MultiQT: Multimodal learning for real-time question tracking in speech
Jakob D. Havtorn, Jan Latko, Joakim Edin, Lars Maaløe, Lasse Borgholt, Lorenzo Belgrano, Nicolai Jacobsen, Regitze Sdun, Željko Agić
Speech and Multimodality Long Paper
Session 4A: Jul 6
(17:00-18:00 GMT)
Session 5A: Jul 6
(20:00-21:00 GMT)
Abstract:
We address a challenging and practical task of labeling questions in speech in real time during telephone calls to emergency medical services in English, which embeds within a broader decision support system for emergency call-takers. We propose a novel multimodal approach to real-time sequence labeling in speech. Our model treats speech and its own textual representation as two separate modalities or views, as it jointly learns from streamed audio and its noisy transcription into text via automatic speech recognition. Our results show significant gains of jointly learning from the two modalities when compared to text or audio only, under adverse noise and limited volume of training data. The results generalize to medical symptoms detection where we observe a similar pattern of improvements with multimodal learning.
You can open the
pre-recorded video
in a separate window.
NOTE: The SlidesLive video may display a random order of the authors.
The correct author list is shown at the top of this webpage.
Similar Papers
MMPE: A Multi-Modal Interface for Post-Editing Machine Translation
Nico Herbig, Tim Düwel, Santanu Pal, Kalliopi Meladaki, Mahsa Monshizadeh, Antonio Krüger, Josef van Genabith,
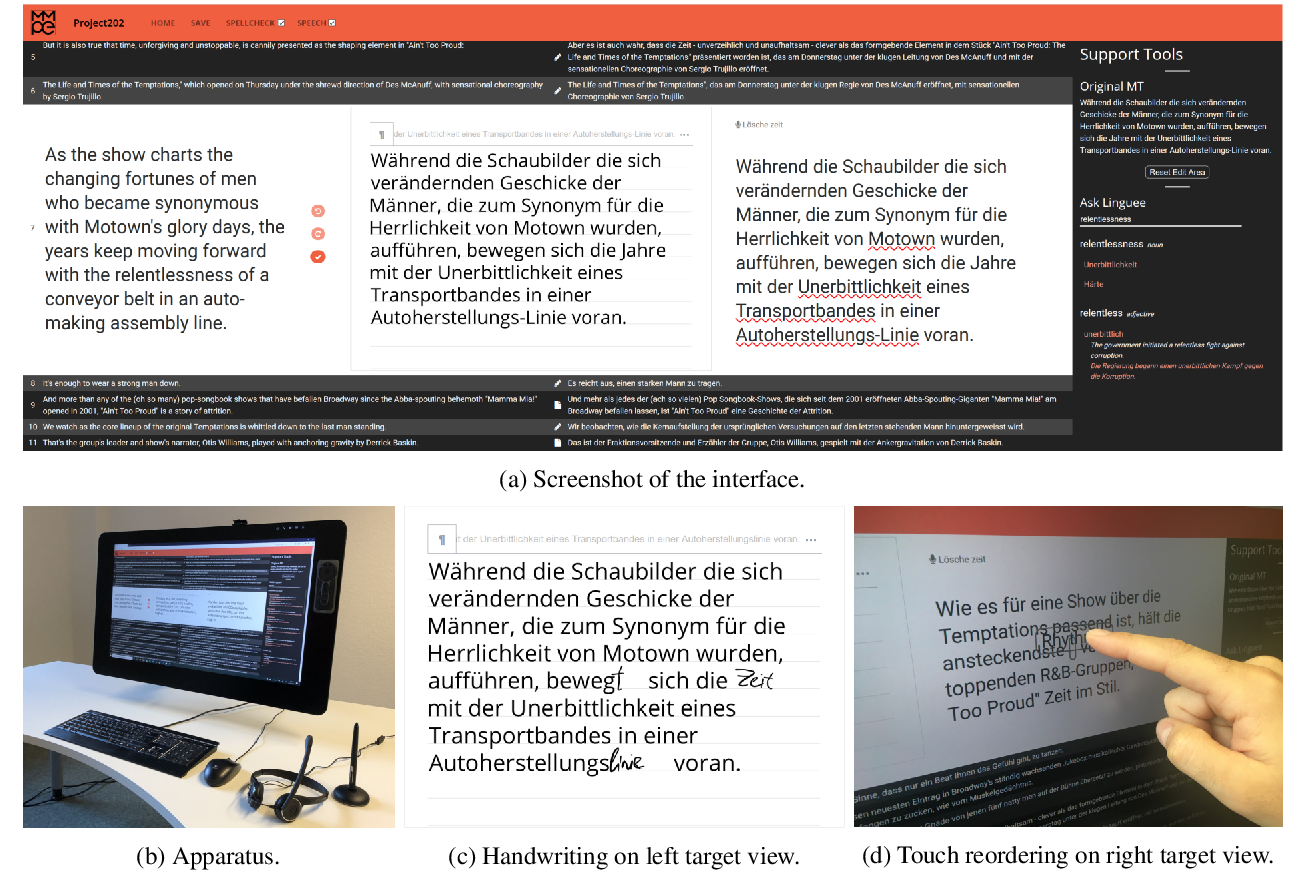
MMPE: A Multi-Modal Interface using Handwriting, Touch Reordering, and Speech Commands for Post-Editing Machine Translation
Nico Herbig, Santanu Pal, Tim Düwel, Kalliopi Meladaki, Mahsa Monshizadeh, Vladislav Hnatovskiy, Antonio Krüger, Josef van Genabith,
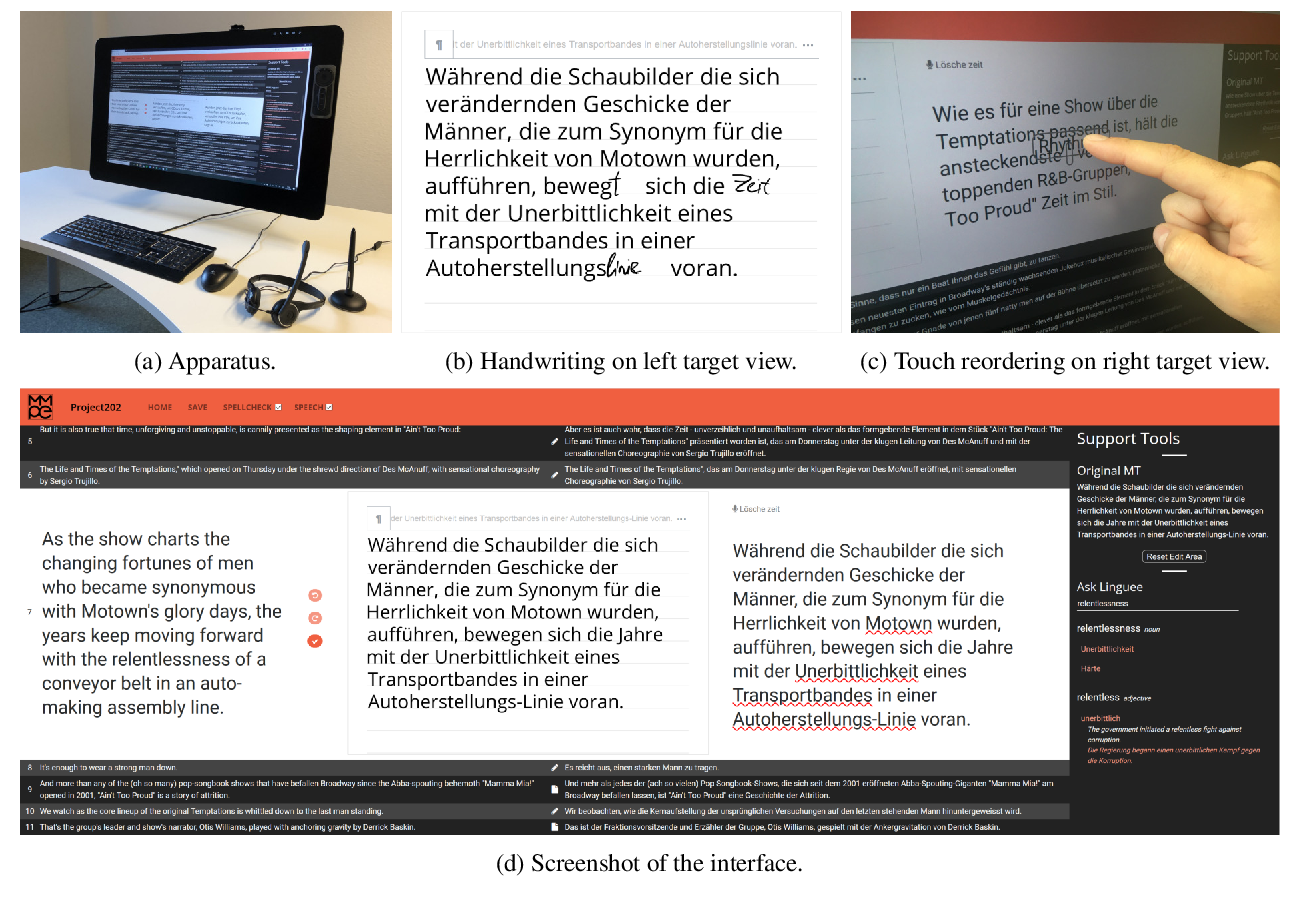