Improving Non-autoregressive Neural Machine Translation with Monolingual Data
Jiawei Zhou, Phillip Keung
Machine Translation Short Paper
Session 3B: Jul 6
(13:00-14:00 GMT)
Session 5A: Jul 6
(20:00-21:00 GMT)
Abstract:
Non-autoregressive (NAR) neural machine translation is usually done via knowledge distillation from an autoregressive (AR) model. Under this framework, we leverage large monolingual corpora to improve the NAR model's performance, with the goal of transferring the AR model's generalization ability while preventing overfitting. On top of a strong NAR baseline, our experimental results on the WMT14 En-De and WMT16 En-Ro news translation tasks confirm that monolingual data augmentation consistently improves the performance of the NAR model to approach the teacher AR model's performance, yields comparable or better results than the best non-iterative NAR methods in the literature and helps reduce overfitting in the training process.
You can open the
pre-recorded video
in a separate window.
NOTE: The SlidesLive video may display a random order of the authors.
The correct author list is shown at the top of this webpage.
Similar Papers
A Study of Non-autoregressive Model for Sequence Generation
Yi Ren, Jinglin Liu, Xu Tan, Zhou Zhao, Sheng Zhao, Tie-Yan Liu,
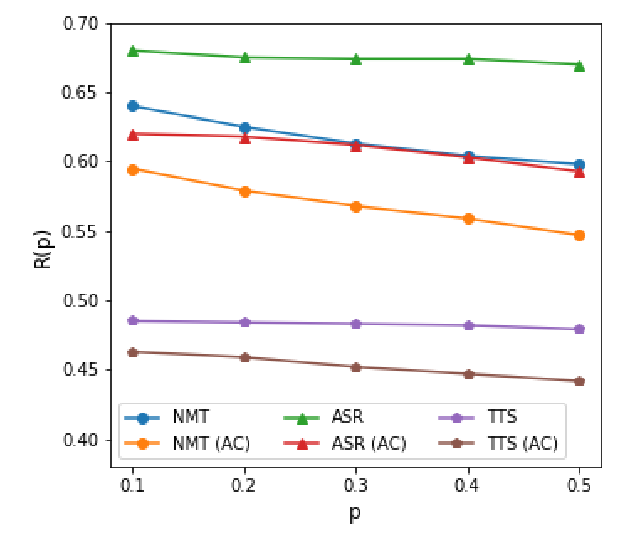
ENGINE: Energy-Based Inference Networks for Non-Autoregressive Machine Translation
Lifu Tu, Richard Yuanzhe Pang, Sam Wiseman, Kevin Gimpel,
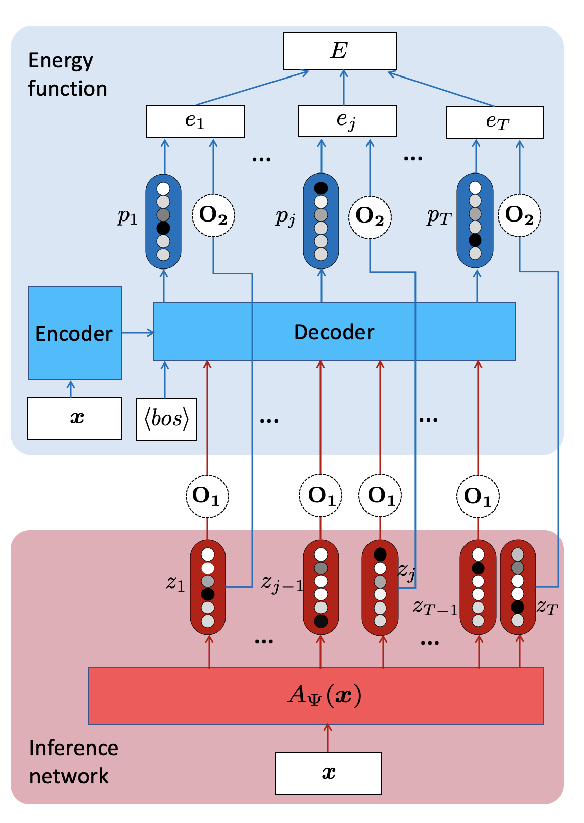
Addressing Posterior Collapse with Mutual Information for Improved Variational Neural Machine Translation
Arya D. McCarthy, Xian Li, Jiatao Gu, Ning Dong,
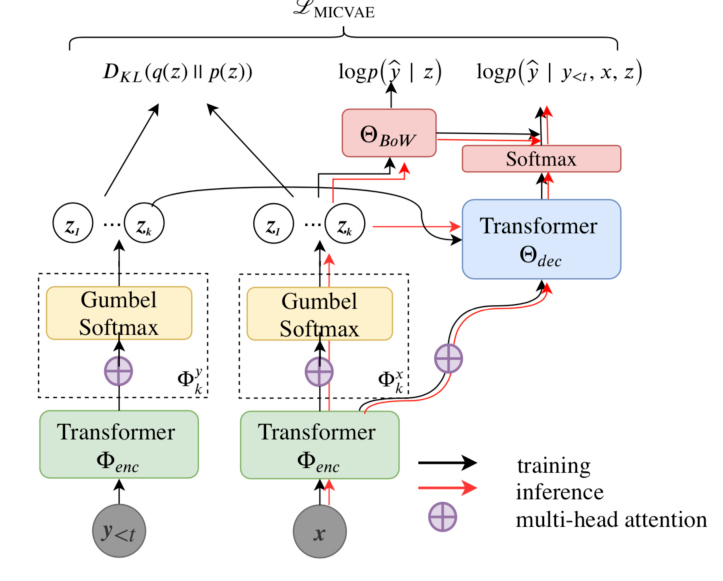
A Batch Normalized Inference Network Keeps the KL Vanishing Away
Qile Zhu, Wei Bi, Xiaojiang Liu, Xiyao Ma, Xiaolin Li, Dapeng Wu,
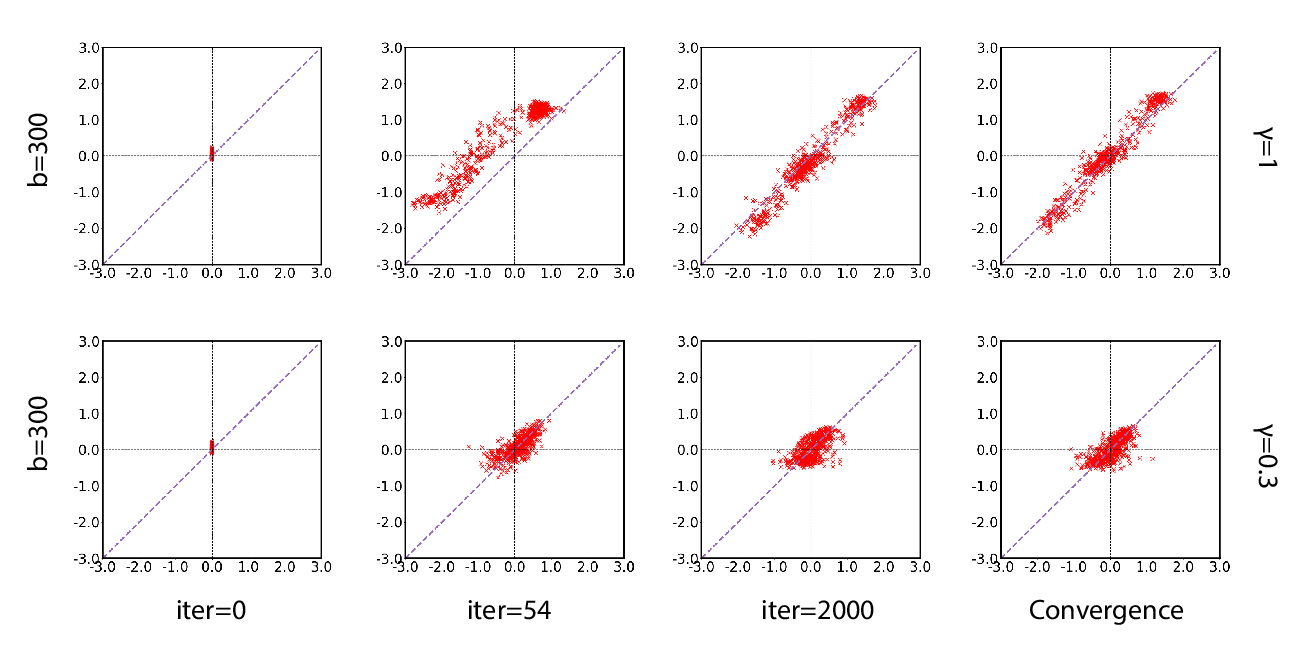