Designing Precise and Robust Dialogue Response Evaluators
Tianyu Zhao, Divesh Lala, Tatsuya Kawahara
Dialogue and Interactive Systems Short Paper
Session 1A: Jul 6
(05:00-06:00 GMT)
Session 2B: Jul 6
(09:00-10:00 GMT)
Abstract:
Automatic dialogue response evaluator has been proposed as an alternative to automated metrics and human evaluation. However, existing automatic evaluators achieve only moderate correlation with human judgement and they are not robust. In this work, we propose to build a reference-free evaluator and exploit the power of semi-supervised training and pretrained (masked) language models. Experimental results demonstrate that the proposed evaluator achieves a strong correlation (> 0.6) with human judgement and generalizes robustly to diverse responses and corpora. We open-source the code and data in https://github.com/ZHAOTING/dialog-processing.
You can open the
pre-recorded video
in a separate window.
NOTE: The SlidesLive video may display a random order of the authors.
The correct author list is shown at the top of this webpage.
Similar Papers
SUPERT: Towards New Frontiers in Unsupervised Evaluation Metrics for Multi-Document Summarization
Yang Gao, Wei Zhao, Steffen Eger,
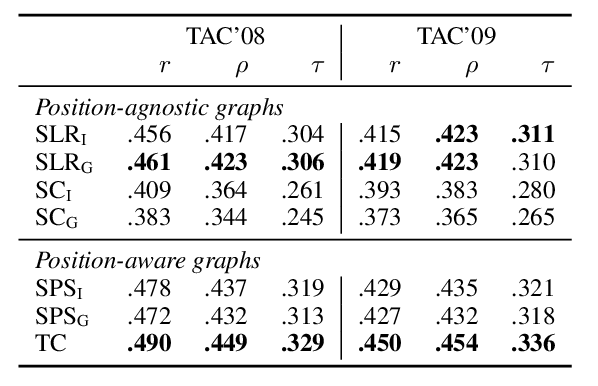
Fluent Response Generation for Conversational Question Answering
Ashutosh Baheti, Alan Ritter, Kevin Small,
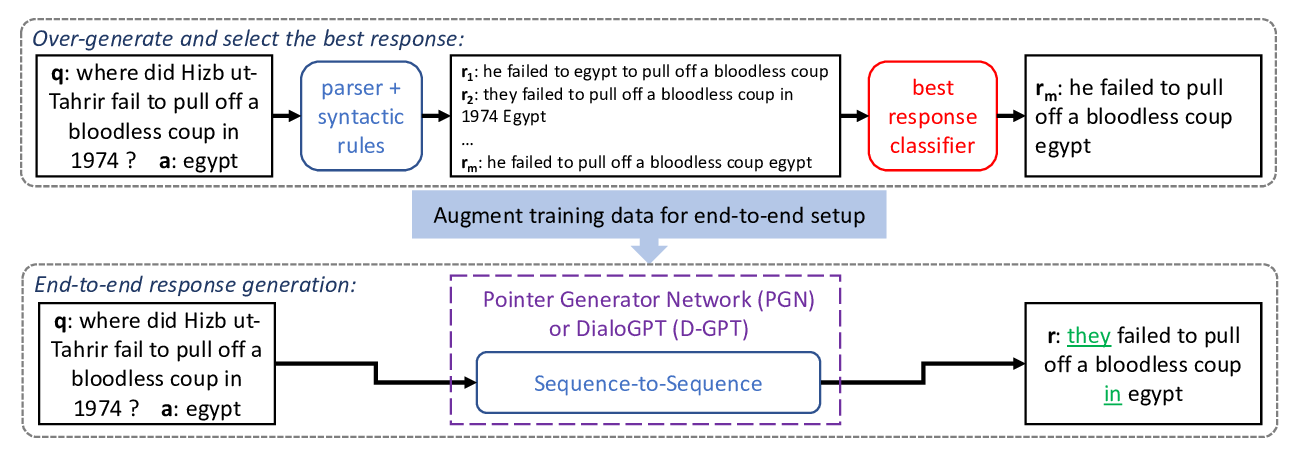
Learning an Unreferenced Metric for Online Dialogue Evaluation
Koustuv Sinha, Prasanna Parthasarathi, Jasmine Wang, Ryan Lowe, William L. Hamilton, Joelle Pineau,
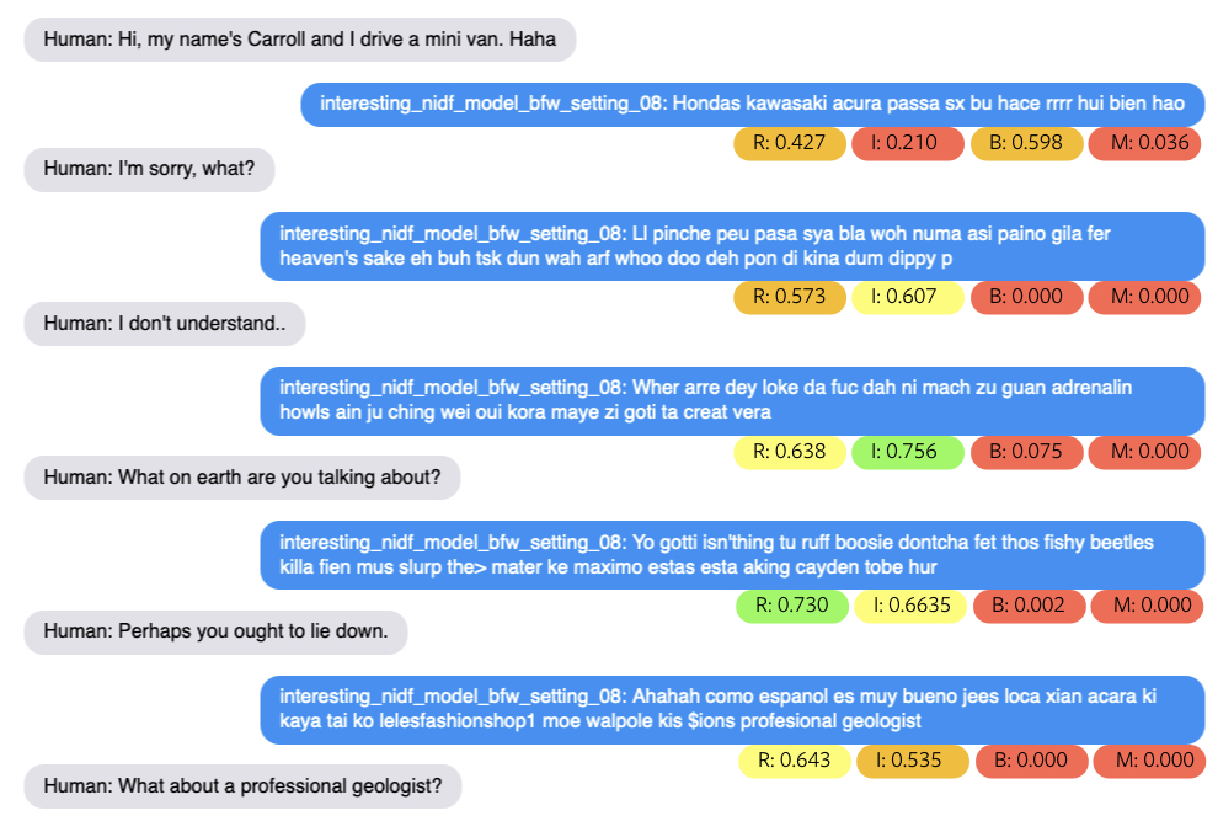
Stanza: A Python Natural Language Processing Toolkit for Many Human Languages
Peng Qi, Yuhao Zhang, Yuhui Zhang, Jason Bolton, Christopher D. Manning,
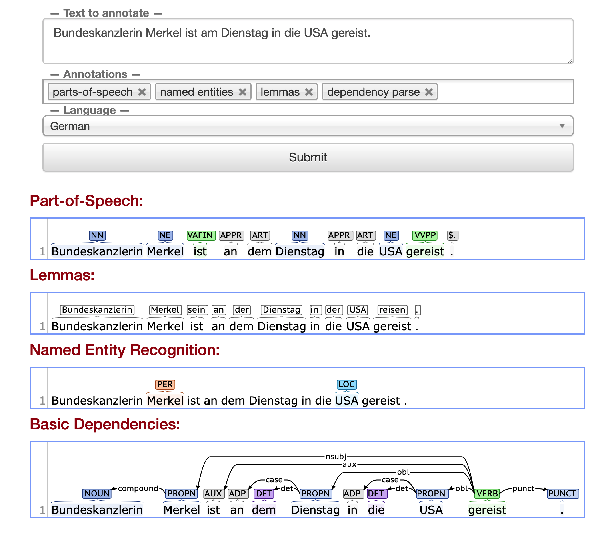