A Self-Training Method for Machine Reading Comprehension with Soft Evidence Extraction
Yilin Niu, Fangkai Jiao, Mantong Zhou, Ting Yao, Jingfang Xu, Minlie Huang
Question Answering Long Paper
Session 7A: Jul 7
(08:00-09:00 GMT)
Session 8A: Jul 7
(12:00-13:00 GMT)
Abstract:
Neural models have achieved great success on machine reading comprehension (MRC), many of which typically consist of two components: an evidence extractor and an answer predictor. The former seeks the most relevant information from a reference text, while the latter is to locate or generate answers from the extracted evidence. Despite the importance of evidence labels for training the evidence extractor, they are not cheaply accessible, particularly in many non-extractive MRC tasks such as YES/NO question answering and multi-choice MRC. To address this problem, we present a Self-Training method (STM), which supervises the evidence extractor with auto-generated evidence labels in an iterative process. At each iteration, a base MRC model is trained with golden answers and noisy evidence labels. The trained model will predict pseudo evidence labels as extra supervision in the next iteration. We evaluate STM on seven datasets over three MRC tasks. Experimental results demonstrate the improvement on existing MRC models, and we also analyze how and why such a self-training method works in MRC.
You can open the
pre-recorded video
in a separate window.
NOTE: The SlidesLive video may display a random order of the authors.
The correct author list is shown at the top of this webpage.
Similar Papers
Interactive Machine Comprehension with Information Seeking Agents
Xingdi Yuan, Jie Fu, Marc-Alexandre Côté, Yi Tay, Chris Pal, Adam Trischler,
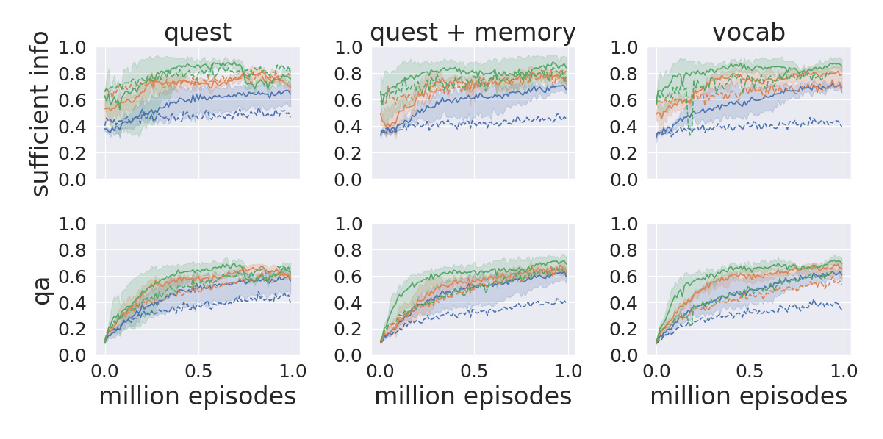
Enhancing Answer Boundary Detection for Multilingual Machine Reading Comprehension
Fei Yuan, Linjun Shou, Xuanyu Bai, Ming Gong, Yaobo Liang, Nan Duan, Yan Fu, Daxin Jiang,
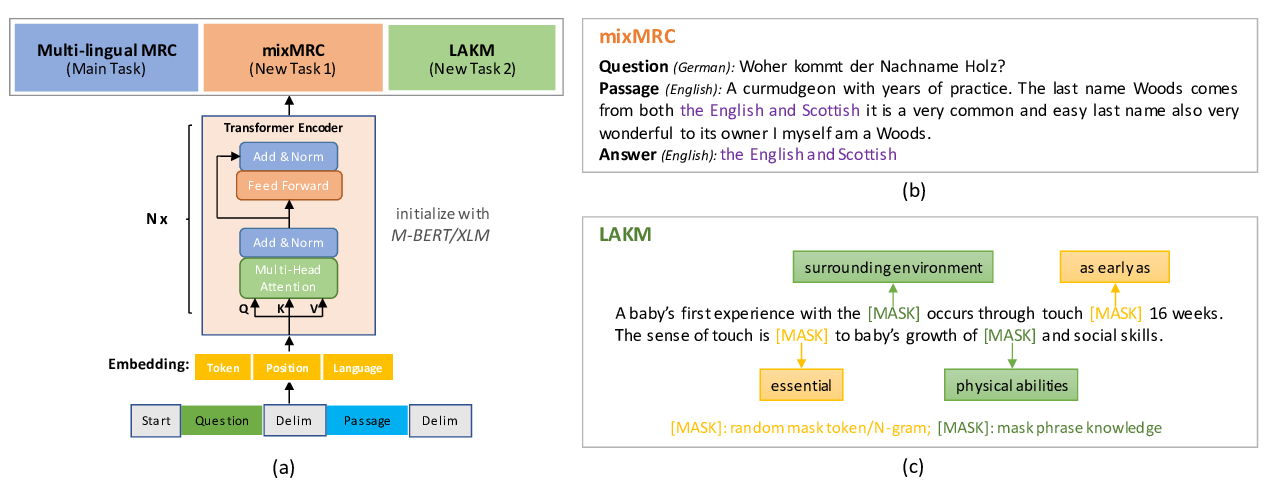
DTCA: Decision Tree-based Co-Attention Networks for Explainable Claim Verification
Lianwei Wu, Yuan Rao, Yongqiang Zhao, Hao Liang, Ambreen Nazir,
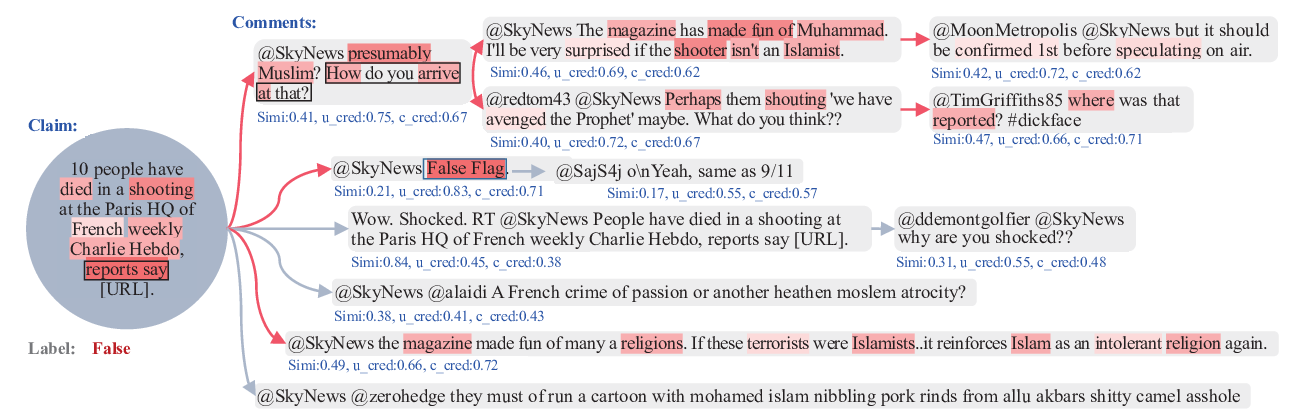
Unsupervised Alignment-based Iterative Evidence Retrieval for Multi-hop Question Answering
Vikas Yadav, Steven Bethard, Mihai Surdeanu,
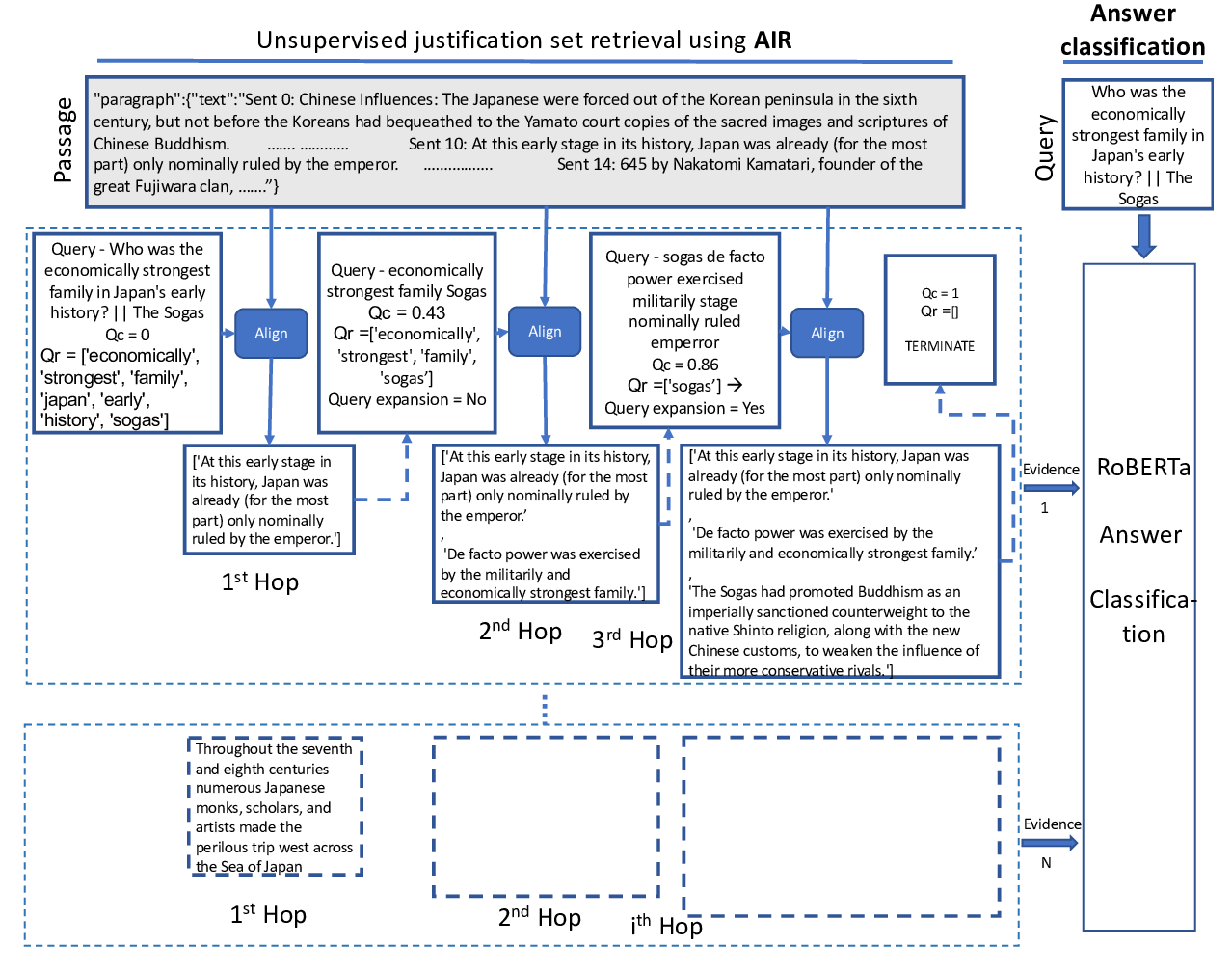