Paraphrase Augmented Task-Oriented Dialog Generation
Silin Gao, Yichi Zhang, Zhijian Ou, Zhou Yu
Dialogue and Interactive Systems Long Paper
Session 1B: Jul 6
(06:00-07:00 GMT)
Session 3A: Jul 6
(12:00-13:00 GMT)
Abstract:
Neural generative models have achieved promising performance on dialog generation tasks if given a huge data set. However, the lack of high-quality dialog data and the expensive data annotation process greatly limit their application in real world settings. We propose a paraphrase augmented response generation (PARG) framework that jointly trains a paraphrase model and a response generation model to improve the dialog generation performance. We also design a method to automatically construct paraphrase training data set based on dialog state and dialog act labels. PARG is applicable to various dialog generation models, such as TSCP (Lei et al., 2018) and DAMD (Zhang et al., 2019). Experimental results show that the proposed framework improves these state-of-the-art dialog models further on CamRest676 and MultiWOZ. PARG also outperforms other data augmentation methods significantly in dialog generation tasks, especially under low resource settings.
You can open the
pre-recorded video
in a separate window.
NOTE: The SlidesLive video may display a random order of the authors.
The correct author list is shown at the top of this webpage.
Similar Papers
Conversation Learner - A Machine Teaching Tool for Building Dialog Managers for Task-Oriented Dialog Systems
Swadheen Shukla, Lars Liden, Shahin Shayandeh, Eslam Kamal, Jinchao Li, Matt Mazzola, Thomas Park, Baolin Peng, Jianfeng Gao,
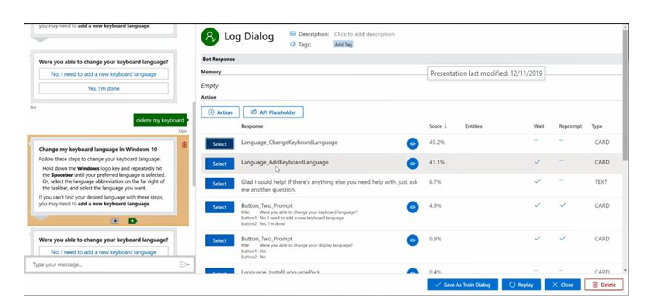
Towards Conversational Recommendation over Multi-Type Dialogs
Zeming Liu, Haifeng Wang, Zheng-Yu Niu, Hua Wu, Wanxiang Che, Ting Liu,
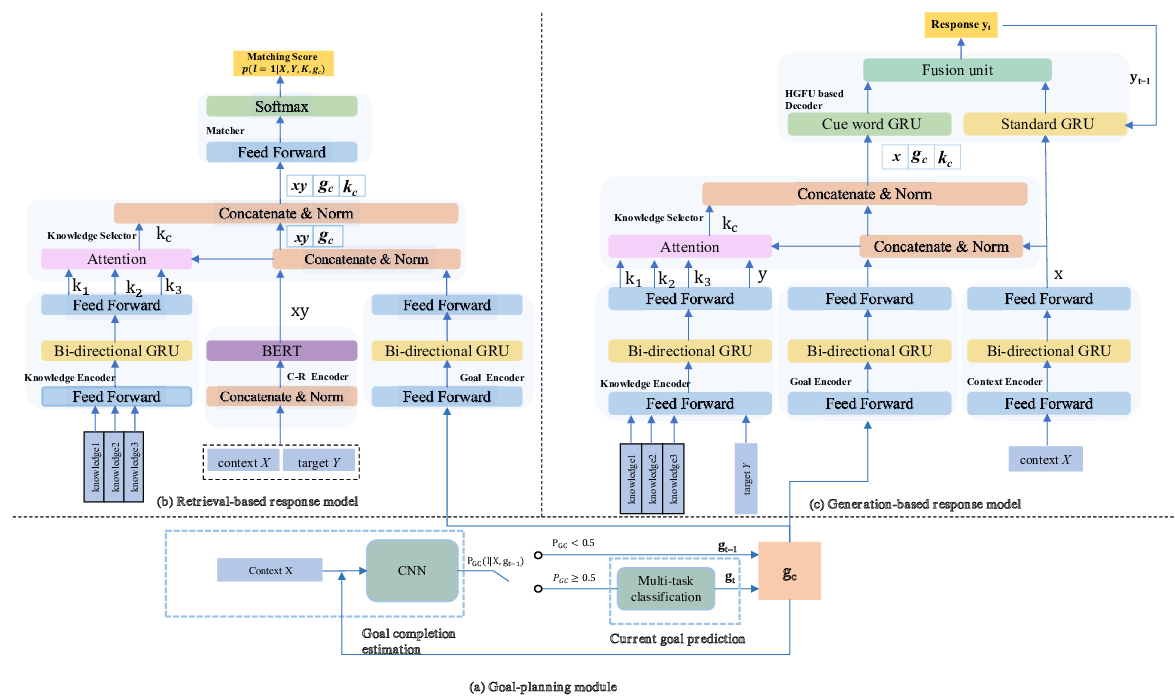
Beyond User Self-Reported Likert Scale Ratings: A Comparison Model for Automatic Dialog Evaluation
Weixin Liang, James Zou, Zhou Yu,
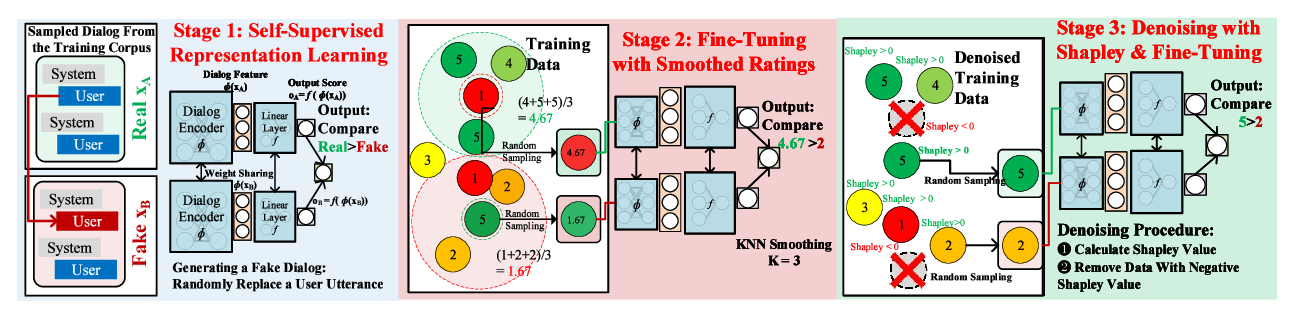
Conversational Graph Grounded Policy Learning for Open-Domain Conversation Generation
Jun Xu, Haifeng Wang, Zheng-Yu Niu, Hua Wu, Wanxiang Che, Ting Liu,
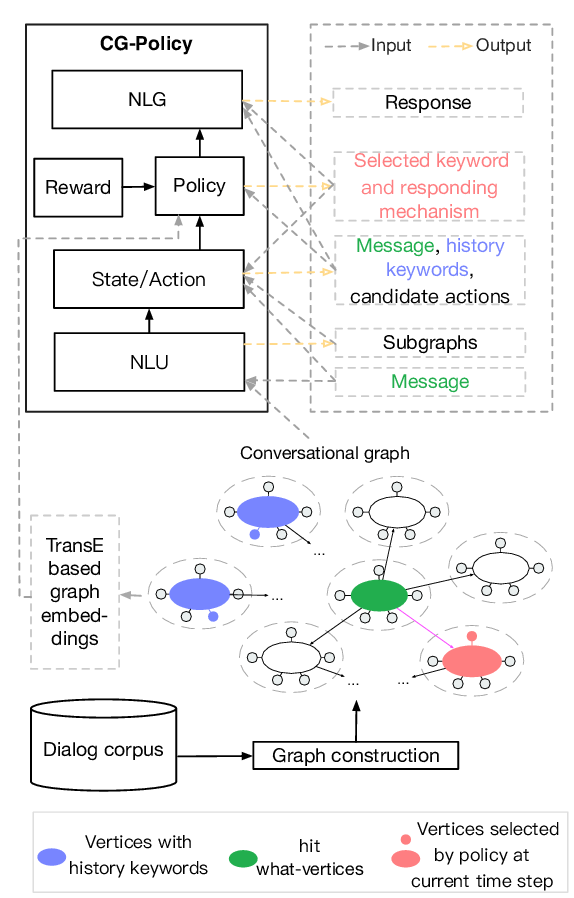