NSTM: Real-Time Query-Driven News Overview Composition at Bloomberg
Joshua Bambrick, Minjie Xu, Andy Almonte, Igor Malioutov, Guim Perarnau, Vittorio Selo, Iat Chong Chan
System Demonstrations Demo Paper
Demo Session 3B-3: Jul 8
(12:45-13:45 GMT)
Demo Session 5B-3: Jul 8
(20:45-21:45 GMT)
Abstract:
Millions of news articles from hundreds of thousands of sources around the globe appear in news aggregators every day. Consuming such a volume of news presents an almost insurmountable challenge. For example, a reader searching on Bloomberg's system for news about the U.K. would find 10,000 articles on a typical day. Apple Inc., the world's most journalistically covered company, garners around 1,800 news articles a day. We realized that a new kind of summarization engine was needed, one that would condense large volumes of news into short, easy to absorb points. The system would filter out noise and duplicates to identify and summarize key news about companies, countries or markets. When given a user query, Bloomberg's solution, Key News Themes (or NSTM), leverages state-of-the-art semantic clustering techniques and novel summarization methods to produce comprehensive, yet concise, digests to dramatically simplify the news consumption process. NSTM is available to hundreds of thousands of readers around the world and serves thousands of requests daily with sub-second latency. At ACL 2020, we will present a demo of NSTM.
You can open the
pre-recorded video
in a separate window.
NOTE: The SlidesLive video may display a random order of the authors.
The correct author list is shown at the top of this webpage.
Similar Papers
MIND: A Large-scale Dataset for News Recommendation
Fangzhao Wu, Ying Qiao, Jiun-Hung Chen, Chuhan Wu, Tao Qi, Jianxun Lian, Danyang Liu, Xing Xie, Jianfeng Gao, Winnie Wu, Ming Zhou,
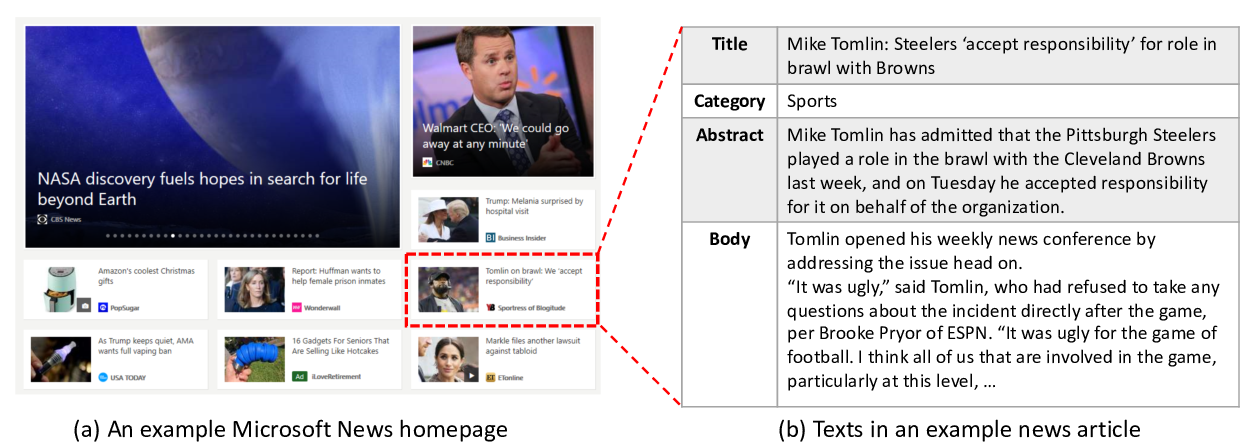
Discourse as a Function of Event: Profiling Discourse Structure in News Articles around the Main Event
Prafulla Kumar Choubey, Aaron Lee, Ruihong Huang, Lu Wang,
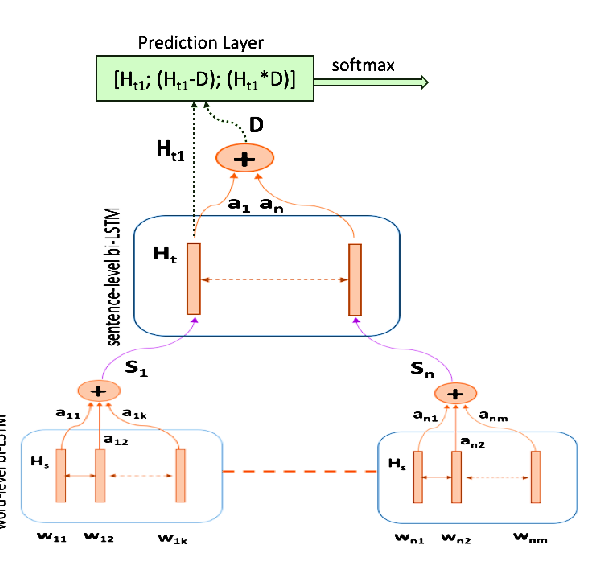
Fine-grained Interest Matching for Neural News Recommendation
Heyuan Wang, Fangzhao Wu, Zheng Liu, Xing Xie,
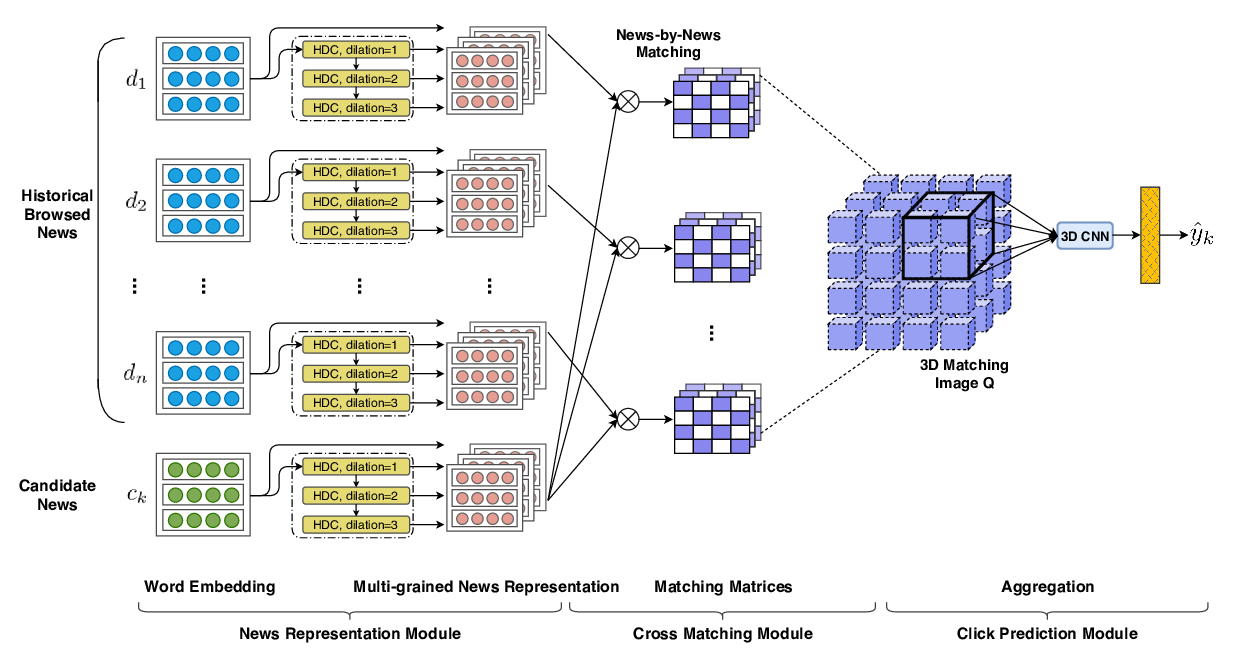