A Systematic Assessment of Syntactic Generalization in Neural Language Models
Jennifer Hu, Jon Gauthier, Peng Qian, Ethan Wilcox, Roger Levy
Cognitive Modeling and Psycholinguistics Long Paper
Session 3A: Jul 6
(12:00-13:00 GMT)
Session 5B: Jul 6
(21:00-22:00 GMT)
Abstract:
While state-of-the-art neural network models continue to achieve lower perplexity scores on language modeling benchmarks, it remains unknown whether optimizing for broad-coverage predictive performance leads to human-like syntactic knowledge. Furthermore, existing work has not provided a clear picture about the model properties required to produce proper syntactic generalizations. We present a systematic evaluation of the syntactic knowledge of neural language models, testing 20 combinations of model types and data sizes on a set of 34 English-language syntactic test suites. We find substantial differences in syntactic generalization performance by model architecture, with sequential models underperforming other architectures. Factorially manipulating model architecture and training dataset size (1M-40M words), we find that variability in syntactic generalization performance is substantially greater by architecture than by dataset size for the corpora tested in our experiments. Our results also reveal a dissociation between perplexity and syntactic generalization performance.
You can open the
pre-recorded video
in a separate window.
NOTE: The SlidesLive video may display a random order of the authors.
The correct author list is shown at the top of this webpage.
Similar Papers
Does Syntax Need to Grow on Trees? Sources of Hierarchical Inductive Bias in Sequence-to-Sequence Networks
R. Thomas McCoy, Robert Frank, Tal Linzen,
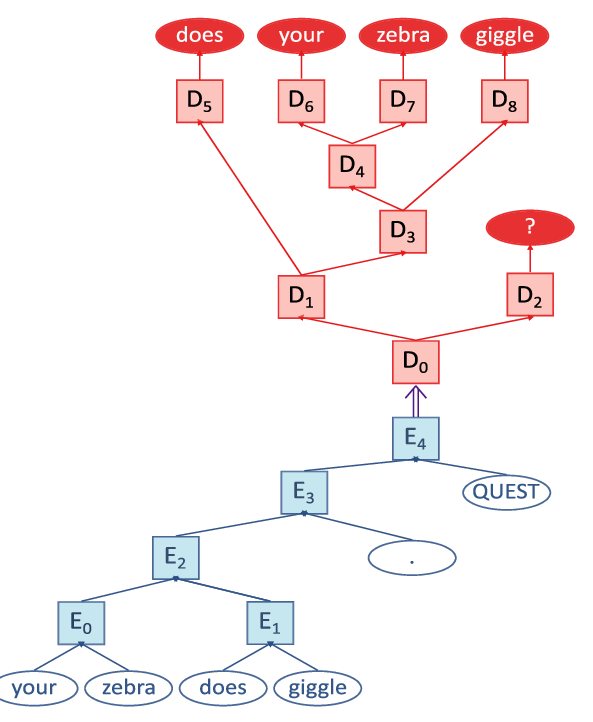
Representations of Syntax [MASK] Useful: Effects of Constituency and Dependency Structure in Recursive LSTMs
Michael Lepori, Tal Linzen, R. Thomas McCoy,
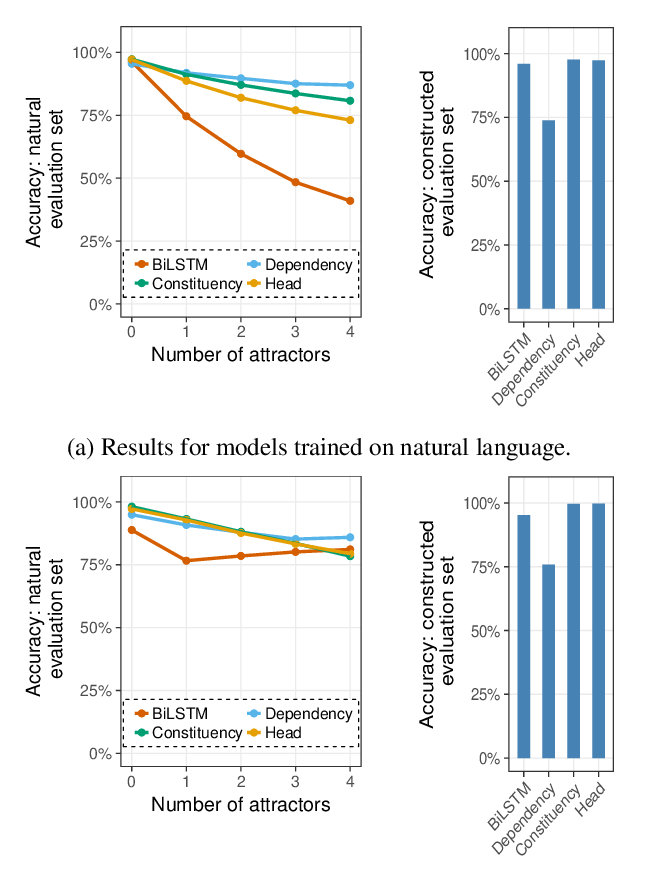
Recurrent Neural Network Language Models Always Learn English-Like Relative Clause Attachment
Forrest Davis, Marten van Schijndel,
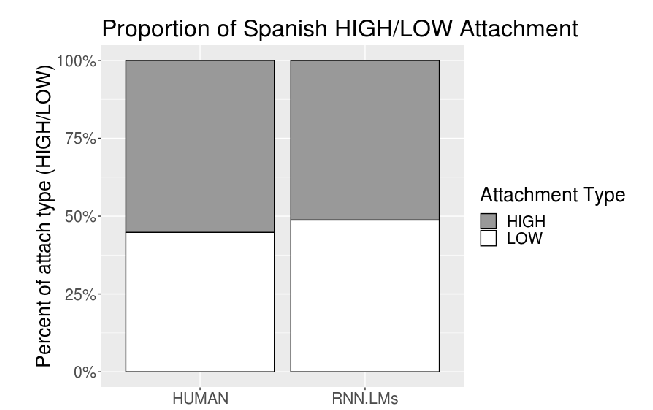
Compositional Generalization by Factorizing Alignment and Translation
Jacob Russin, Jason Jo, Randall O'Reilly, Yoshua Bengio,
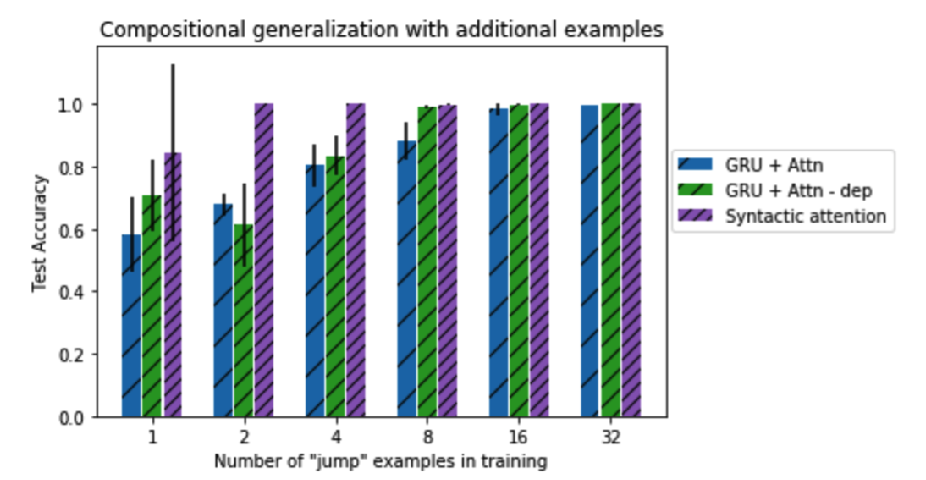