Inflecting When There's No Majority: Limitations of Encoder-Decoder Neural Networks as Cognitive Models for German Plurals
Kate McCurdy, Sharon Goldwater, Adam Lopez
Cognitive Modeling and Psycholinguistics Long Paper
Session 3A: Jul 6
(12:00-13:00 GMT)
Session 4A: Jul 6
(17:00-18:00 GMT)
Abstract:
Can artificial neural networks learn to represent inflectional morphology and generalize to new words as human speakers do? Kirov and Cotterell (2018) argue that the answer is yes: modern Encoder-Decoder (ED) architectures learn human-like behavior when inflecting English verbs, such as extending the regular past tense form /-(e)d/ to novel words. However, their work does not address the criticism raised by Marcus et al. (1995): that neural models may learn to extend not the regular, but the most frequent class — and thus fail on tasks like German number inflection, where infrequent suffixes like /-s/ can still be productively generalized. To investigate this question, we first collect a new dataset from German speakers (production and ratings of plural forms for novel nouns) that is designed to avoid sources of information unavailable to the ED model. The speaker data show high variability, and two suffixes evince 'regular' behavior, appearing more often with phonologically atypical inputs. Encoder-decoder models do generalize the most frequently produced plural class, but do not show human-like variability or 'regular' extension of these other plural markers. We conclude that modern neural models may still struggle with minority-class generalization.
You can open the
pre-recorded video
in a separate window.
NOTE: The SlidesLive video may display a random order of the authors.
The correct author list is shown at the top of this webpage.
Similar Papers
Negated and Misprimed Probes for Pretrained Language Models: Birds Can Talk, But Cannot Fly
Nora Kassner, Hinrich Schütze,
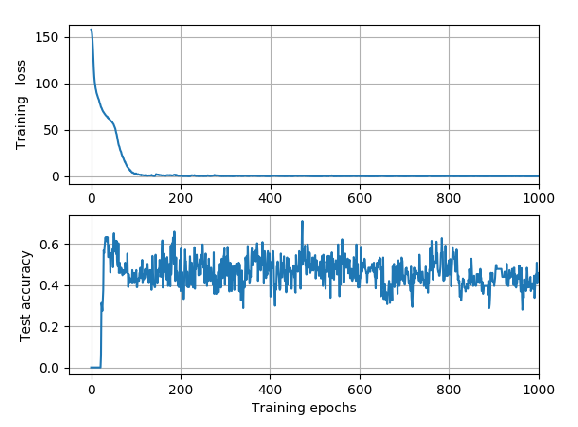
Predicting Declension Class from Form and Meaning
Adina Williams, Tiago Pimentel, Hagen Blix, Arya D. McCarthy, Eleanor Chodroff, Ryan Cotterell,
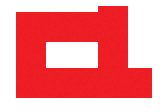
TACRED Revisited: A Thorough Evaluation of the TACRED Relation Extraction Task
Christoph Alt, Aleksandra Gabryszak, Leonhard Hennig,
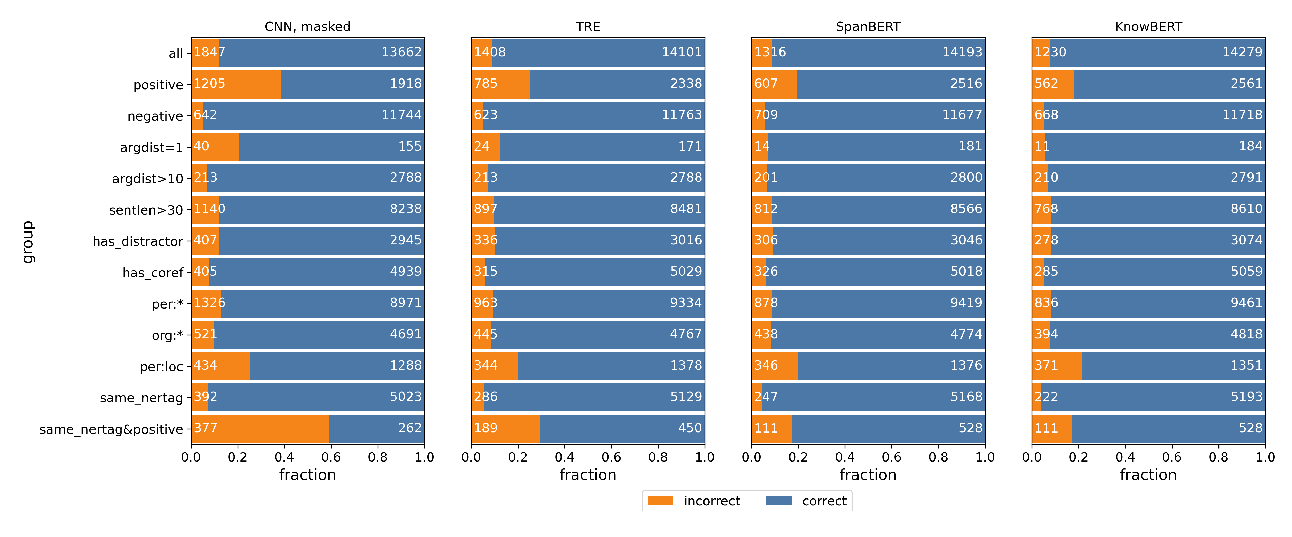