Encoder-Decoder Models Can Benefit from Pre-trained Masked Language Models in Grammatical Error Correction
Masahiro Kaneko, Masato Mita, Shun Kiyono, Jun Suzuki, Kentaro Inui
NLP Applications Short Paper
Session 7B: Jul 7
(09:00-10:00 GMT)
Session 8B: Jul 7
(13:00-14:00 GMT)
Abstract:
This paper investigates how to effectively incorporate a pre-trained masked language model (MLM), such as BERT, into an encoder-decoder (EncDec) model for grammatical error correction (GEC). The answer to this question is not as straightforward as one might expect because the previous common methods for incorporating a MLM into an EncDec model have potential drawbacks when applied to GEC. For example, the distribution of the inputs to a GEC model can be considerably different (erroneous, clumsy, etc.) from that of the corpora used for pre-training MLMs; however, this issue is not addressed in the previous methods. Our experiments show that our proposed method, where we first fine-tune a MLM with a given GEC corpus and then use the output of the fine-tuned MLM as additional features in the GEC model, maximizes the benefit of the MLM. The best-performing model achieves state-of-the-art performances on the BEA-2019 and CoNLL-2014 benchmarks. Our code is publicly available at: https://github.com/kanekomasahiro/bert-gec.
You can open the
pre-recorded video
in a separate window.
NOTE: The SlidesLive video may display a random order of the authors.
The correct author list is shown at the top of this webpage.
Similar Papers
A Graph-based Model for Joint Chinese Word Segmentation and Dependency Parsing
Hang Yan, Xipeng Qiu, Xuanjing Huang,
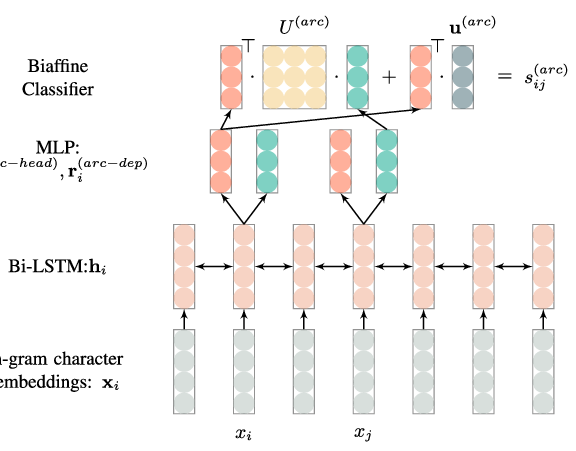
A Two-Stage Masked LM Method for Term Set Expansion
Guy Kushilevitz, Shaul Markovitch, Yoav Goldberg,
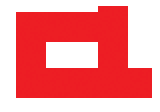