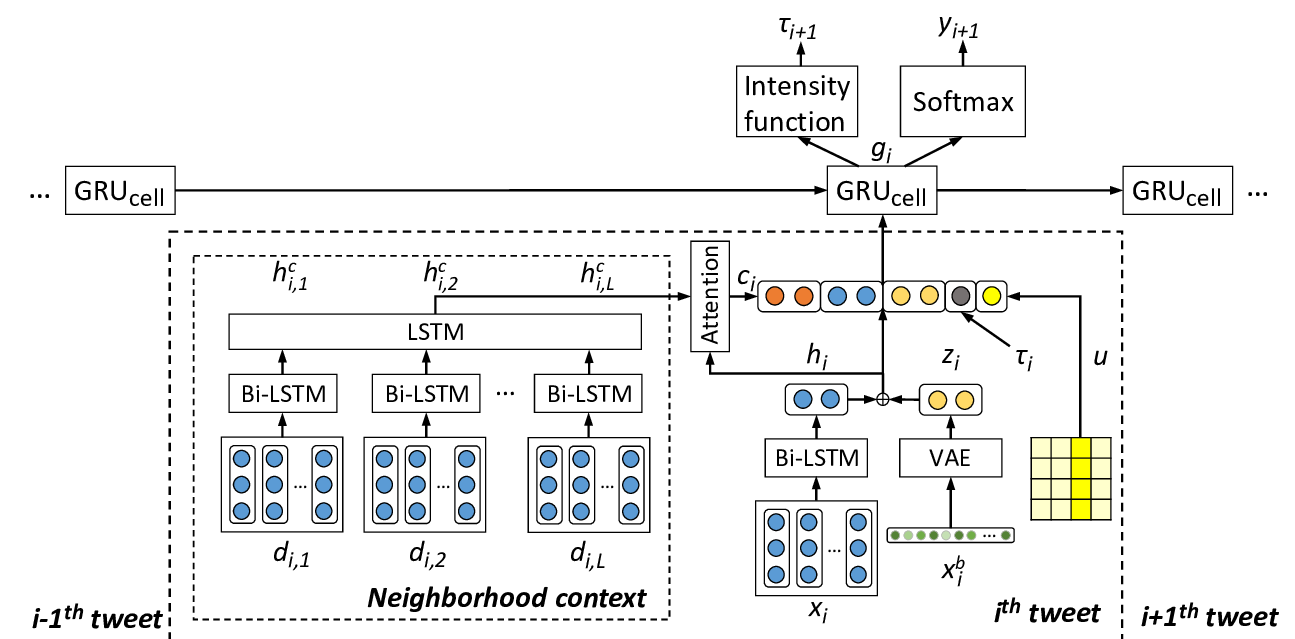
Agreement Prediction of Arguments in Cyber Argumentation for Detecting Stance Polarity and Intensity
Joseph Sirrianni, Xiaoqing Liu, Douglas Adams,
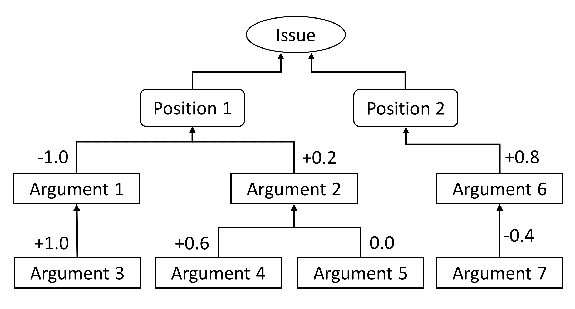
Dynamic Online Conversation Recommendation
Xingshan Zeng, Jing Li, Lu Wang, Zhiming Mao, Kam-Fai Wong,
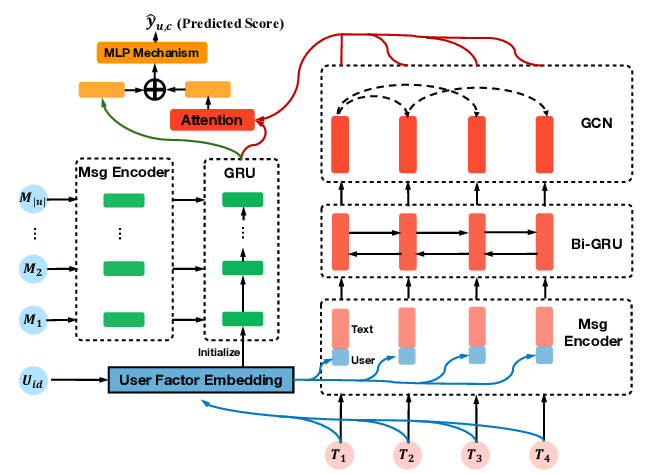