Modeling Long Context for Task-Oriented Dialogue State Generation
Jun Quan, Deyi Xiong
Dialogue and Interactive Systems Short Paper
Session 12B: Jul 8
(09:00-10:00 GMT)
Session 13B: Jul 8
(13:00-14:00 GMT)
Abstract:
Based on the recently proposed transferable dialogue state generator (TRADE) that predicts dialogue states from utterance-concatenated dialogue context, we propose a multi-task learning model with a simple yet effective utterance tagging technique and a bidirectional language model as an auxiliary task for task-oriented dialogue state generation. By enabling the model to learn a better representation of the long dialogue context, our approaches attempt to solve the problem that the performance of the baseline significantly drops when the input dialogue context sequence is long. In our experiments, our proposed model achieves a 7.03% relative improvement over the baseline, establishing a new state-of-the-art joint goal accuracy of 52.04% on the MultiWOZ 2.0 dataset.
You can open the
pre-recorded video
in a separate window.
NOTE: The SlidesLive video may display a random order of the authors.
The correct author list is shown at the top of this webpage.
Similar Papers
SAS: Dialogue State Tracking via Slot Attention and Slot Information Sharing
Jiaying Hu, Yan Yang, Chencai Chen, liang he, Zhou Yu,
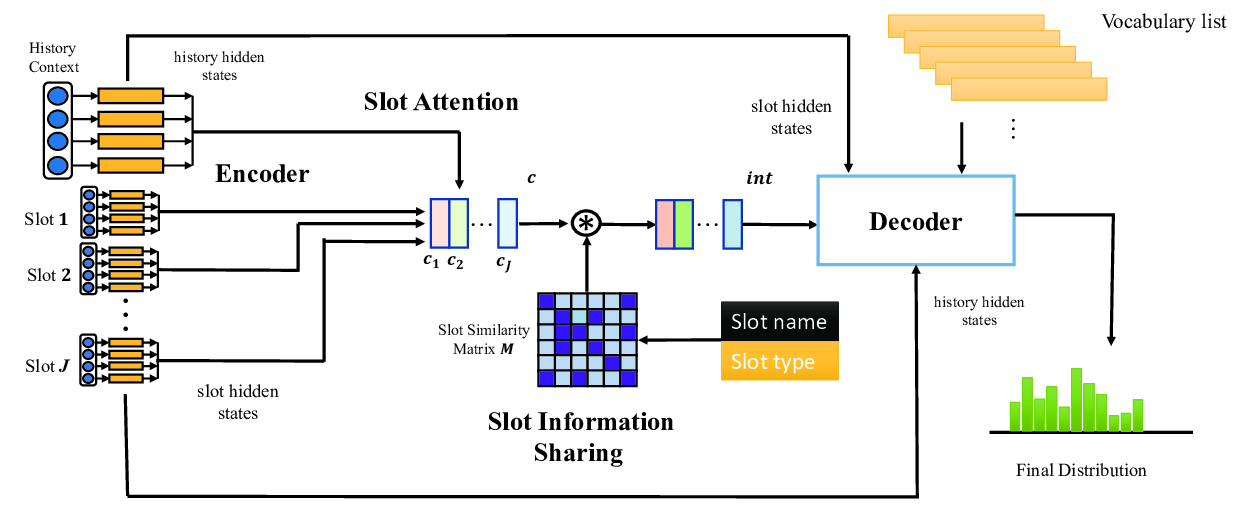
Semi-Supervised Dialogue Policy Learning via Stochastic Reward Estimation
Xinting Huang, Jianzhong Qi, Yu Sun, Rui Zhang,
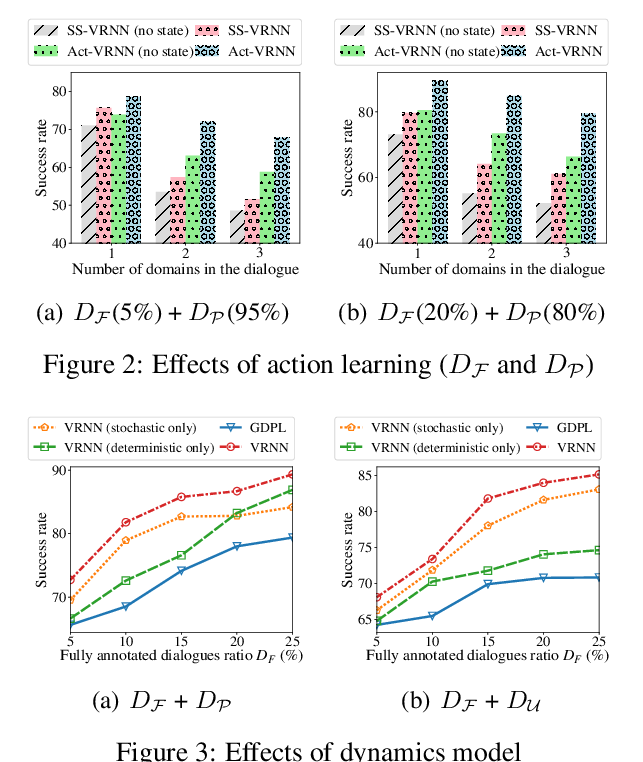
Efficient Dialogue State Tracking by Selectively Overwriting Memory
Sungdong Kim, Sohee Yang, Gyuwan Kim, Sang-Woo Lee,
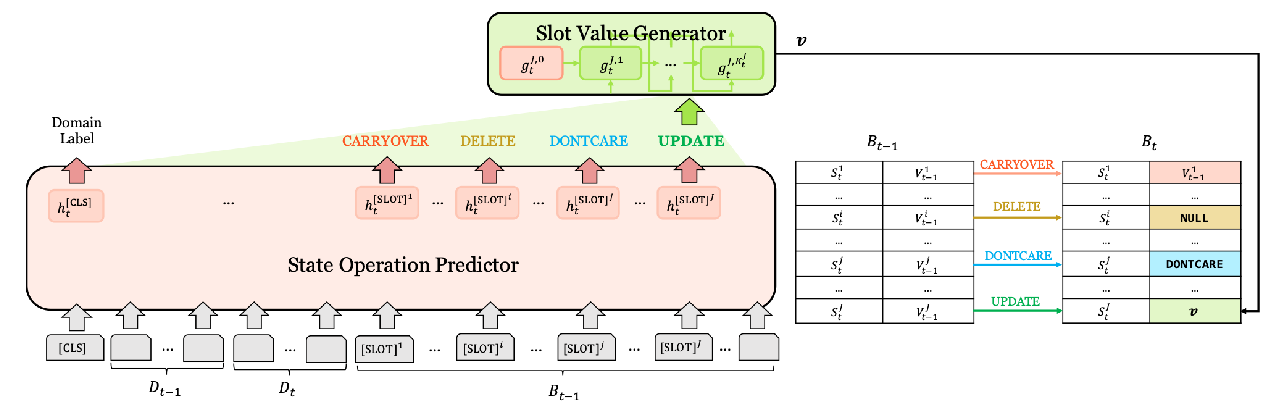
Multi-Domain Dialogue Acts and Response Co-Generation
Kai Wang, Junfeng Tian, Rui Wang, Xiaojun Quan, Jianxing Yu,
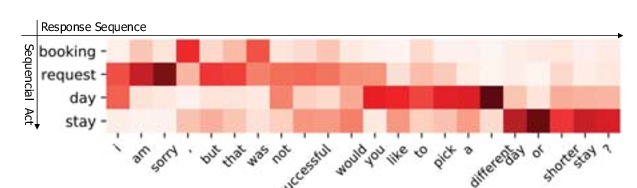