Learning to Update Natural Language Comments Based on Code Changes
Sheena Panthaplackel, Pengyu Nie, Milos Gligoric, Junyi Jessy Li, Raymond Mooney
Generation Long Paper
Session 3B: Jul 6
(13:00-14:00 GMT)
Session 5A: Jul 6
(20:00-21:00 GMT)
Abstract:
We formulate the novel task of automatically updating an existing natural language comment based on changes in the body of code it accompanies. We propose an approach that learns to correlate changes across two distinct language representations, to generate a sequence of edits that are applied to the existing comment to reflect the source code modifications. We train and evaluate our model using a dataset that we collected from commit histories of open-source software projects, with each example consisting of a concurrent update to a method and its corresponding comment. We compare our approach against multiple baselines using both automatic metrics and human evaluation. Results reflect the challenge of this task and that our model outperforms baselines with respect to making edits.
You can open the
pre-recorded video
in a separate window.
NOTE: The SlidesLive video may display a random order of the authors.
The correct author list is shown at the top of this webpage.
Similar Papers
TAG : Type Auxiliary Guiding for Code Comment Generation
Ruichu Cai, Zhihao Liang, Boyan Xu, zijian li, Yuexing Hao, Yao Chen,
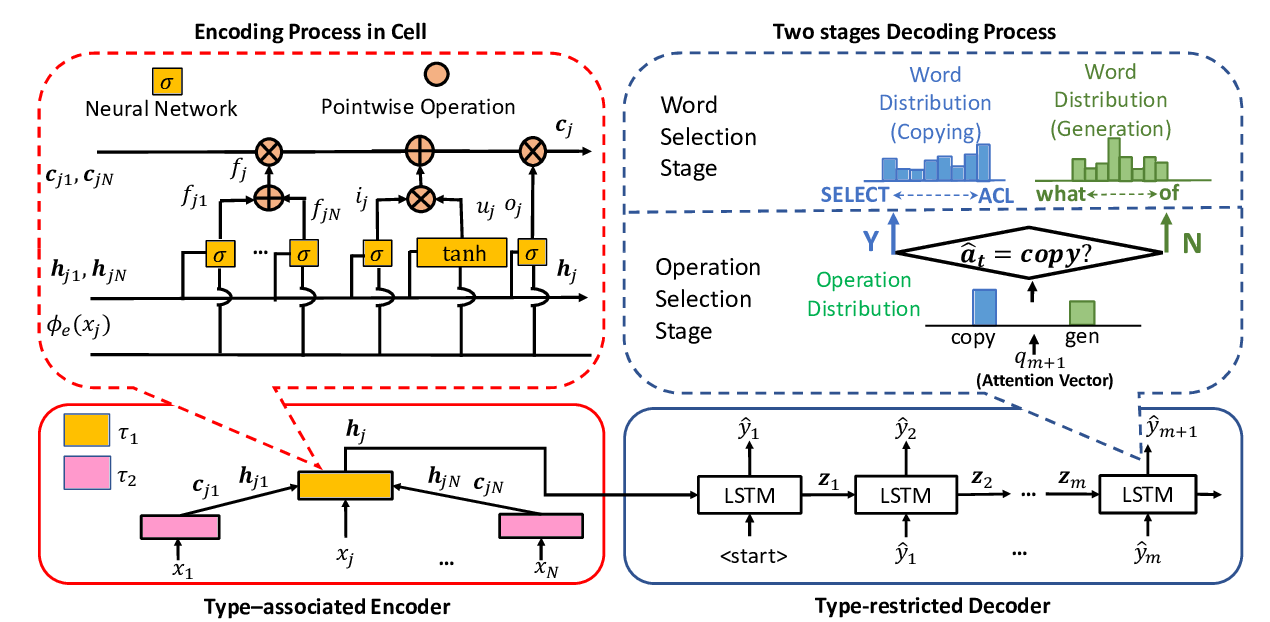
Designing Precise and Robust Dialogue Response Evaluators
Tianyu Zhao, Divesh Lala, Tatsuya Kawahara,
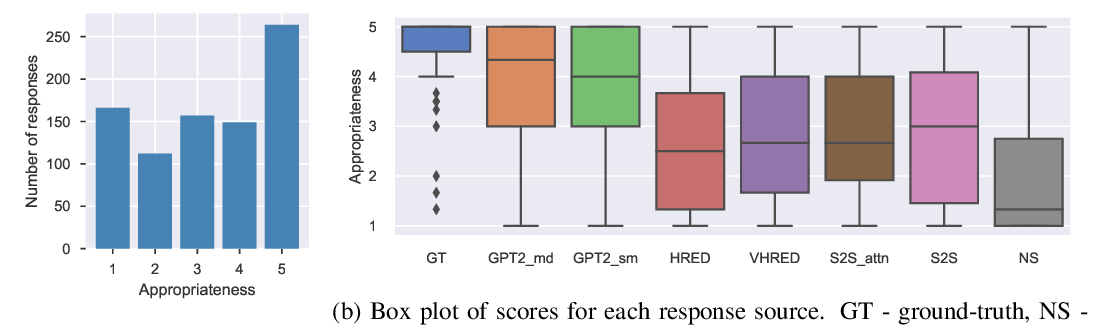