DTCA: Decision Tree-based Co-Attention Networks for Explainable Claim Verification
Lianwei Wu, Yuan Rao, Yongqiang Zhao, Hao Liang, Ambreen Nazir
Computational Social Science and Social Media Long Paper
Session 2A: Jul 6
(08:00-09:00 GMT)
Session 3A: Jul 6
(12:00-13:00 GMT)
Abstract:
Recently, many methods discover effective evidence from reliable sources by appropriate neural networks for explainable claim verification, which has been widely recognized. However, in these methods, the discovery process of evidence is nontransparent and unexplained. Simultaneously, the discovered evidence is aimed at the interpretability of the whole sequence of claims but insufficient to focus on the false parts of claims. In this paper, we propose a Decision Tree-based Co-Attention model (DTCA) to discover evidence for explainable claim verification. Specifically, we first construct Decision Tree-based Evidence model (DTE) to select comments with high credibility as evidence in a transparent and interpretable way. Then we design Co-attention Self-attention networks (CaSa) to make the selected evidence interact with claims, which is for 1) training DTE to determine the optimal decision thresholds and obtain more powerful evidence; and 2) utilizing the evidence to find the false parts in the claim. Experiments on two public datasets, RumourEval and PHEME, demonstrate that DTCA not only provides explanations for the results of claim verification but also achieves the state-of-the-art performance, boosting the F1-score by more than 3.11%, 2.41%, respectively.
You can open the
pre-recorded video
in a separate window.
NOTE: The SlidesLive video may display a random order of the authors.
The correct author list is shown at the top of this webpage.
Similar Papers
Reasoning Over Semantic-Level Graph for Fact Checking
Wanjun Zhong, Jingjing Xu, Duyu Tang, Zenan Xu, Nan Duan, Ming Zhou, Jiahai Wang, Jian Yin,
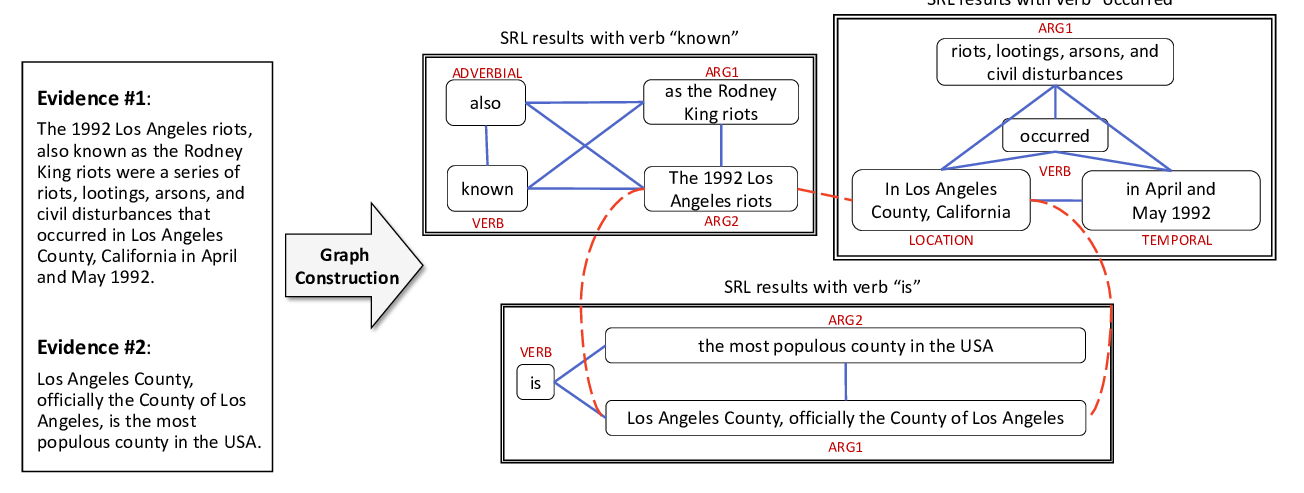
Fine-grained Fact Verification with Kernel Graph Attention Network
Zhenghao Liu, Chenyan Xiong, Maosong Sun, Zhiyuan Liu,
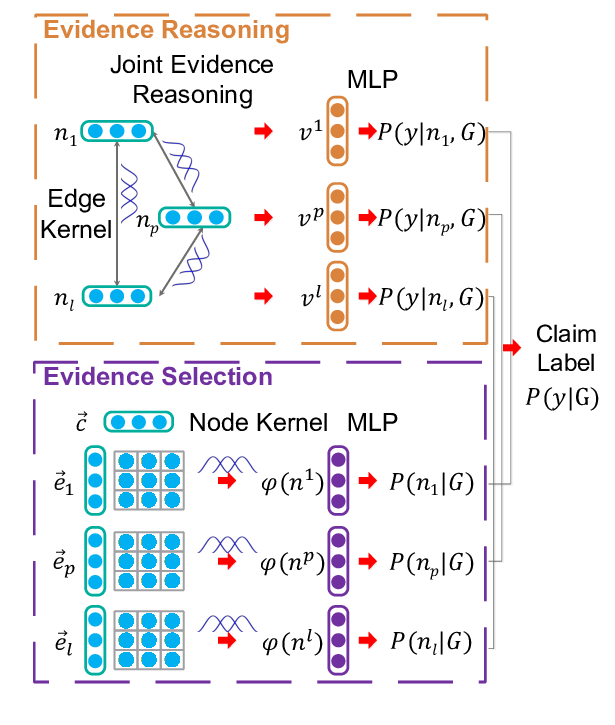
DeSePtion: Dual Sequence Prediction and Adversarial Examples for Improved Fact-Checking
Christopher Hidey, Tuhin Chakrabarty, Tariq Alhindi, Siddharth Varia, Kriste Krstovski, Mona Diab, Smaranda Muresan,
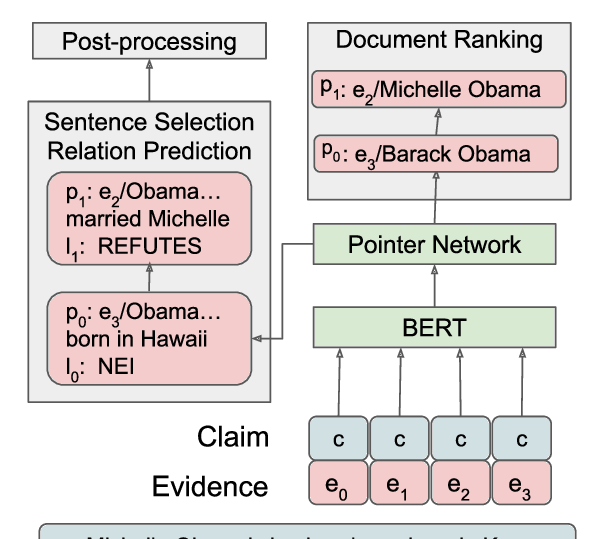
A Self-Training Method for Machine Reading Comprehension with Soft Evidence Extraction
Yilin Niu, Fangkai Jiao, Mantong Zhou, Ting Yao, Jingfang Xu, Minlie Huang,
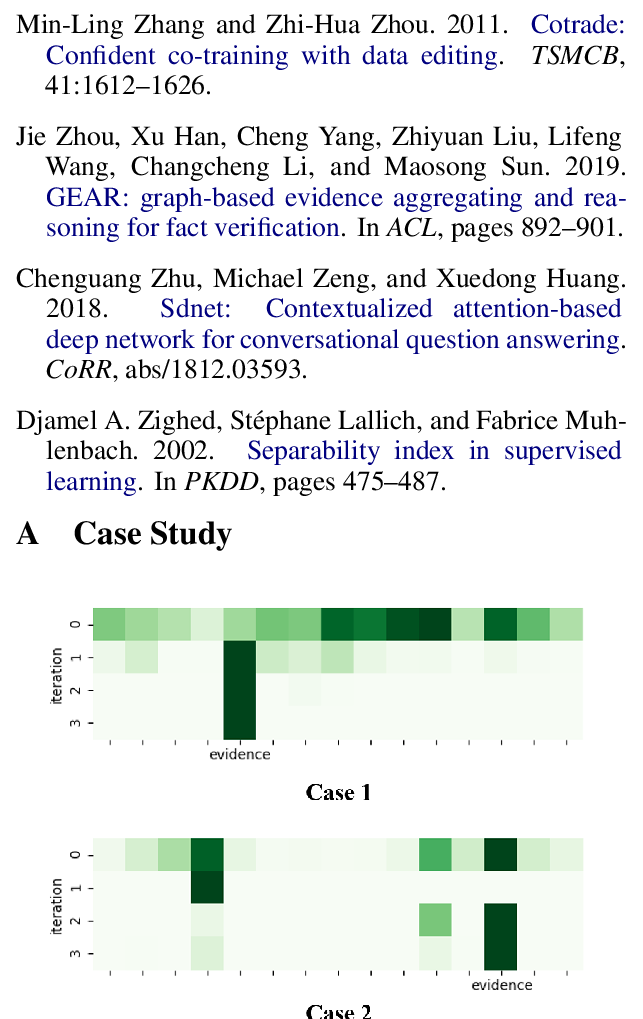