Multimodal Transformer for Multimodal Machine Translation
Shaowei Yao, Xiaojun Wan
Speech and Multimodality Short Paper
Session 7B: Jul 7
(09:00-10:00 GMT)
Session 8B: Jul 7
(13:00-14:00 GMT)
Abstract:
Multimodal Machine Translation (MMT) aims to introduce information from other modality, generally static images, to improve the translation quality. Previous works propose various incorporation methods, but most of them do not consider the relative importance of multiple modalities. Equally treating all modalities may encode too much useless information from less important modalities. In this paper, we introduce the multimodal self-attention in Transformer to solve the issues above in MMT. The proposed method learns the representation of images based on the text, which avoids encoding irrelevant information in images. Experiments and visualization analysis demonstrate that our model benefits from visual information and substantially outperforms previous works and competitive baselines in terms of various metrics.
You can open the
pre-recorded video
in a separate window.
NOTE: The SlidesLive video may display a random order of the authors.
The correct author list is shown at the top of this webpage.
Similar Papers
Unsupervised Multimodal Neural Machine Translation with Pseudo Visual Pivoting
Po-Yao Huang, Junjie Hu, Xiaojun Chang, Alexander Hauptmann,
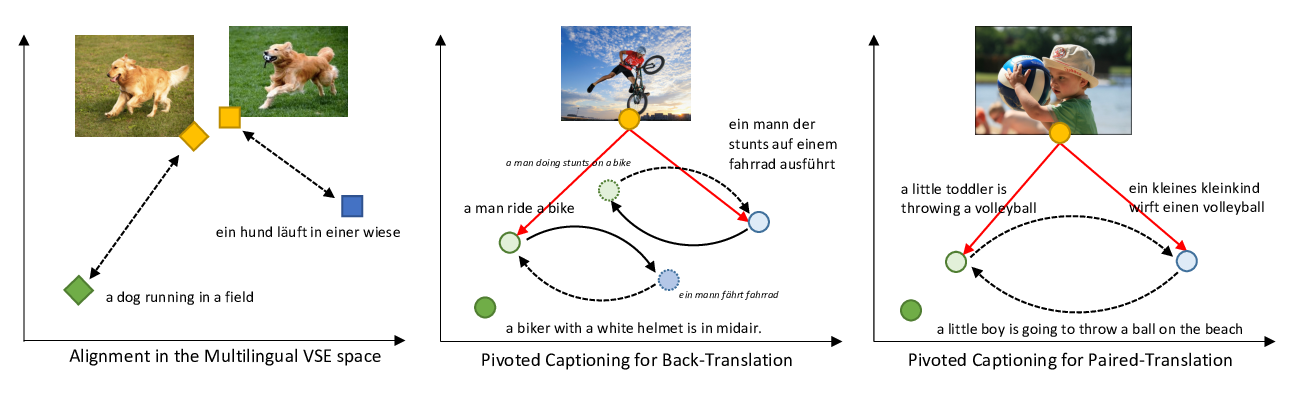
CH-SIMS: A Chinese Multimodal Sentiment Analysis Dataset with Fine-grained Annotation of Modality
Wenmeng Yu, Hua Xu, Fanyang Meng, Yilin Zhu, Yixiao Ma, Jiele Wu, Jiyun Zou, Kaicheng Yang,
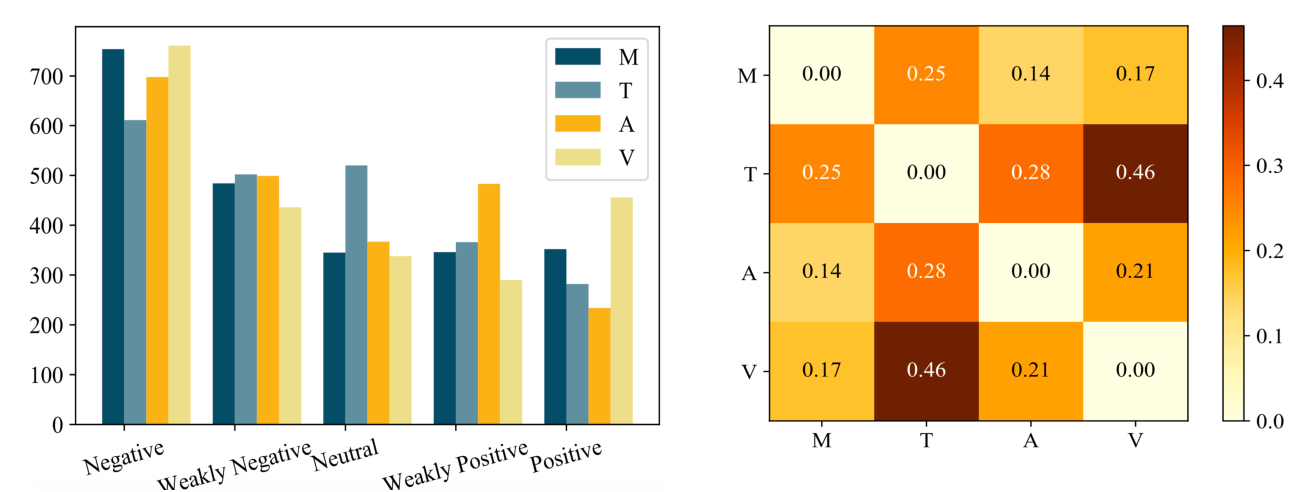
MultiQT: Multimodal learning for real-time question tracking in speech
Jakob D. Havtorn, Jan Latko, Joakim Edin, Lars Maaløe, Lasse Borgholt, Lorenzo Belgrano, Nicolai Jacobsen, Regitze Sdun, Željko Agić,
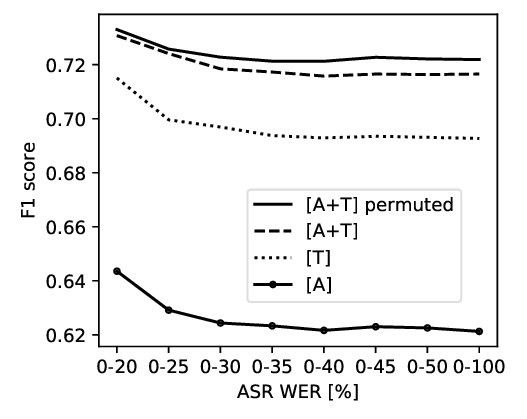