MIND: A Large-scale Dataset for News Recommendation
Fangzhao Wu, Ying Qiao, Jiun-Hung Chen, Chuhan Wu, Tao Qi, Jianxun Lian, Danyang Liu, Xing Xie, Jianfeng Gao, Winnie Wu, Ming Zhou
Resources and Evaluation Long Paper
Session 6B: Jul 7
(06:00-07:00 GMT)
Session 8A: Jul 7
(12:00-13:00 GMT)
Abstract:
News recommendation is an important technique for personalized news service. Compared with product and movie recommendations which have been comprehensively studied, the research on news recommendation is much more limited, mainly due to the lack of a high-quality benchmark dataset. In this paper, we present a large-scale dataset named MIND for news recommendation. Constructed from the user click logs of Microsoft News, MIND contains 1 million users and more than 160k English news articles, each of which has rich textual content such as title, abstract and body. We demonstrate MIND a good testbed for news recommendation through a comparative study of several state-of-the-art news recommendation methods which are originally developed on different proprietary datasets. Our results show the performance of news recommendation highly relies on the quality of news content understanding and user interest modeling. Many natural language processing techniques such as effective text representation methods and pre-trained language models can effectively improve the performance of news recommendation. The MIND dataset will be available at https://msnews.github.io.
You can open the
pre-recorded video
in a separate window.
NOTE: The SlidesLive video may display a random order of the authors.
The correct author list is shown at the top of this webpage.
Similar Papers
Fine-grained Interest Matching for Neural News Recommendation
Heyuan Wang, Fangzhao Wu, Zheng Liu, Xing Xie,
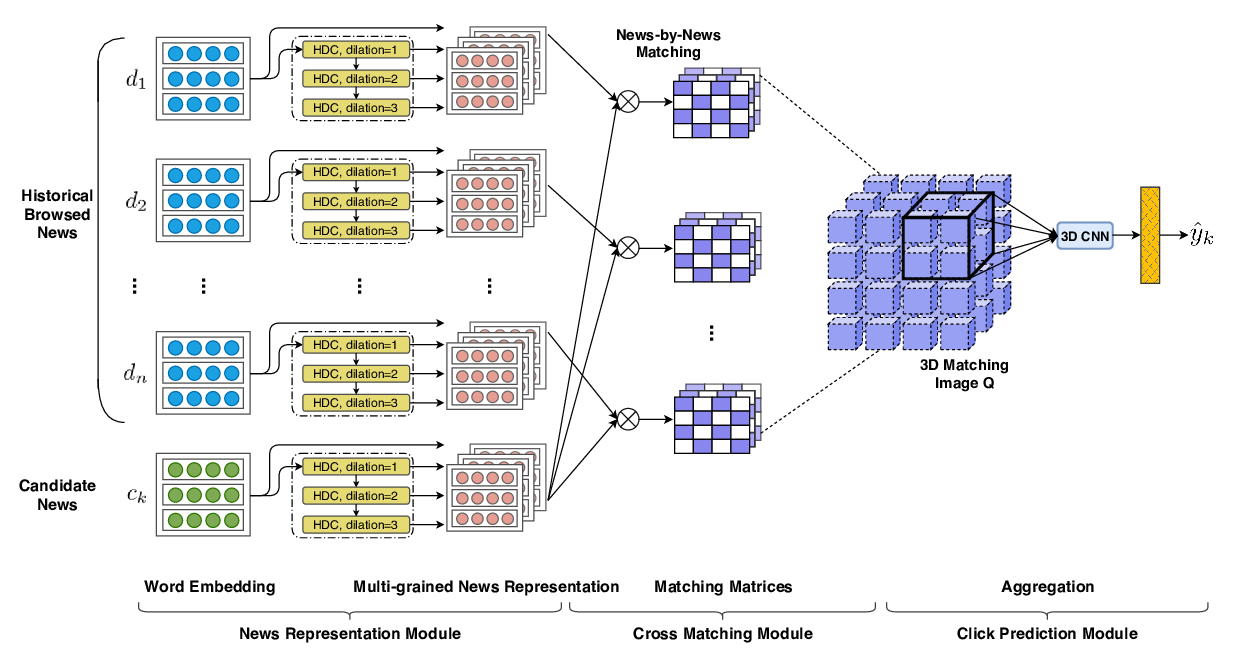
Graph Neural News Recommendation with Unsupervised Preference Disentanglement
Linmei Hu, Siyong Xu, Chen Li, Cheng Yang, Chuan Shi, Nan Duan, Xing Xie, Ming Zhou,
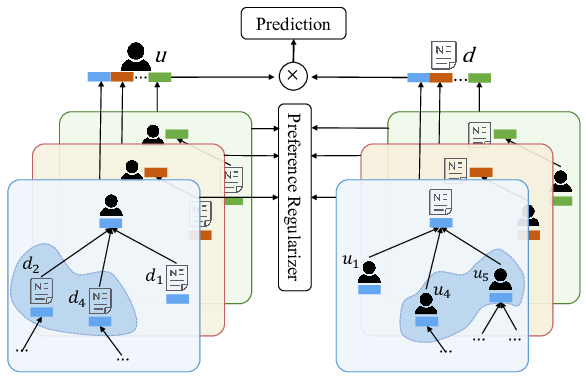
NSTM: Real-Time Query-Driven News Overview Composition at Bloomberg
Joshua Bambrick, Minjie Xu, Andy Almonte, Igor Malioutov, Guim Perarnau, Vittorio Selo, Iat Chong Chan,
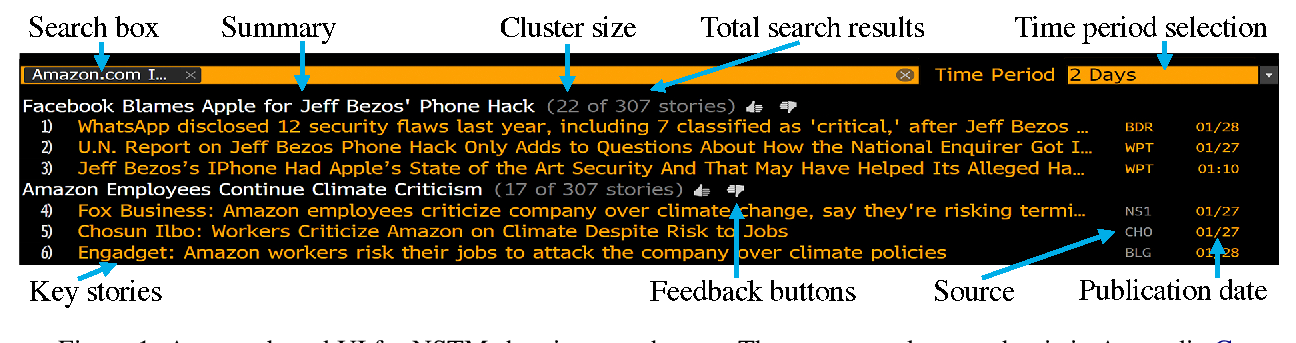