Towards Better Non-Tree Argument Mining: Proposition-Level Biaffine Parsing with Task-Specific Parameterization
Gaku Morio, Hiroaki Ozaki, Terufumi Morishita, Yuta Koreeda, Kohsuke Yanai
Sentiment Analysis, Stylistic Analysis, and Argument Mining Short Paper
Session 6A: Jul 7
(05:00-06:00 GMT)
Session 7A: Jul 7
(08:00-09:00 GMT)
Abstract:
State-of-the-art argument mining studies have advanced the techniques for predicting argument structures. However, the technology for capturing non-tree-structured arguments is still in its infancy. In this paper, we focus on non-tree argument mining with a neural model. We jointly predict proposition types and edges between propositions. Our proposed model incorporates (i) task-specific parameterization (TSP) that effectively encodes a sequence of propositions and (ii) a proposition-level biaffine attention (PLBA) that can predict a non-tree argument consisting of edges. Experimental results show that both TSP and PLBA boost edge prediction performance compared to baselines.
You can open the
pre-recorded video
in a separate window.
NOTE: The SlidesLive video may display a random order of the authors.
The correct author list is shown at the top of this webpage.
Similar Papers
Neural Temporal Opinion Modelling for Opinion Prediction on Twitter
Lixing Zhu, Yulan He, Deyu Zhou,
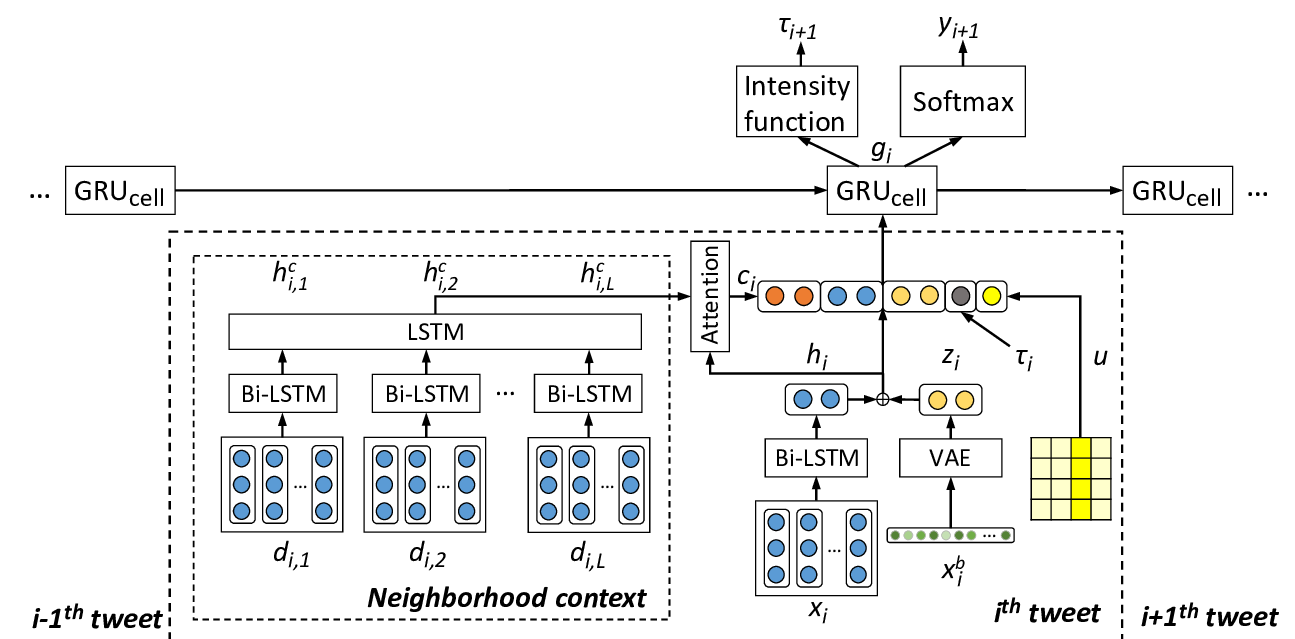
TransS-Driven Joint Learning Architecture for Implicit Discourse Relation Recognition
Ruifang He, Jian Wang, Fengyu Guo, Yugui Han,
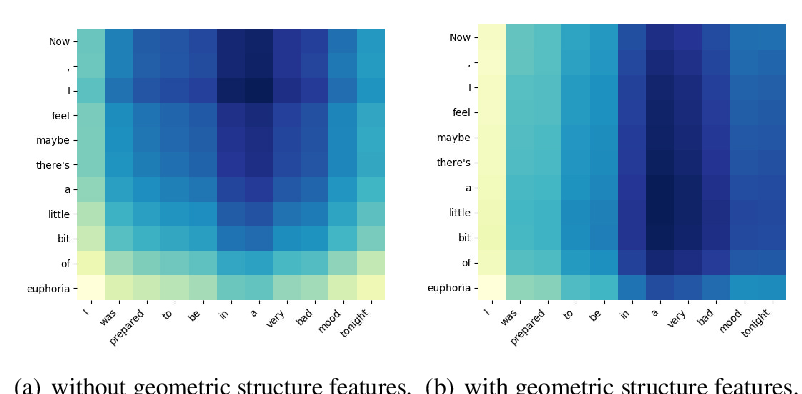
Hierarchical Entity Typing via Multi-level Learning to Rank
Tongfei Chen, Yunmo Chen, Benjamin Van Durme,
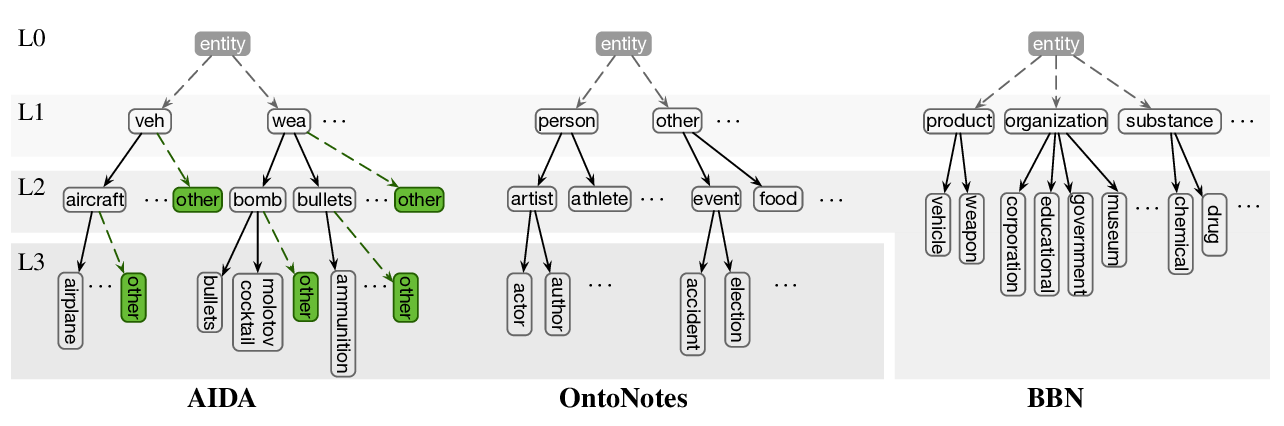
Fast and Accurate Non-Projective Dependency Tree Linearization
Xiang Yu, Simon Tannert, Ngoc Thang Vu, Jonas Kuhn,
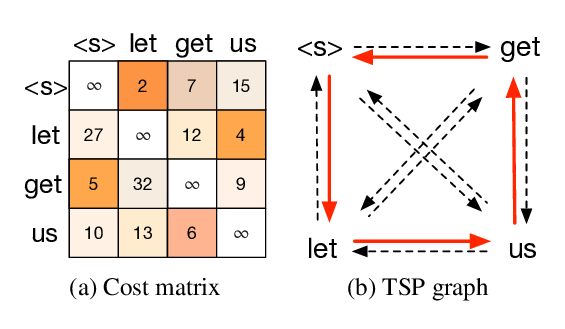