Effective Inter-Clause Modeling for End-to-End Emotion-Cause Pair Extraction
Penghui Wei, Jiahao Zhao, Wenji Mao
Sentiment Analysis, Stylistic Analysis, and Argument Mining Long Paper
Session 6A: Jul 7
(05:00-06:00 GMT)
Session 7A: Jul 7
(08:00-09:00 GMT)
Abstract:
Emotion-cause pair extraction aims to extract all emotion clauses coupled with their cause clauses from a given document. Previous work employs two-step approaches, in which the first step extracts emotion clauses and cause clauses separately, and the second step trains a classifier to filter out negative pairs. However, such pipeline-style system for emotion-cause pair extraction is suboptimal because it suffers from error propagation and the two steps may not adapt to each other well. In this paper, we tackle emotion-cause pair extraction from a ranking perspective, i.e., ranking clause pair candidates in a document, and propose a one-step neural approach which emphasizes inter-clause modeling to perform end-to-end extraction. It models the interrelations between the clauses in a document to learn clause representations with graph attention, and enhances clause pair representations with kernel-based relative position embedding for effective ranking. Experimental results show that our approach significantly outperforms the current two-step systems, especially in the condition of extracting multiple pairs in one document.
You can open the
pre-recorded video
in a separate window.
NOTE: The SlidesLive video may display a random order of the authors.
The correct author list is shown at the top of this webpage.
Similar Papers
ECPE-2D: Emotion-Cause Pair Extraction based on Joint Two-Dimensional Representation, Interaction and Prediction
Zixiang Ding, Rui Xia, Jianfei Yu,
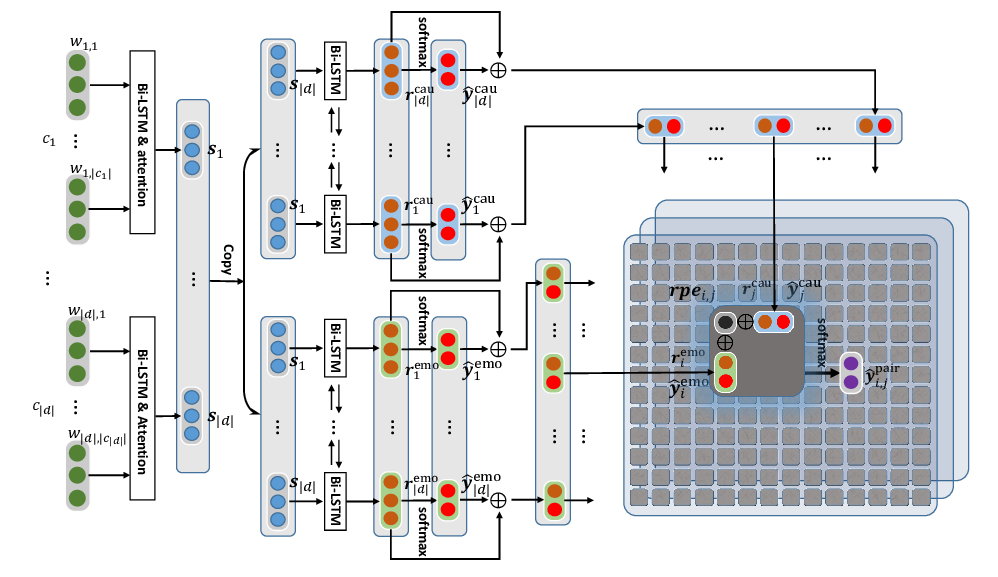
Transition-based Directed Graph Construction for Emotion-Cause Pair Extraction
Chuang Fan, Chaofa Yuan, Jiachen Du, Lin Gui, Min Yang, Ruifeng Xu,
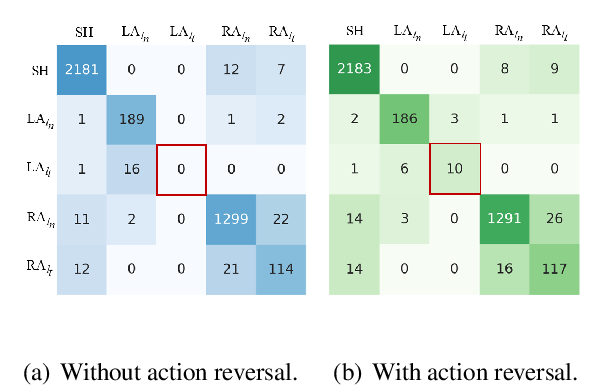
Representation Learning for Information Extraction from Form-like Documents
Bodhisattwa Prasad Majumder, Navneet Potti, Sandeep Tata, James Bradley Wendt, Qi Zhao, Marc Najork,
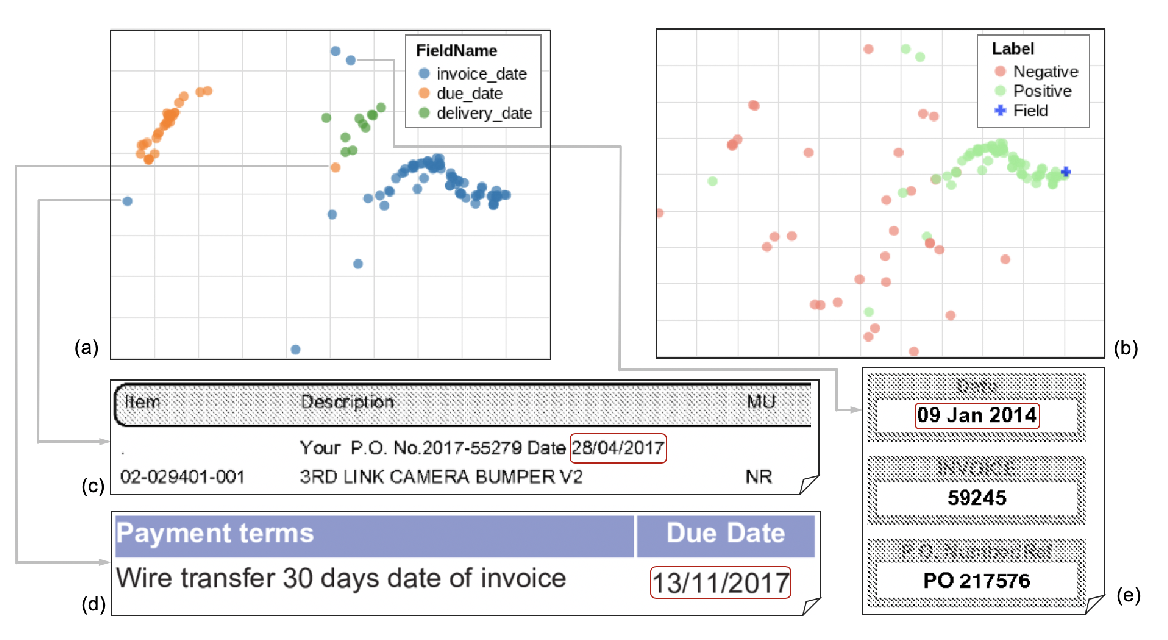
Response-Anticipated Memory for On-Demand Knowledge Integration in Response Generation
Zhiliang Tian, Wei Bi, Dongkyu Lee, Lanqing Xue, Yiping Song, Xiaojiang Liu, Nevin L. Zhang,
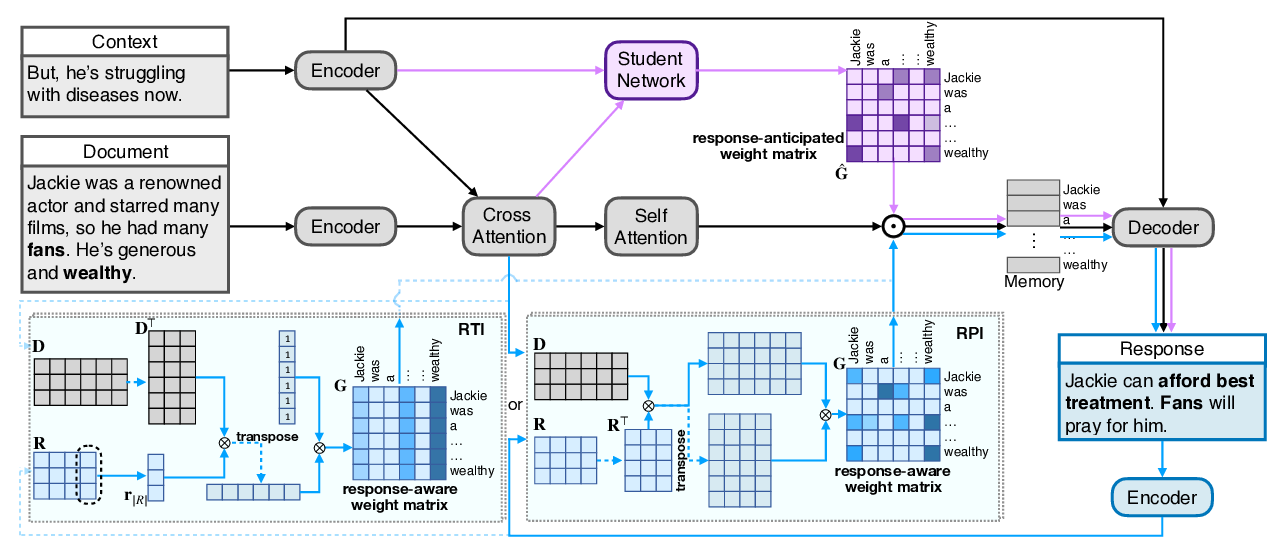