Learning to Contextually Aggregate Multi-Source Supervision for Sequence Labeling
Ouyu Lan, Xiao Huang, Bill Yuchen Lin, He Jiang, Liyuan Liu, Xiang Ren
Machine Learning for NLP Long Paper
Session 4A: Jul 6
(17:00-18:00 GMT)
Session 5A: Jul 6
(20:00-21:00 GMT)
Abstract:
Sequence labeling is a fundamental task for a range of natural language processing problems. When used in practice, its performance is largely influenced by the annotation quality and quantity, and meanwhile, obtaining ground truth labels is often costly. In many cases, ground truth labels do not exist, but noisy annotations or annotations from different domains are accessible. In this paper, we propose a novel framework Consensus Network (ConNet) that can be trained on annotations from multiple sources (e.g., crowd annotation, cross-domain data). It learns individual representation for every source and dynamically aggregates source-specific knowledge by a context-aware attention module. Finally, it leads to a model reflecting the agreement (consensus) among multiple sources. We evaluate the proposed framework in two practical settings of multi-source learning: learning with crowd annotations and unsupervised cross-domain model adaptation. Extensive experimental results show that our model achieves significant improvements over existing methods in both settings. We also demonstrate that the method can apply to various tasks and cope with different encoders.
You can open the
pre-recorded video
in a separate window.
NOTE: The SlidesLive video may display a random order of the authors.
The correct author list is shown at the top of this webpage.
Similar Papers
Single-/Multi-Source Cross-Lingual NER via Teacher-Student Learning on Unlabeled Data in Target Language
Qianhui Wu, Zijia Lin, Börje Karlsson, Jian-Guang Lou, Biqing Huang,
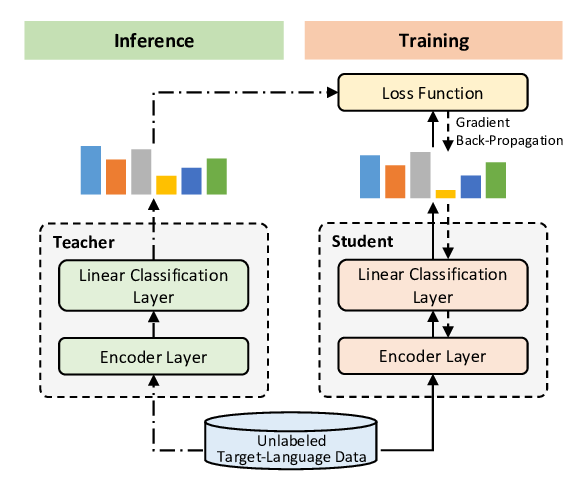
Cross-Lingual Semantic Role Labeling with High-Quality Translated Training Corpus
Hao Fei, Meishan Zhang, Donghong Ji,
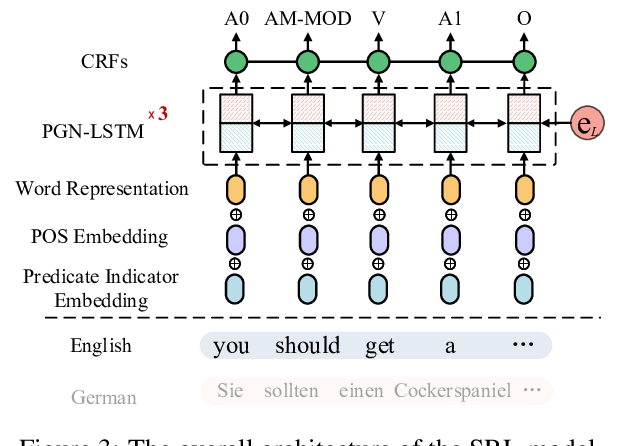
Coach: A Coarse-to-Fine Approach for Cross-domain Slot Filling
Zihan Liu, Genta Indra Winata, Peng Xu, Pascale Fung,
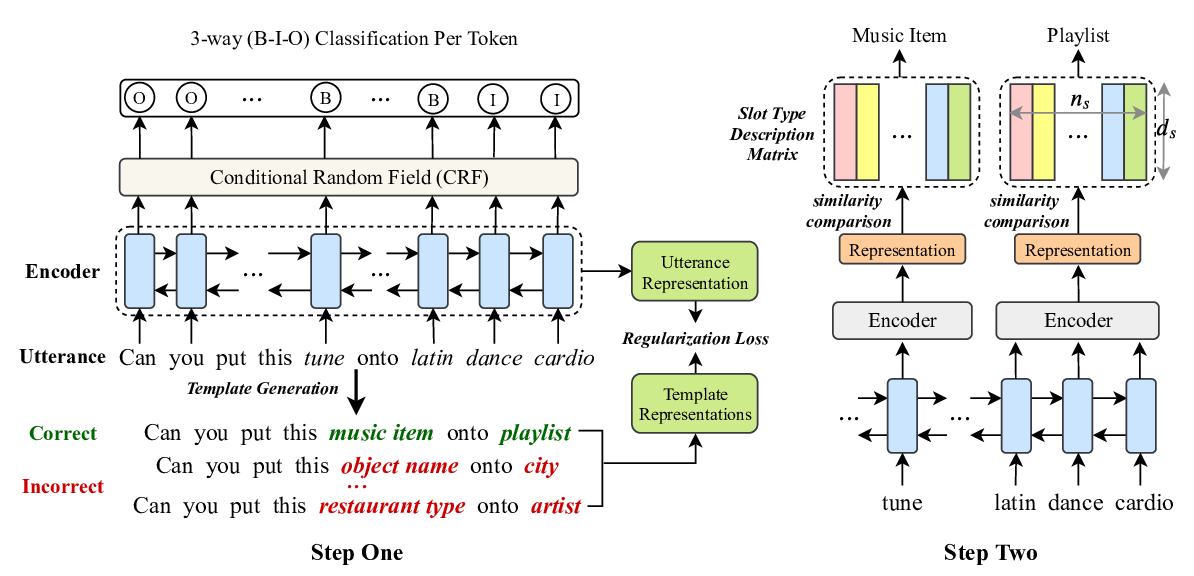
A Novel Cascade Binary Tagging Framework for Relational Triple Extraction
Zhepei Wei, Jianlin Su, Yue Wang, Yuan Tian, Yi Chang,
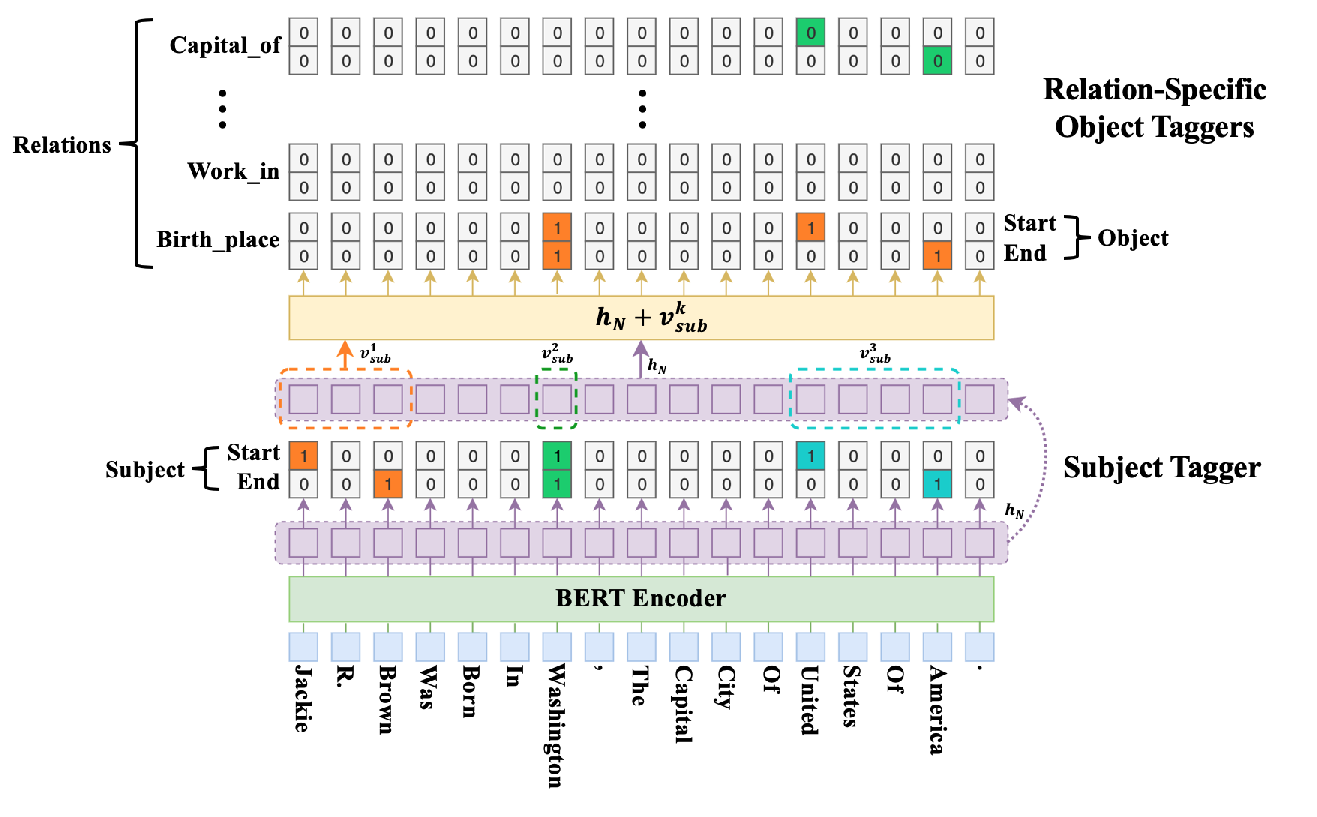