Hiring Now: A Skill-Aware Multi-Attention Model for Job Posting Generation
Liting Liu, Jie Liu, Wenzheng Zhang, Ziming Chi, Wenxuan Shi, Yalou Huang
NLP Applications Long Paper
Session 6A: Jul 7
(05:00-06:00 GMT)
Session 8B: Jul 7
(13:00-14:00 GMT)
Abstract:
Writing a good job posting is a critical step in the recruiting process, but the task is often more difficult than many people think. It is challenging to specify the level of education, experience, relevant skills per the company information and job description. To this end, we propose a novel task of Job Posting Generation (JPG) which is cast as a conditional text generation problem to generate job requirements according to the job descriptions. To deal with this task, we devise a data-driven global Skill-Aware Multi-Attention generation model, named SAMA. Specifically, to model the complex mapping relationships between input and output, we design a hierarchical decoder that we first label the job description with multiple skills, then we generate a complete text guided by the skill labels. At the same time, to exploit the prior knowledge about the skills, we further construct a skill knowledge graph to capture the global prior knowledge of skills and refine the generated results. The proposed approach is evaluated on real-world job posting data. Experimental results clearly demonstrate the effectiveness of the proposed method.
You can open the
pre-recorded video
in a separate window.
NOTE: The SlidesLive video may display a random order of the authors.
The correct author list is shown at the top of this webpage.
Similar Papers
A Knowledge-Enhanced Pretraining Model for Commonsense Story Generation
Jian Guan, Fei Huang, Minlie Huang, Zhihao Zhao, Xiaoyan Zhu,
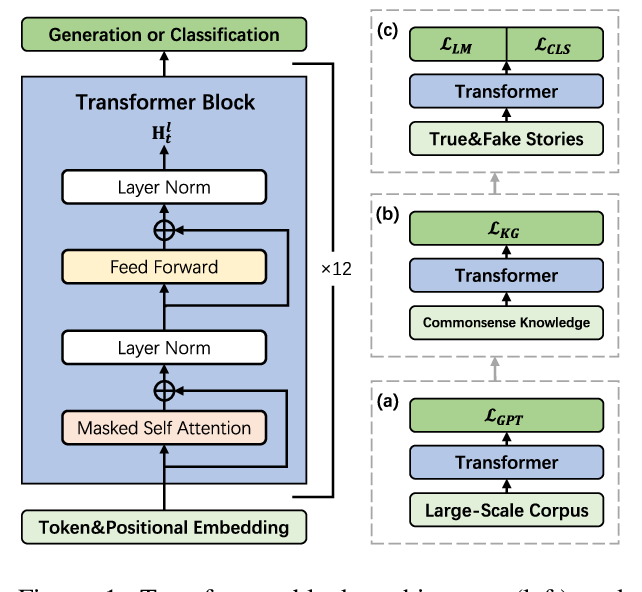
Neural Temporal Opinion Modelling for Opinion Prediction on Twitter
Lixing Zhu, Yulan He, Deyu Zhou,
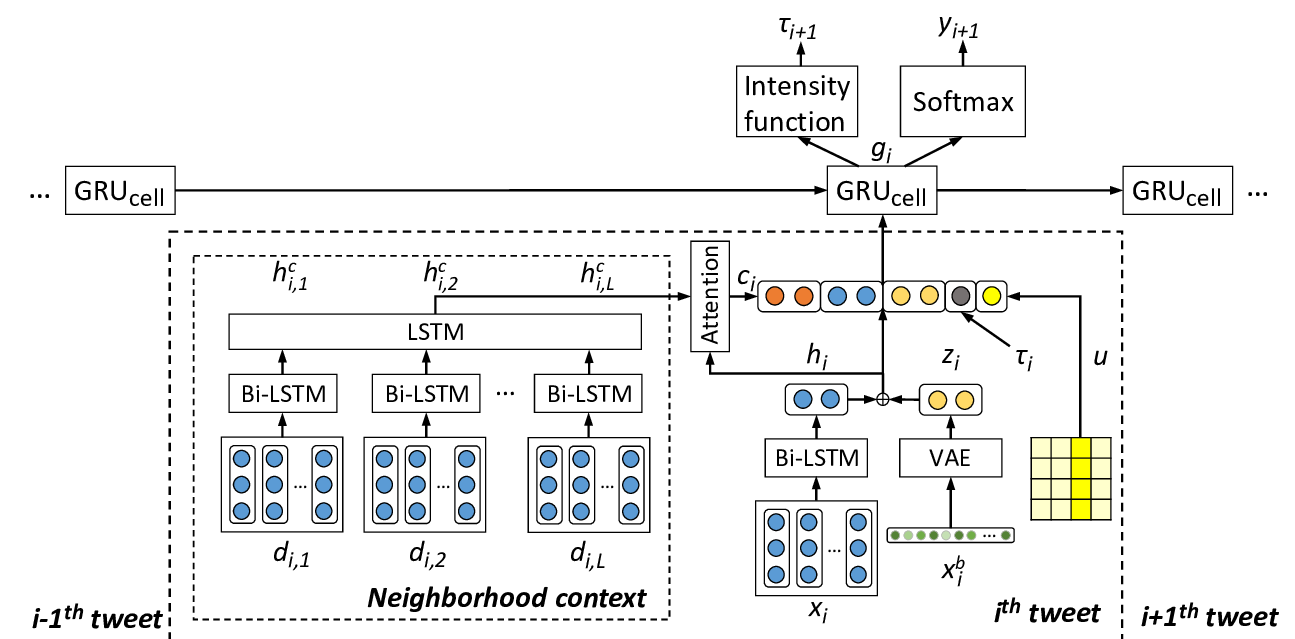
Low-Resource Generation of Multi-hop Reasoning Questions
Jianxing Yu, Wei Liu, Shuang Qiu, Qinliang Su, Kai Wang, Xiaojun Quan, Jian Yin,
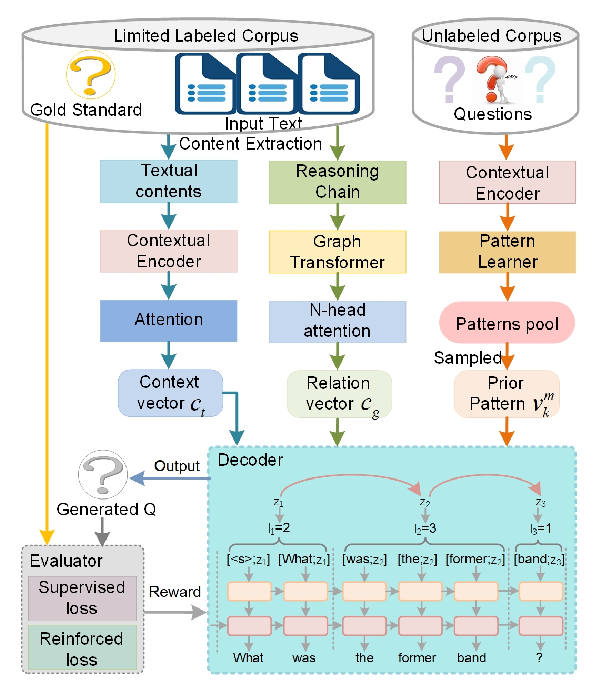