Few-Shot NLG with Pre-Trained Language Model
Zhiyu Chen, Harini Eavani, Wenhu Chen, Yinyin Liu, William Yang Wang
Generation Short Paper
Session 1A: Jul 6
(05:00-06:00 GMT)
Session 5A: Jul 6
(20:00-21:00 GMT)
Abstract:
Neural-based end-to-end approaches to natural language generation (NLG) from structured data or knowledge are data-hungry, making their adoption for real-world applications difficult with limited data. In this work, we propose the new task of few-shot natural language generation. Motivated by how humans tend to summarize tabular data, we propose a simple yet effective approach and show that it not only demonstrates strong performance but also provides good generalization across domains. The design of the model architecture is based on two aspects: content selection from input data and language modeling to compose coherent sentences, which can be acquired from prior knowledge. With just 200 training examples, across multiple domains, we show that our approach achieves very reasonable performances and outperforms the strongest baseline by an average of over 8.0 BLEU points improvement. Our code and data can be found at https://github.com/czyssrs/Few-Shot-NLG
You can open the
pre-recorded video
in a separate window.
NOTE: The SlidesLive video may display a random order of the authors.
The correct author list is shown at the top of this webpage.
Similar Papers
Incorporating External Knowledge through Pre-training for Natural Language to Code Generation
Frank F. Xu, Zhengbao Jiang, Pengcheng Yin, Bogdan Vasilescu, Graham Neubig,
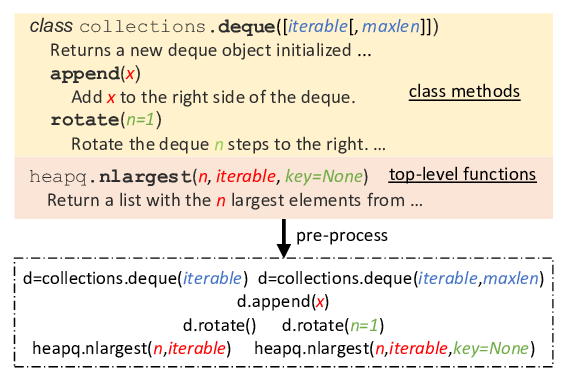
Shaping Visual Representations with Language for Few-Shot Classification
Jesse Mu, Percy Liang, Noah Goodman,
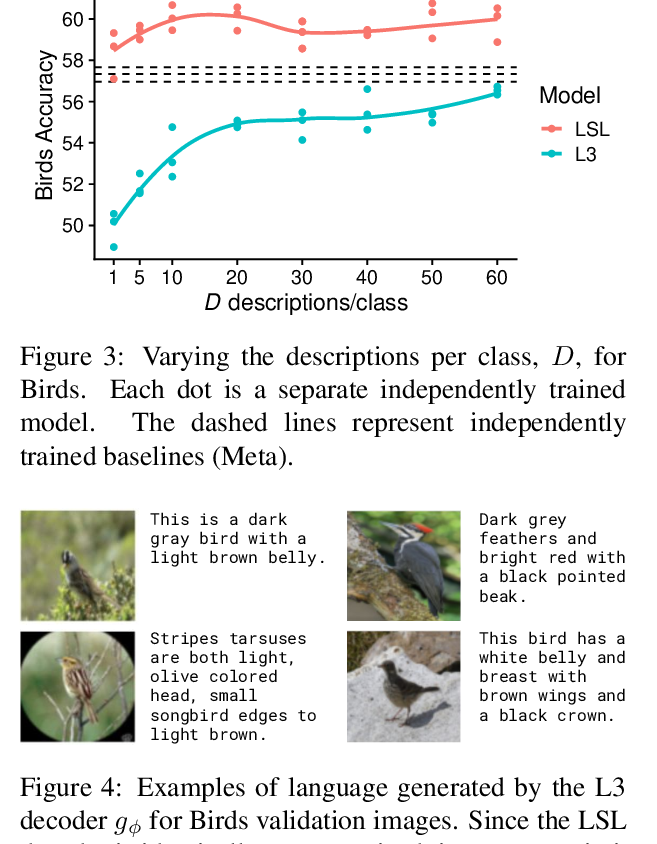
Semantic Graphs for Generating Deep Questions
Liangming Pan, Yuxi Xie, Yansong Feng, Tat-Seng Chua, Min-Yen Kan,
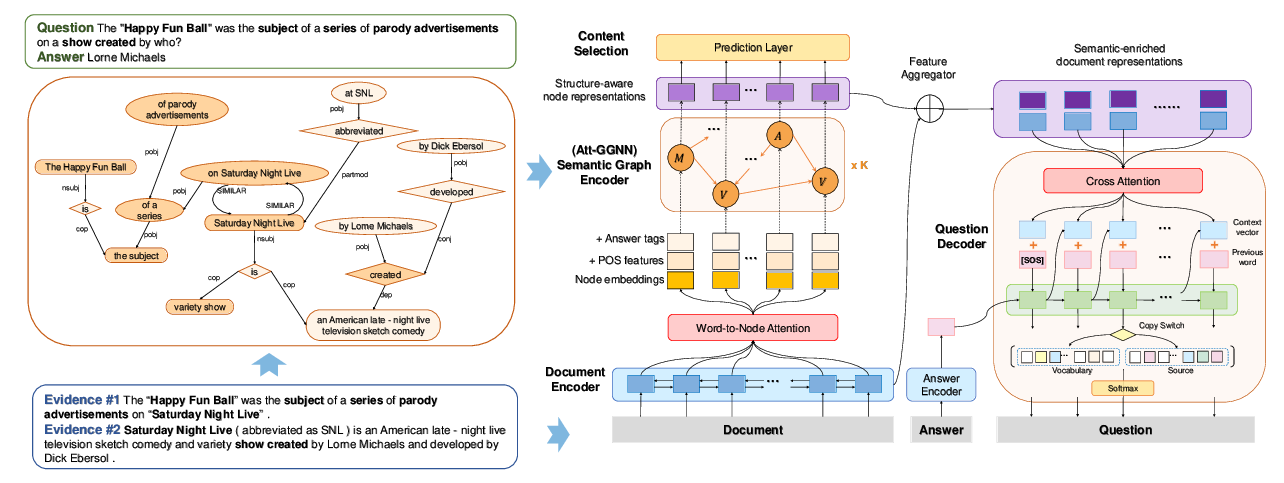
Improving Event Detection via Open-domain Trigger Knowledge
Meihan Tong, Bin Xu, Shuai Wang, Yixin Cao, Lei Hou, Juanzi Li, Jun Xie,
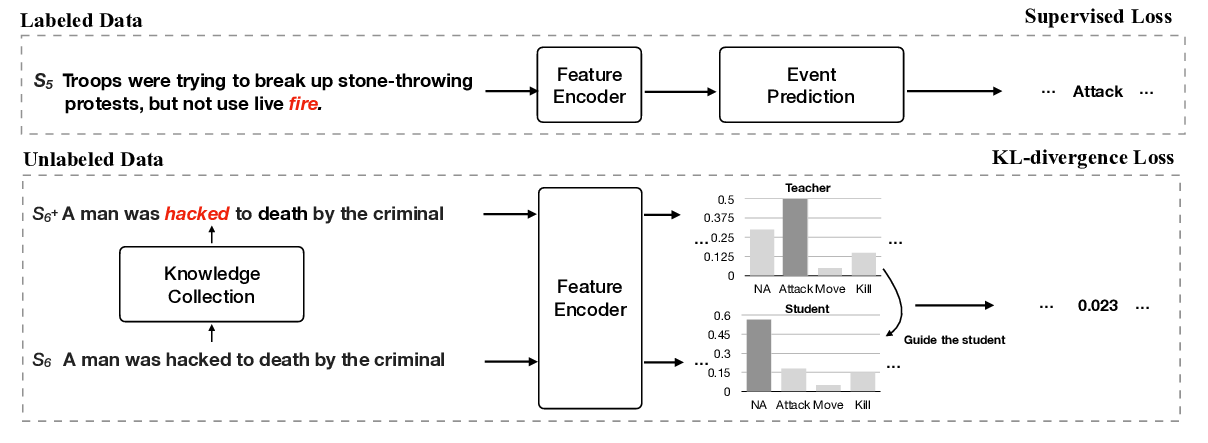