Transition-based Directed Graph Construction for Emotion-Cause Pair Extraction
Chuang Fan, Chaofa Yuan, Jiachen Du, Lin Gui, Min Yang, Ruifeng Xu
Sentiment Analysis, Stylistic Analysis, and Argument Mining Long Paper
Session 6B: Jul 7
(06:00-07:00 GMT)
Session 7B: Jul 7
(09:00-10:00 GMT)
Abstract:
Emotion-cause pair extraction aims to extract all potential pairs of emotions and corresponding causes from unannotated emotion text. Most existing methods are pipelined framework, which identifies emotions and extracts causes separately, leading to a drawback of error propagation. Towards this issue, we propose a transition-based model to transform the task into a procedure of parsing-like directed graph construction. The proposed model incrementally generates the directed graph with labeled edges based on a sequence of actions, from which we can recognize emotions with the corresponding causes simultaneously, thereby optimizing separate subtasks jointly and maximizing mutual benefits of tasks interdependently. Experimental results show that our approach achieves the best performance, outperforming the state-of-the-art methods by 6.71% (p<0.01) in F1 measure.
You can open the
pre-recorded video
in a separate window.
NOTE: The SlidesLive video may display a random order of the authors.
The correct author list is shown at the top of this webpage.
Similar Papers
ECPE-2D: Emotion-Cause Pair Extraction based on Joint Two-Dimensional Representation, Interaction and Prediction
Zixiang Ding, Rui Xia, Jianfei Yu,
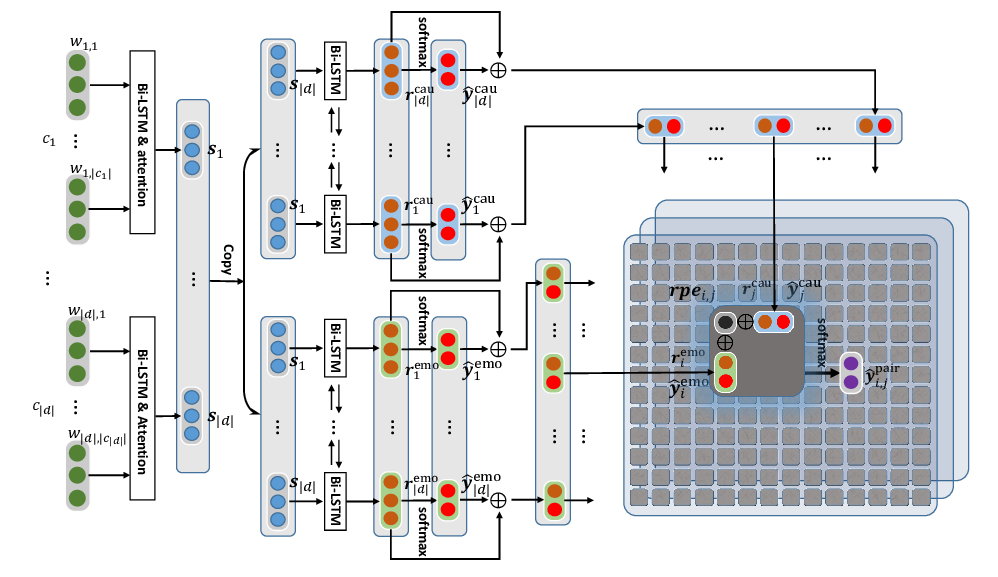
Effective Inter-Clause Modeling for End-to-End Emotion-Cause Pair Extraction
Penghui Wei, Jiahao Zhao, Wenji Mao,
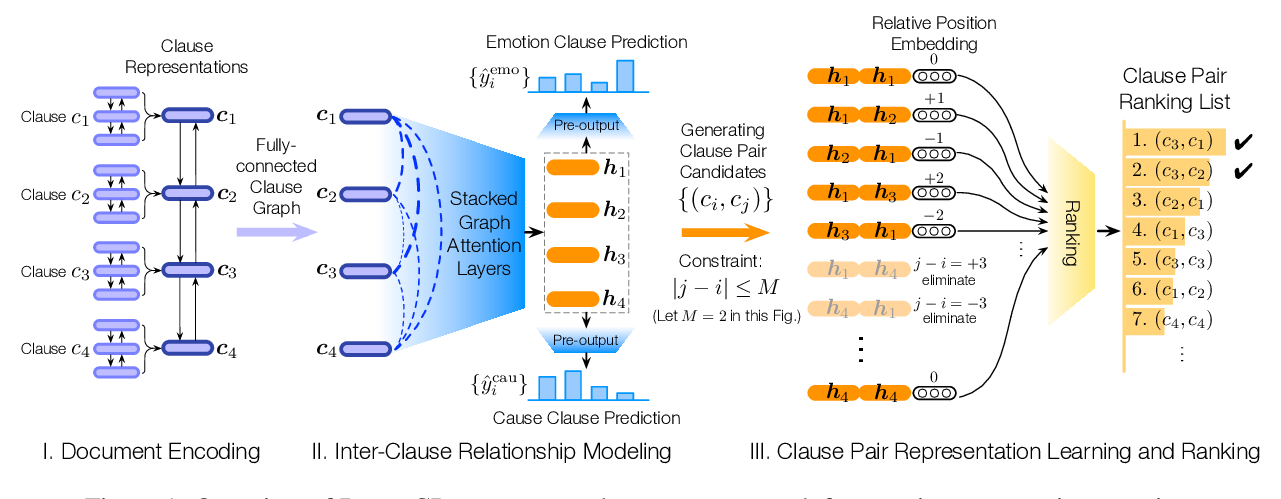
Line Graph Enhanced AMR-to-Text Generation with Mix-Order Graph Attention Networks
Yanbin Zhao, Lu Chen, Zhi Chen, Ruisheng Cao, Su Zhu, Kai Yu,
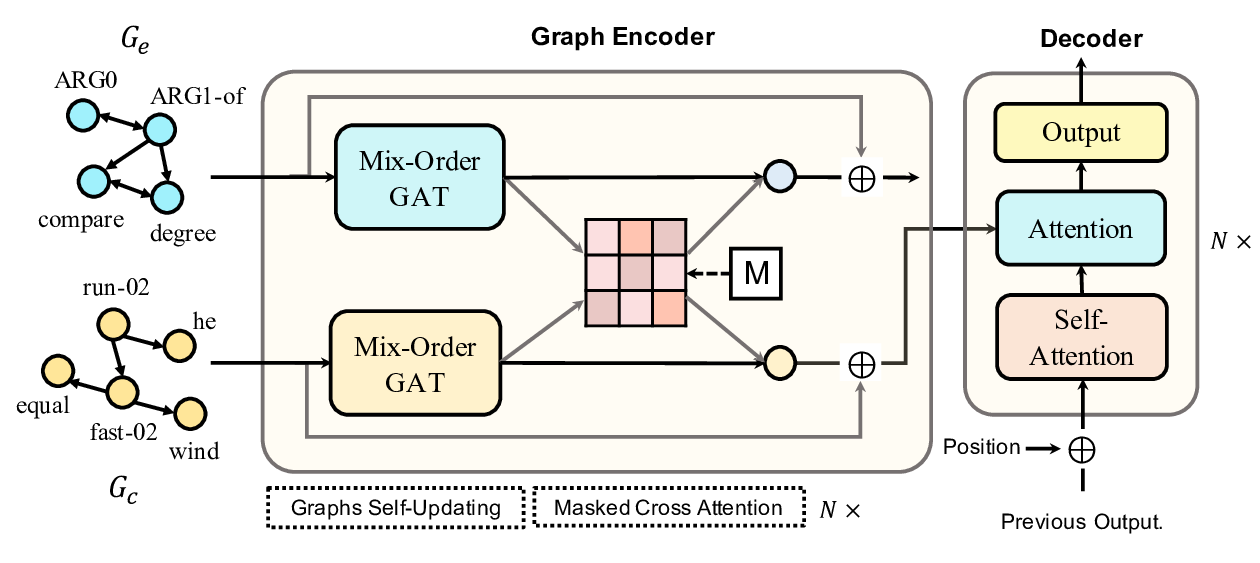