Enhancing Pre-trained Chinese Character Representation with Word-aligned Attention
Yanzeng Li, Bowen Yu, Xue Mengge, Tingwen Liu
Machine Learning for NLP Short Paper
Session 6B: Jul 7
(06:00-07:00 GMT)
Session 7B: Jul 7
(09:00-10:00 GMT)
Abstract:
Most Chinese pre-trained models take character as the basic unit and learn representation according to character's external contexts, ignoring the semantics expressed in the word, which is the smallest meaningful utterance in Chinese. Hence, we propose a novel word-aligned attention to exploit explicit word information, which is complementary to various character-based Chinese pre-trained language models. Specifically, we devise a pooling mechanism to align the character-level attention to the word level and propose to alleviate the potential issue of segmentation error propagation by multi-source information fusion. As a result, word and character information are explicitly integrated at the fine-tuning procedure. Experimental results on five Chinese NLP benchmark tasks demonstrate that our method achieves significant improvements against BERT, ERNIE and BERT-wwm.
You can open the
pre-recorded video
in a separate window.
NOTE: The SlidesLive video may display a random order of the authors.
The correct author list is shown at the top of this webpage.
Similar Papers
A Graph-based Model for Joint Chinese Word Segmentation and Dependency Parsing
Hang Yan, Xipeng Qiu, Xuanjing Huang,
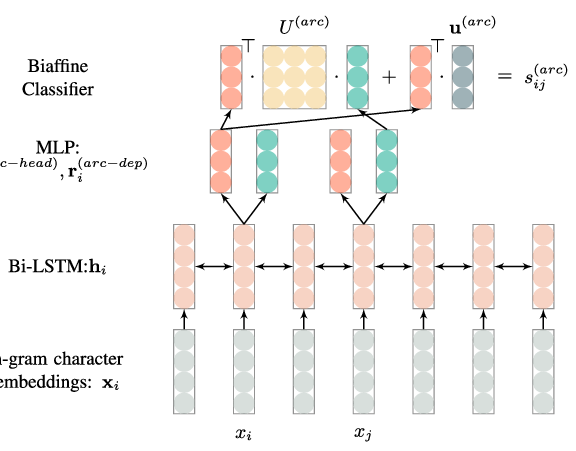
Simplify the Usage of Lexicon in Chinese NER
Ruotian Ma, Minlong Peng, Qi Zhang, Zhongyu Wei, Xuanjing Huang,
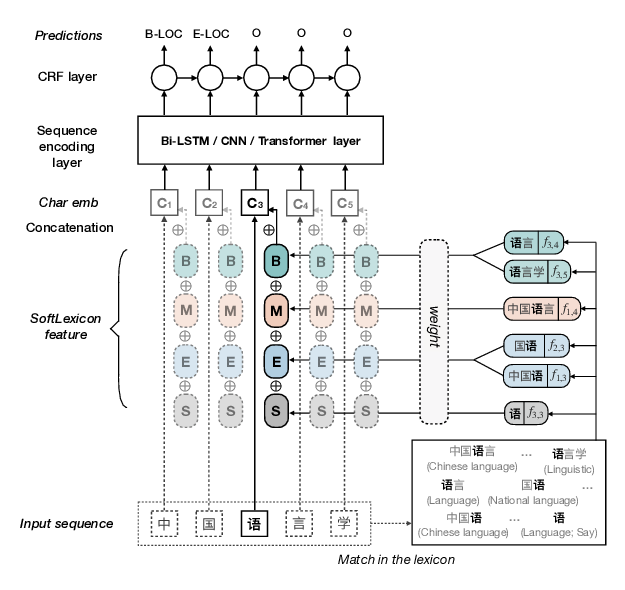
Roles and Utilization of Attention Heads in Transformer-based Neural Language Models
Jae-young Jo, Sung-Hyon Myaeng,
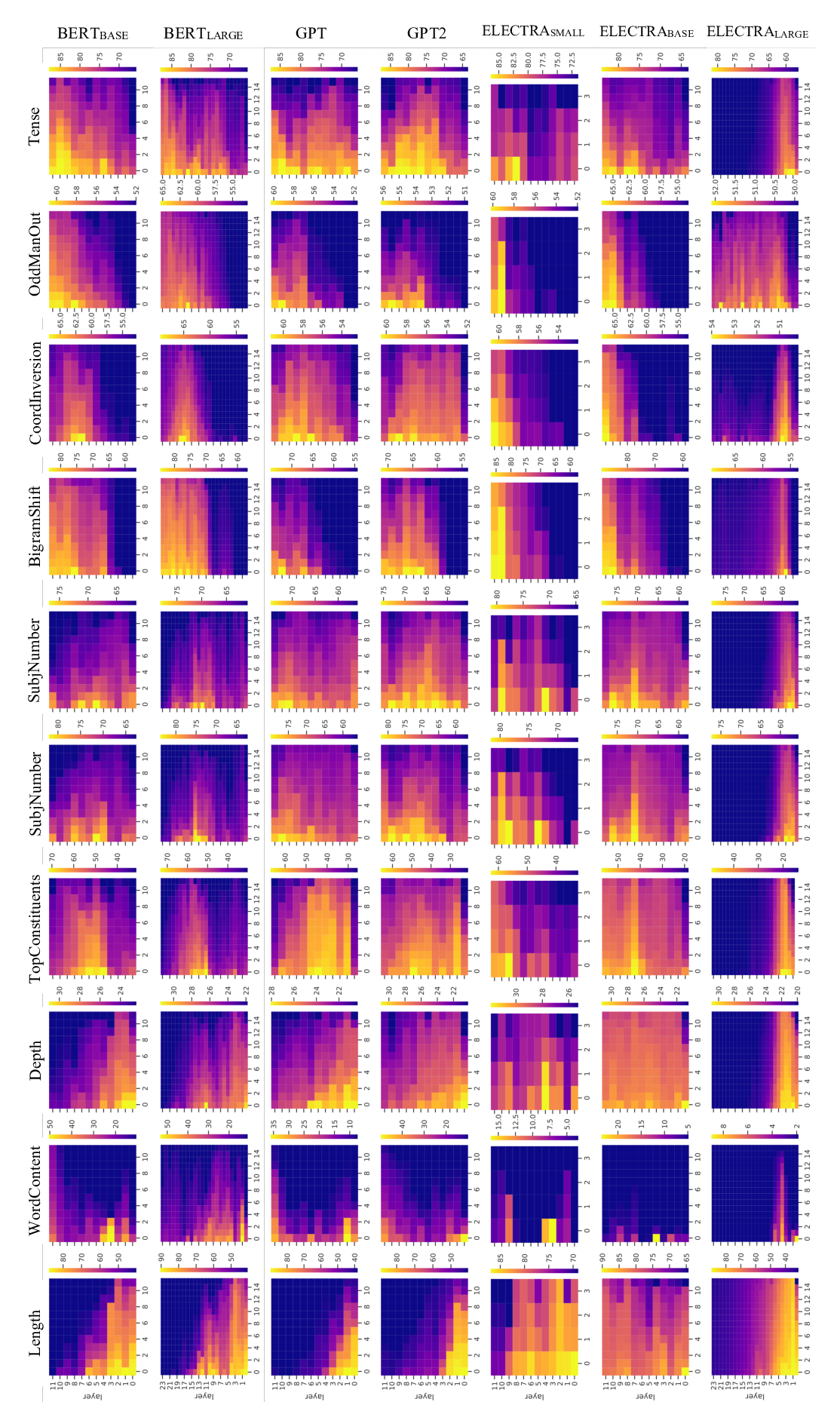