DIALOGPT : Large-Scale Generative Pre-training for Conversational Response Generation
Yizhe Zhang, Siqi Sun, Michel Galley, Yen-Chun Chen, Chris Brockett, Xiang Gao, Jianfeng Gao, Jingjing Liu, Bill Dolan
System Demonstrations Demo Paper
Demo Session 5A-2: Jul 7
(20:00-21:00 GMT)
Demo Session 5A-3: Jul 8
(20:00-21:00 GMT)
Abstract:
We present a large, tunable neural conversational response generation model, DIALOGPT (dialogue generative pre-trained transformer). Trained on 147M conversation-like exchanges extracted from Reddit comment chains over a period spanning from 2005 through 2017, DialoGPT extends the Hugging Face PyTorch transformer to attain a performance close to human both in terms of automatic and human evaluation in single-turn dialogue settings. We show that conversational systems that leverage DialoGPT generate more relevant, contentful and context-consistent responses than strong baseline systems. The pre-trained model and training pipeline are publicly released to facilitate research into neural response generation and the development of more intelligent open-domain dialogue systems.
You can open the
pre-recorded video
in a separate window.
NOTE: The SlidesLive video may display a random order of the authors.
The correct author list is shown at the top of this webpage.
Similar Papers
Multi-Domain Dialogue Acts and Response Co-Generation
Kai Wang, Junfeng Tian, Rui Wang, Xiaojun Quan, Jianxing Yu,
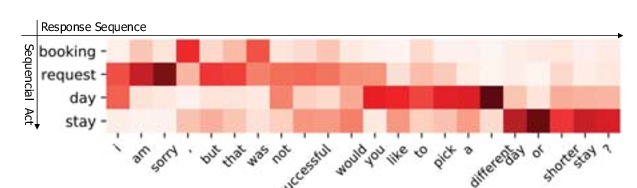
Conversational Graph Grounded Policy Learning for Open-Domain Conversation Generation
Jun Xu, Haifeng Wang, Zheng-Yu Niu, Hua Wu, Wanxiang Che, Ting Liu,
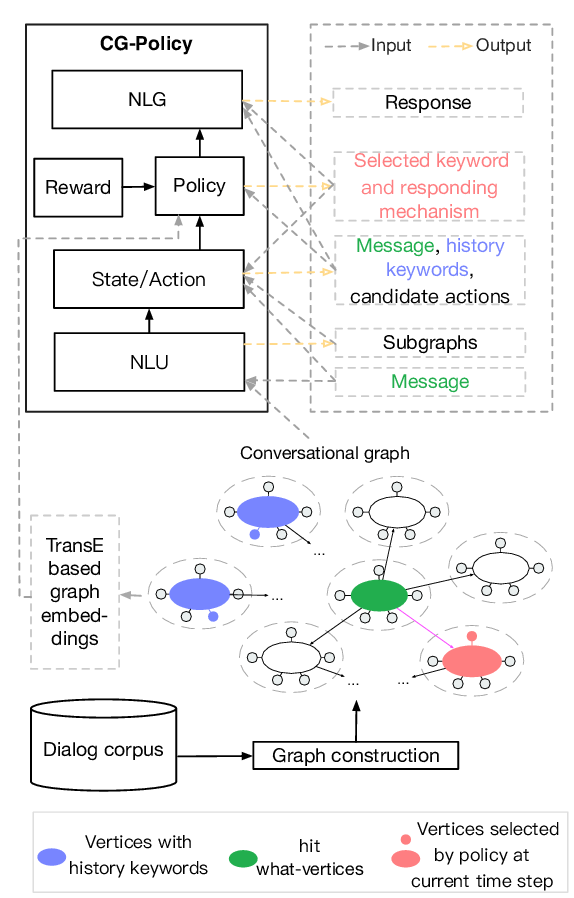