A Knowledge-Enhanced Pretraining Model for Commonsense Story Generation
Jian Guan, Fei Huang, Minlie Huang, Zhihao Zhao, Xiaoyan Zhu
Generation TACL Paper
Session 14A: Jul 8
(17:00-18:00 GMT)
Session 15A: Jul 8
(20:00-21:00 GMT)
Abstract:
Story generation, namely generating a reasonable story from a leading context, is an important but challenging task. In spite of the success in modeling fluency and local coherence, existing neural language generation models (e.g., GPT-2) still suffer from repetition, logic conflicts, and lack of long-range coherence in generated stories. We conjecture that this is because of the difficulty of associating relevant commonsense knowledge, understanding the causal relationships, and planning entities and events with proper temporal order. In this paper, we devise a knowledge-enhanced pretraining model for commonsense story generation. We propose to utilize commonsense knowledge from external knowledge bases to generate reasonable stories. To further capture the causal and temporal dependencies between the sentences in a reasonable story, we employ multi-task learning which combines a discriminative objective to distinguish true and fake stories during fine-tuning. Automatic and manual evaluation shows that our model can generate more reasonable stories than state-of-the-art baselines, particularly in terms of logic and global coherence.
You can open the
pre-recorded video
in a separate window.
NOTE: The SlidesLive video may display a random order of the authors.
The correct author list is shown at the top of this webpage.
Similar Papers
Recollection versus Imagination: Exploring Human Memory and Cognition via Neural Language Models
Maarten Sap, Eric Horvitz, Yejin Choi, Noah A. Smith, James Pennebaker,
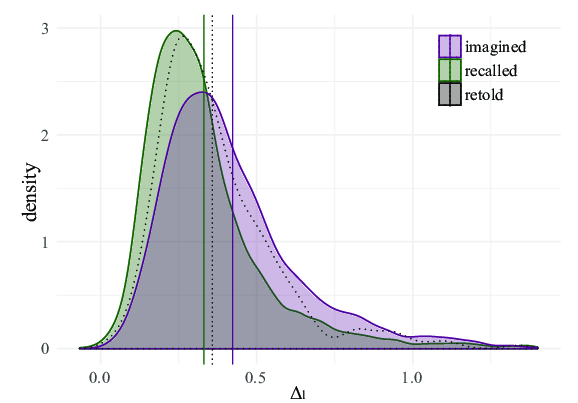
Dialogue Coherence Assessment Without Explicit Dialogue Act Labels
Mohsen Mesgar, Sebastian Bücker, Iryna Gurevych,
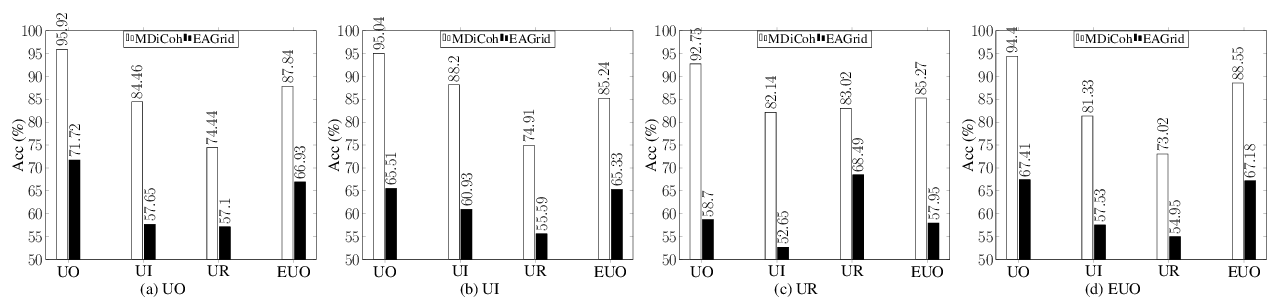
Diverse and Informative Dialogue Generation with Context-Specific Commonsense Knowledge Awareness
Sixing Wu, Ying Li, Dawei Zhang, Yang Zhou, Zhonghai Wu,
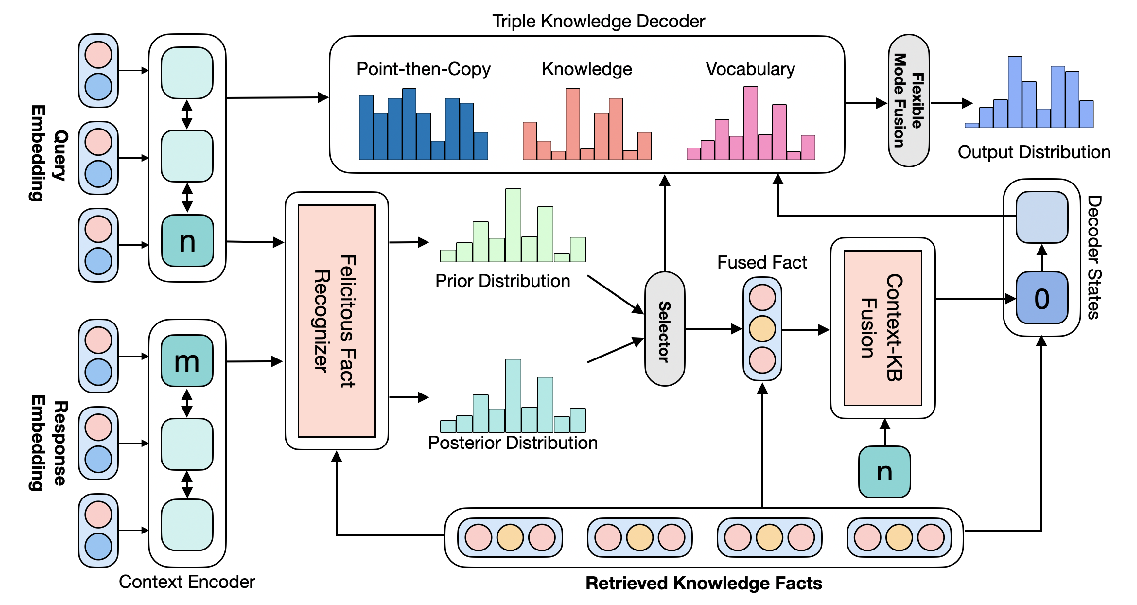
R^3: Reverse, Retrieve, and Rank for Sarcasm Generation with Commonsense Knowledge
Tuhin Chakrabarty, Debanjan Ghosh, Smaranda Muresan, Nanyun Peng,
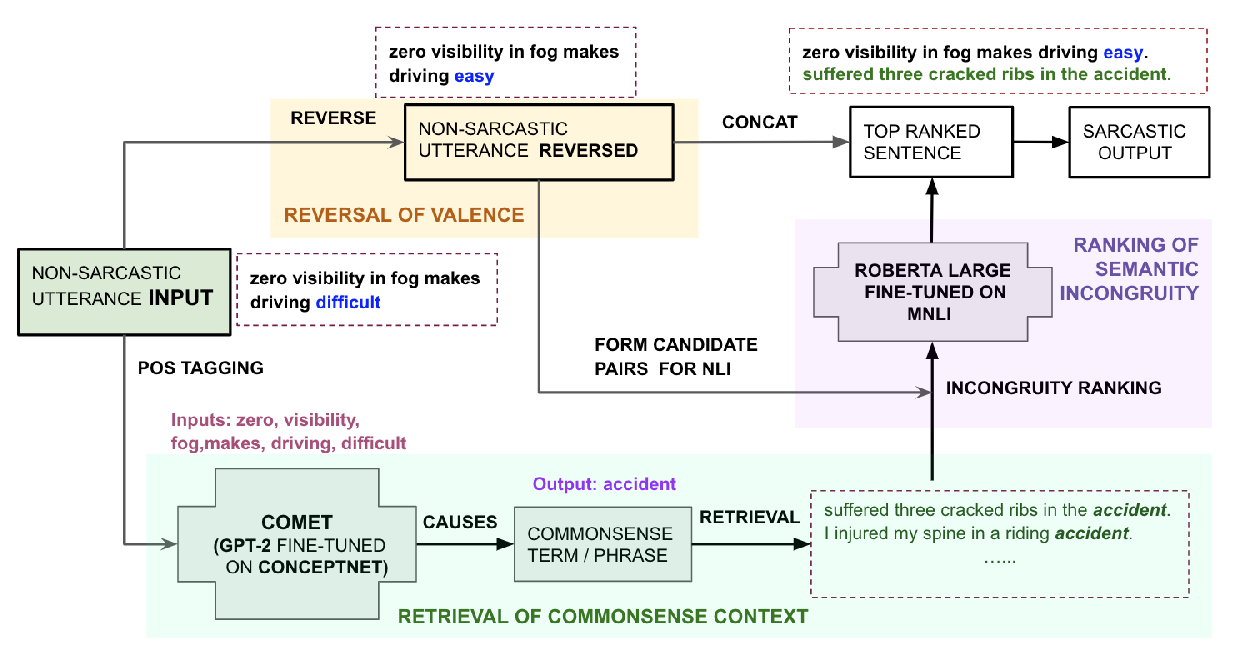