TransS-Driven Joint Learning Architecture for Implicit Discourse Relation Recognition
Ruifang He, Jian Wang, Fengyu Guo, Yugui Han
Discourse and Pragmatics Long Paper
Session 1A: Jul 6
(05:00-06:00 GMT)
Session 3B: Jul 6
(13:00-14:00 GMT)
Abstract:
Implicit discourse relation recognition is a challenging task due to the lack of connectives as strong linguistic clues. Previous methods primarily encode two arguments separately or extract the specific interaction patterns for the task, which have not fully exploited the annotated relation signal. Therefore, we propose a novel TransS-driven joint learning architecture to address the issues. Specifically, based on the multi-level encoder, we 1) translate discourse relations in low-dimensional embedding space (called TransS), which could mine the latent geometric structure information of argument-relation instances; 2) further exploit the semantic features of arguments to assist discourse understanding; 3) jointly learn 1) and 2) to mutually reinforce each other to obtain the better argument representations, so as to improve the performance of the task. Extensive experimental results on the Penn Discourse TreeBank (PDTB) show that our model achieves competitive results against several state-of-the-art systems.
You can open the
pre-recorded video
in a separate window.
NOTE: The SlidesLive video may display a random order of the authors.
The correct author list is shown at the top of this webpage.
Similar Papers
