Few-shot Slot Tagging with Collapsed Dependency Transfer and Label-enhanced Task-adaptive Projection Network
Yutai Hou, Wanxiang Che, Yongkui Lai, Zhihan Zhou, Yijia Liu, Han Liu, Ting Liu
Dialogue and Interactive Systems Long Paper
Session 2B: Jul 6
(09:00-10:00 GMT)
Session 3A: Jul 6
(12:00-13:00 GMT)
Abstract:
In this paper, we explore the slot tagging with only a few labeled support sentences (a.k.a. few-shot). Few-shot slot tagging faces a unique challenge compared to the other fewshot classification problems as it calls for modeling the dependencies between labels. But it is hard to apply previously learned label dependencies to an unseen domain, due to the discrepancy of label sets. To tackle this, we introduce a collapsed dependency transfer mechanism into the conditional random field (CRF) to transfer abstract label dependency patterns as transition scores. In the few-shot setting, the emission score of CRF can be calculated as a word’s similarity to the representation of each label. To calculate such similarity, we propose a Label-enhanced Task-Adaptive Projection Network (L-TapNet) based on the state-of-the-art few-shot classification model – TapNet, by leveraging label name semantics in representing labels. Experimental results show that our model significantly outperforms the strongest few-shot learning baseline by 14.64 F1 scores in the one-shot setting.
You can open the
pre-recorded video
in a separate window.
NOTE: The SlidesLive video may display a random order of the authors.
The correct author list is shown at the top of this webpage.
Similar Papers
Shaping Visual Representations with Language for Few-Shot Classification
Jesse Mu, Percy Liang, Noah Goodman,
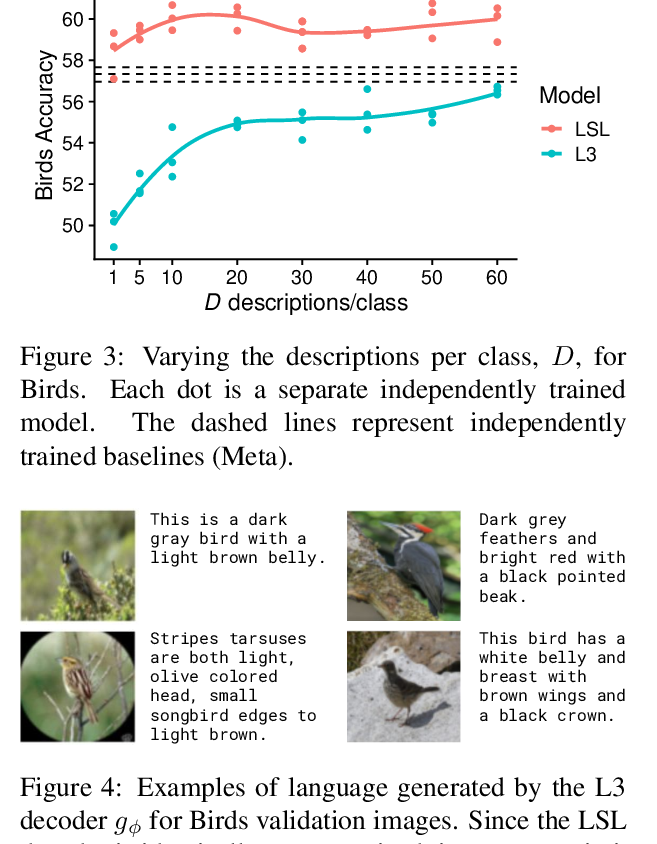
Modeling Label Semantics for Predicting Emotional Reactions
Radhika Gaonkar, Heeyoung Kwon, Mohaddeseh Bastan, Niranjan Balasubramanian, Nathanael Chambers,
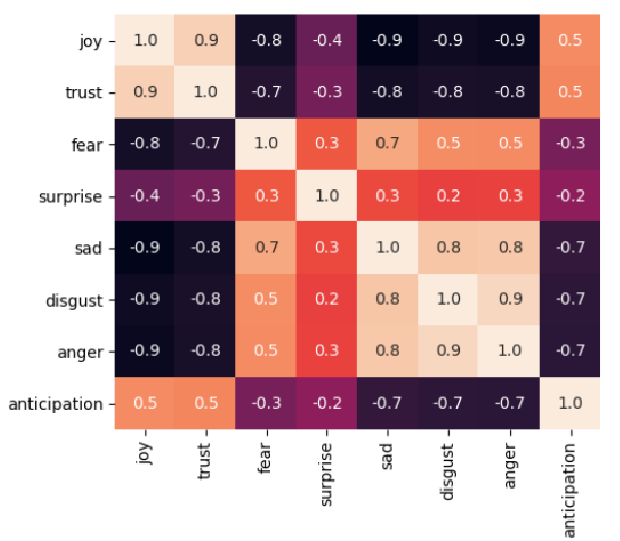
LEAN-LIFE: A Label-Efficient Annotation Framework Towards Learning from Explanation
Dong-Ho Lee, Rahul Khanna, Bill Yuchen Lin, Seyeon Lee, Qinyuan Ye, Elizabeth Boschee, Leonardo Neves, Xiang Ren,
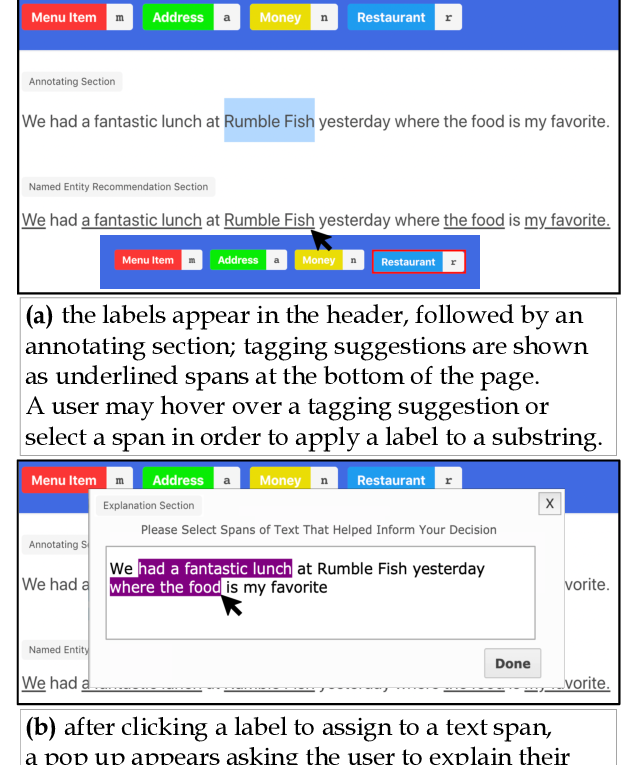
Improving Massively Multilingual Neural Machine Translation and Zero-Shot Translation
Biao Zhang, Philip Williams, Ivan Titov, Rico Sennrich,
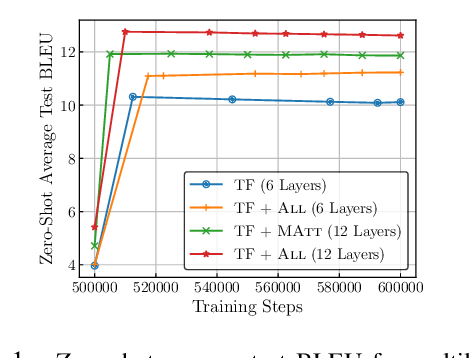