End-to-End Neural Pipeline for Goal-Oriented Dialogue Systems using GPT-2
Donghoon Ham, Jeong-Gwan Lee, Youngsoo Jang, Kee-Eung Kim
Dialogue and Interactive Systems Long Paper
Session 1B: Jul 6
(06:00-07:00 GMT)
Session 3B: Jul 6
(13:00-14:00 GMT)
Abstract:
The goal-oriented dialogue system needs to be optimized for tracking the dialogue flow and carrying out an effective conversation under various situations to meet the user goal. The traditional approach to build such a dialogue system is to take a pipelined modular architecture, where its modules are optimized individually. However, such an optimization scheme does not necessarily yield the overall performance improvement of the whole system. On the other hand, end-to-end dialogue systems with monolithic neural architecture are often trained only with input-output utterances, without taking into account the entire annotations available in the corpus. This scheme makes it difficult for goal-oriented dialogues where the system needs to integrate with external systems or to provide interpretable information about why the system generated a particular response. In this paper, we present an end-to-end neural architecture for dialogue systems that addresses both challenges above. In the human evaluation, our dialogue system achieved the success rate of 68.32%, the language understanding score of 4.149, and the response appropriateness score of 4.287, which ranked the system at the top position in the end-to-end multi-domain dialogue system task in the 8th dialogue systems technology challenge (DSTC8).
You can open the
pre-recorded video
in a separate window.
NOTE: The SlidesLive video may display a random order of the authors.
The correct author list is shown at the top of this webpage.
Similar Papers
ConvLab-2: An Open-Source Toolkit for Building, Evaluating, and Diagnosing Dialogue Systems
Qi Zhu, Zheng Zhang, Yan Fang, Xiang Li, Ryuichi Takanobu, Jinchao Li, Baolin Peng, Jianfeng Gao, Xiaoyan Zhu, Minlie Huang,
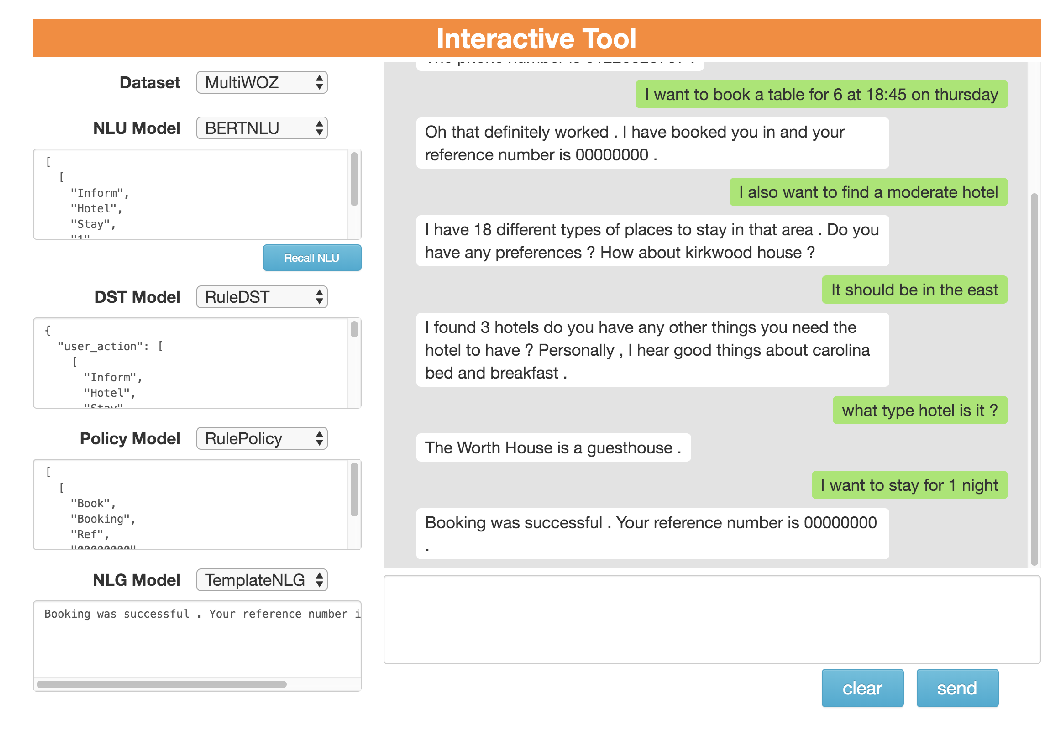
Multi-Domain Dialogue Acts and Response Co-Generation
Kai Wang, Junfeng Tian, Rui Wang, Xiaojun Quan, Jianxing Yu,
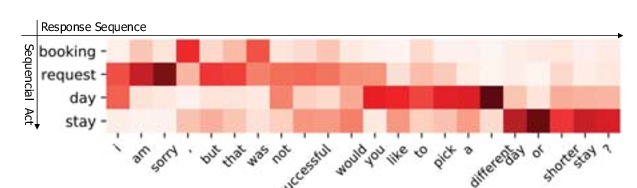
Semi-Supervised Dialogue Policy Learning via Stochastic Reward Estimation
Xinting Huang, Jianzhong Qi, Yu Sun, Rui Zhang,
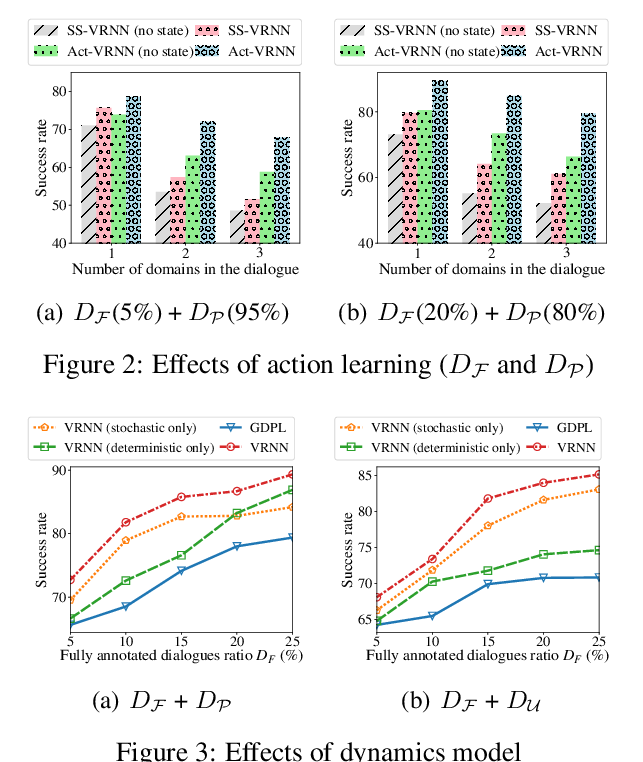