Implicit Discourse Relation Classification: We Need to Talk about Evaluation
Najoung Kim, Song Feng, Chulaka Gunasekara, Luis Lastras,
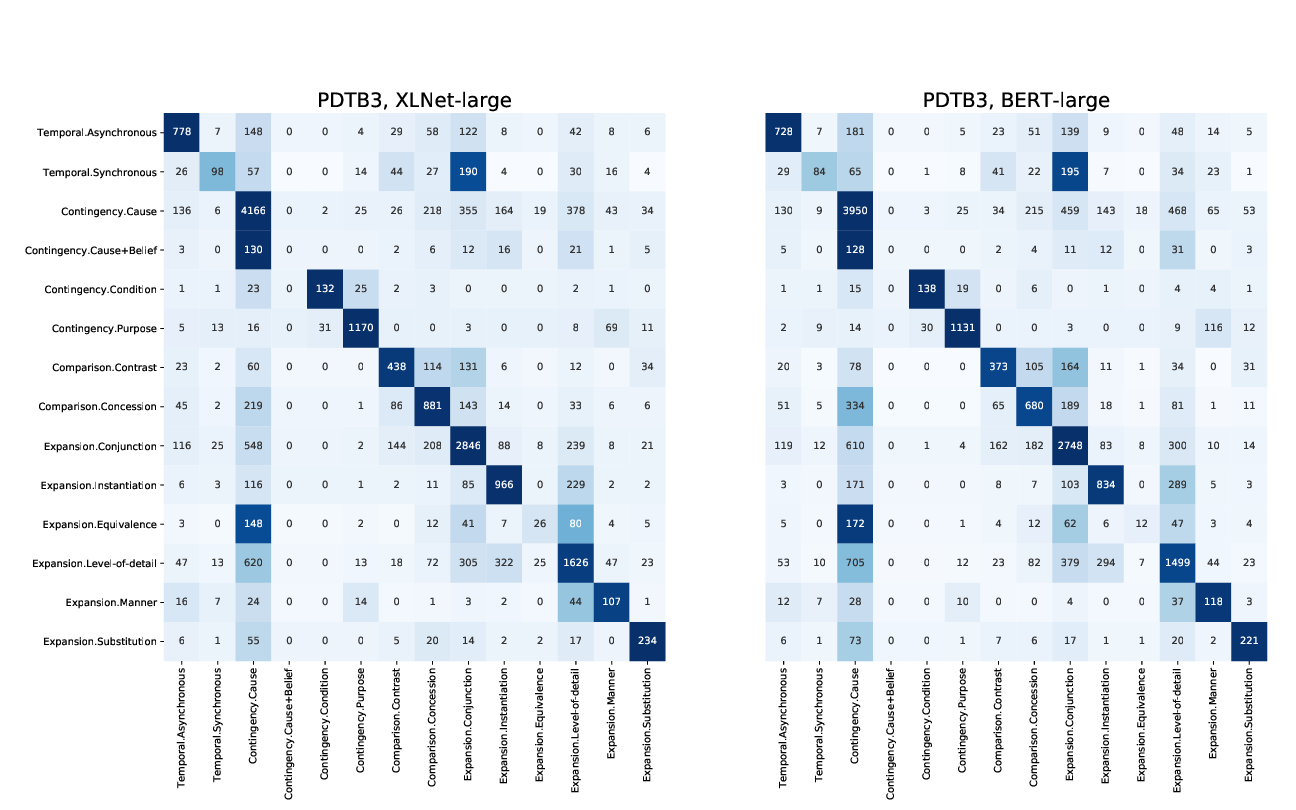
Cross-media Structured Common Space for Multimedia Event Extraction
Manling Li, Alireza Zareian, Qi Zeng, Spencer Whitehead, Di Lu, Heng Ji, Shih-Fu Chang,
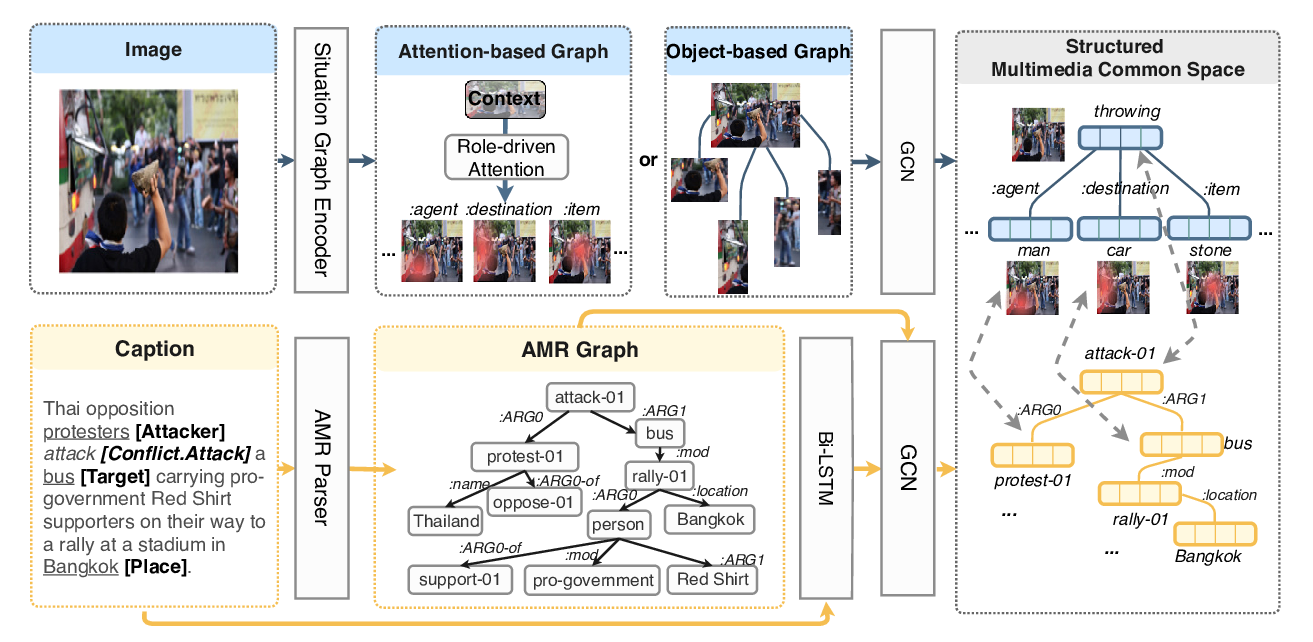
ReInceptionE: Relation-Aware Inception Network with Joint Local-Global Structural Information for Knowledge Graph Embedding
Zhiwen Xie, Guangyou Zhou, Jin Liu, Jimmy Xiangji Huang,
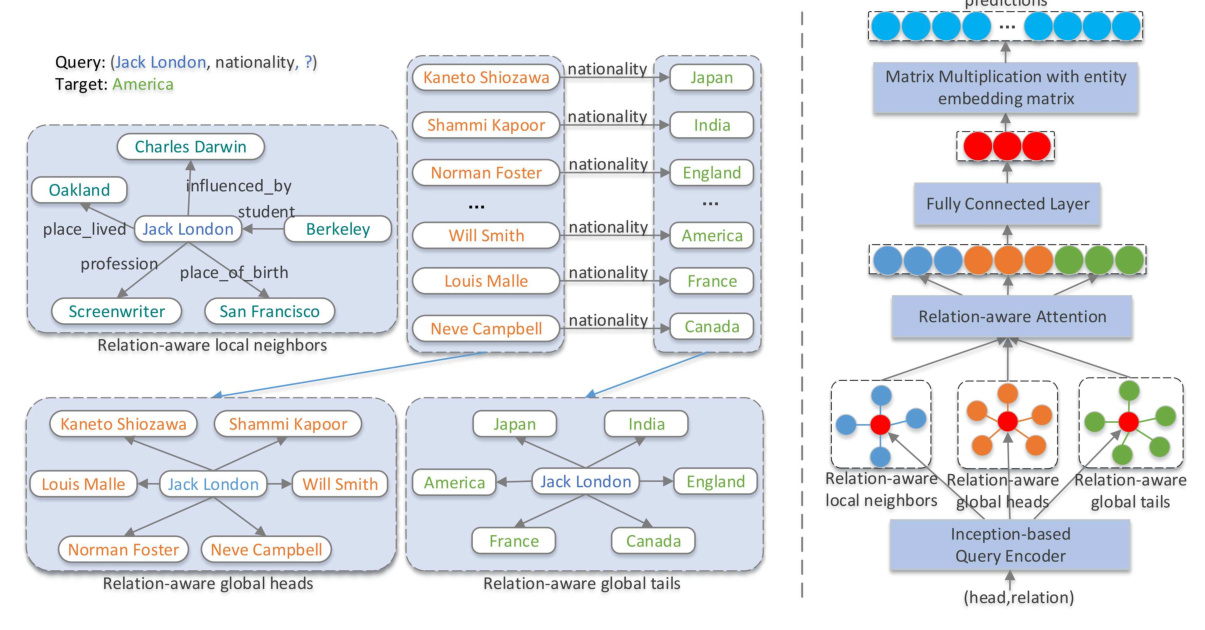
Orthogonal Relation Transforms with Graph Context Modeling for Knowledge Graph Embedding
Yun Tang, Jing Huang, Guangtao Wang, Xiaodong He, Bowen Zhou,
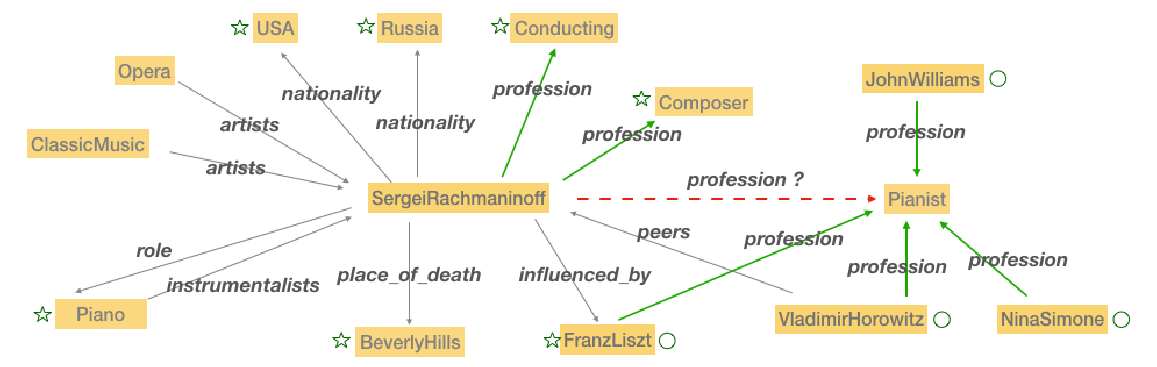