Shaping Visual Representations with Language for Few-Shot Classification
Jesse Mu, Percy Liang, Noah Goodman
Language Grounding to Vision, Robotics and Beyond Short Paper
Session 9A: Jul 7
(17:00-18:00 GMT)
Session 10B: Jul 7
(21:00-22:00 GMT)
Abstract:
By describing the features and abstractions of our world, language is a crucial tool for human learning and a promising source of supervision for machine learning models. We use language to improve few-shot visual classification in the underexplored scenario where natural language task descriptions are available during training, but unavailable for novel tasks at test time. Existing models for this setting sample new descriptions at test time and use those to classify images. Instead, we propose language-shaped learning (LSL), an end-to-end model that regularizes visual representations to predict language. LSL is conceptually simpler, more data efficient, and outperforms baselines in two challenging few-shot domains.
You can open the
pre-recorded video
in a separate window.
NOTE: The SlidesLive video may display a random order of the authors.
The correct author list is shown at the top of this webpage.
Similar Papers
Few-Shot NLG with Pre-Trained Language Model
Zhiyu Chen, Harini Eavani, Wenhu Chen, Yinyin Liu, William Yang Wang,
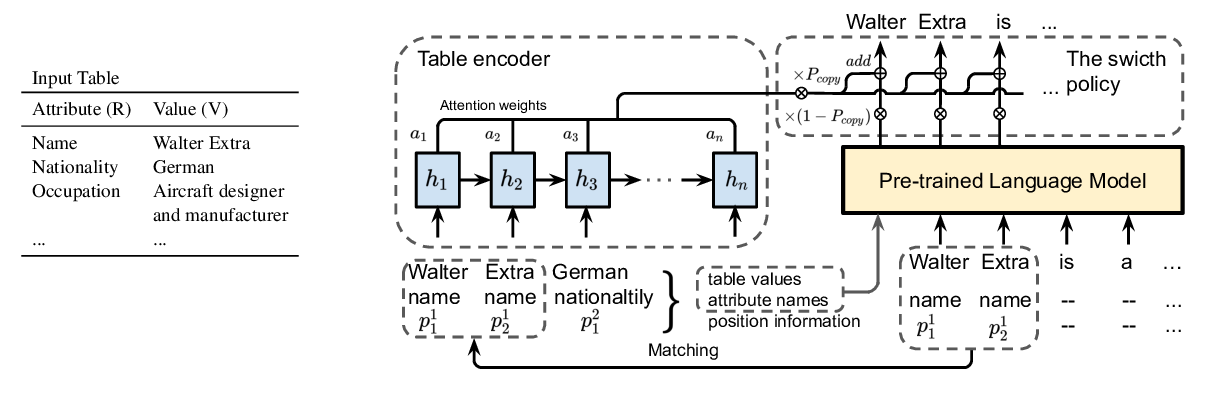
Few-shot Slot Tagging with Collapsed Dependency Transfer and Label-enhanced Task-adaptive Projection Network
Yutai Hou, Wanxiang Che, Yongkui Lai, Zhihan Zhou, Yijia Liu, Han Liu, Ting Liu,
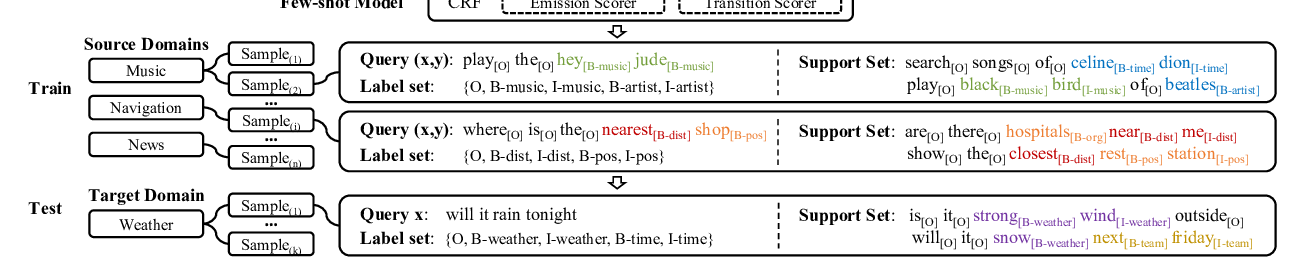
Unsupervised Multimodal Neural Machine Translation with Pseudo Visual Pivoting
Po-Yao Huang, Junjie Hu, Xiaojun Chang, Alexander Hauptmann,
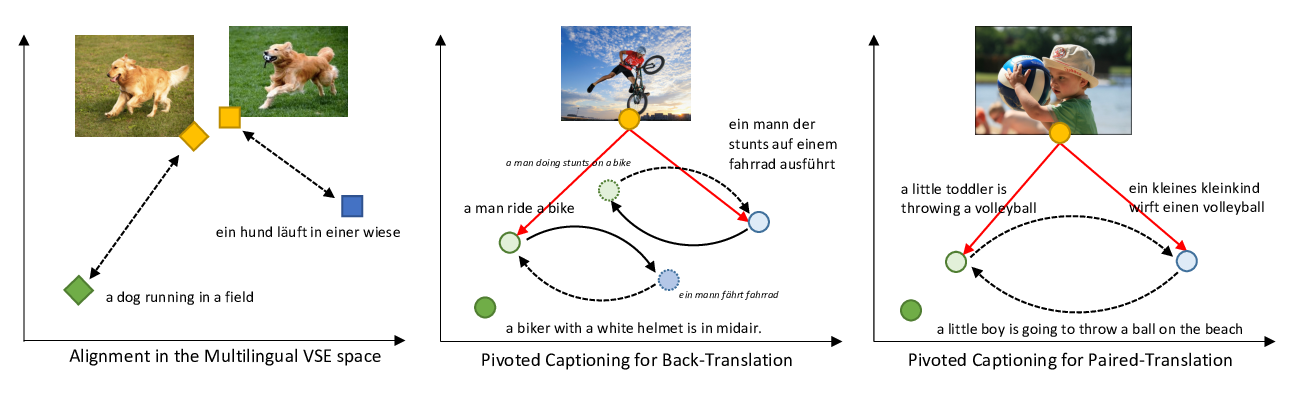
Zero-Shot Transfer Learning with Synthesized Data for Multi-Domain Dialogue State Tracking
Giovanni Campagna, Agata Foryciarz, Mehrad Moradshahi, Monica Lam,
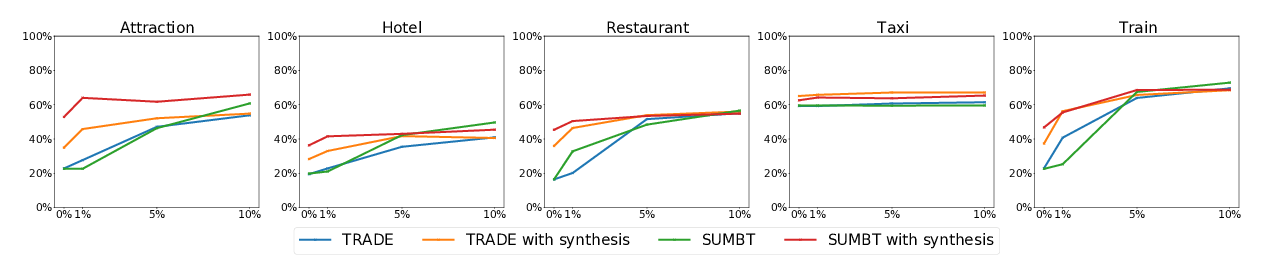