Learning Implicit Text Generation via Feature Matching
Inkit Padhi, Pierre Dognin, Ke Bai, Cícero Nogueira dos Santos, Vijil Chenthamarakshan, Youssef Mroueh, Payel Das
Generation Short Paper
Session 7A: Jul 7
(08:00-09:00 GMT)
Session 8B: Jul 7
(13:00-14:00 GMT)
Abstract:
Generative feature matching network (GFMN) is an approach for training state-of-the-art implicit generative models for images by performing moment matching on features from pre-trained neural networks. In this paper, we present new GFMN formulations that are effective for sequential data. Our experimental results show the effectiveness of the proposed method, SeqGFMN, for three distinct generation tasks in English: unconditional text generation, class-conditional text generation, and unsupervised text style transfer. SeqGFMN is stable to train and outperforms various adversarial approaches for text generation and text style transfer.
You can open the
pre-recorded video
in a separate window.
NOTE: The SlidesLive video may display a random order of the authors.
The correct author list is shown at the top of this webpage.
Similar Papers
Exploring Contextual Word-level Style Relevance for Unsupervised Style Transfer
Chulun Zhou, Liangyu Chen, Jiachen Liu, Xinyan Xiao, Jinsong Su, Sheng Guo, Hua Wu,
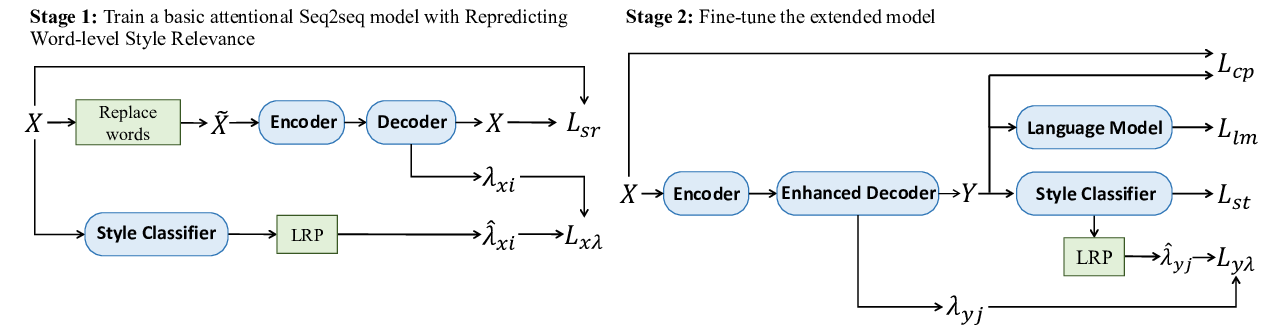
Politeness Transfer: A Tag and Generate Approach
Aman Madaan, Amrith Setlur, Tanmay Parekh, Barnabas Poczos, Graham Neubig, Yiming Yang, Ruslan Salakhutdinov, Alan W Black, Shrimai Prabhumoye,

Improving Disentangled Text Representation Learning with Information-Theoretic Guidance
Pengyu Cheng, Martin Renqiang Min, Dinghan Shen, Christopher Malon, Yizhe Zhang, Yitong Li, Lawrence Carin,
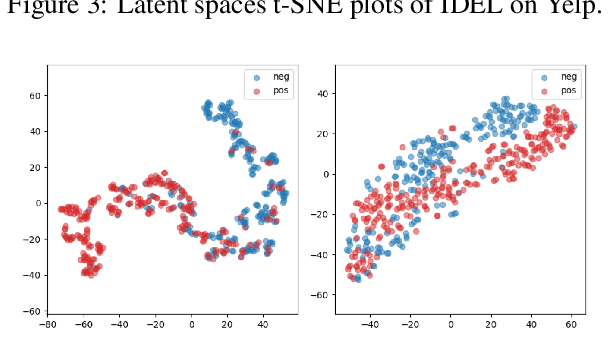
Distilling Knowledge Learned in BERT for Text Generation
Yen-Chun Chen, Zhe Gan, Yu Cheng, Jingzhou Liu, Jingjing Liu,
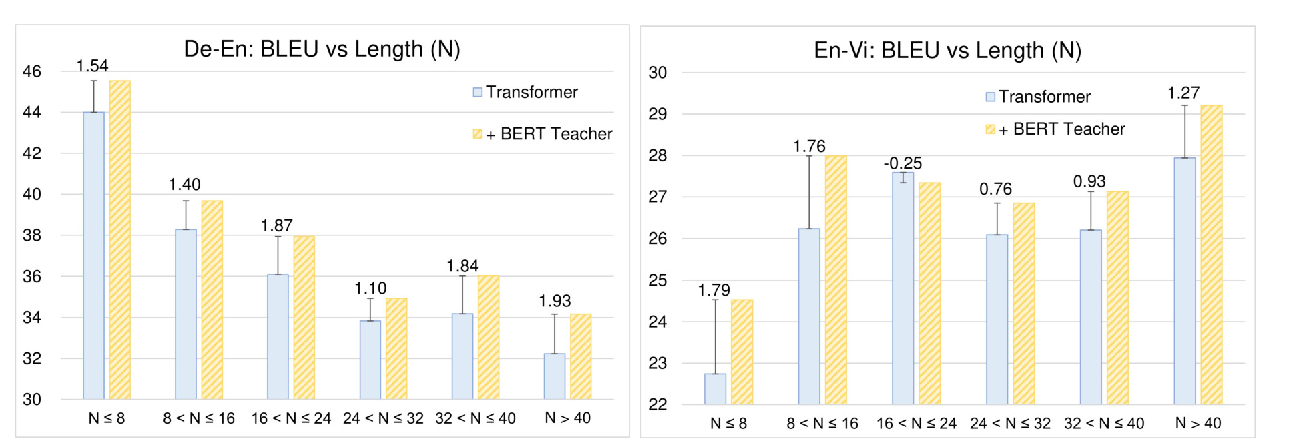