Text-Based Ideal Points
Keyon Vafa, Suresh Naidu, David Blei
Computational Social Science and Social Media Long Paper
Session 9B: Jul 7
(18:00-19:00 GMT)
Session 10A: Jul 7
(20:00-21:00 GMT)
Abstract:
Ideal point models analyze lawmakers' votes to quantify their political positions, or ideal points. But votes are not the only way to express a political position. Lawmakers also give speeches, release press statements, and post tweets. In this paper, we introduce the text-based ideal point model (TBIP), an unsupervised probabilistic topic model that analyzes texts to quantify the political positions of its authors. We demonstrate the TBIP with two types of politicized text data: U.S. Senate speeches and senator tweets. Though the model does not analyze their votes or political affiliations, the TBIP separates lawmakers by party, learns interpretable politicized topics, and infers ideal points close to the classical vote-based ideal points. One benefit of analyzing texts, as opposed to votes, is that the TBIP can estimate ideal points of anyone who authors political texts, including non-voting actors. To this end, we use it to study tweets from the 2020 Democratic presidential candidates. Using only the texts of their tweets, it identifies them along an interpretable progressive-to-moderate spectrum.
You can open the
pre-recorded video
in a separate window.
NOTE: The SlidesLive video may display a random order of the authors.
The correct author list is shown at the top of this webpage.
Similar Papers
Analyzing Political Parody in Social Media
Antonios Maronikolakis, Danae Sánchez Villegas, Daniel Preotiuc-Pietro, Nikolaos Aletras,
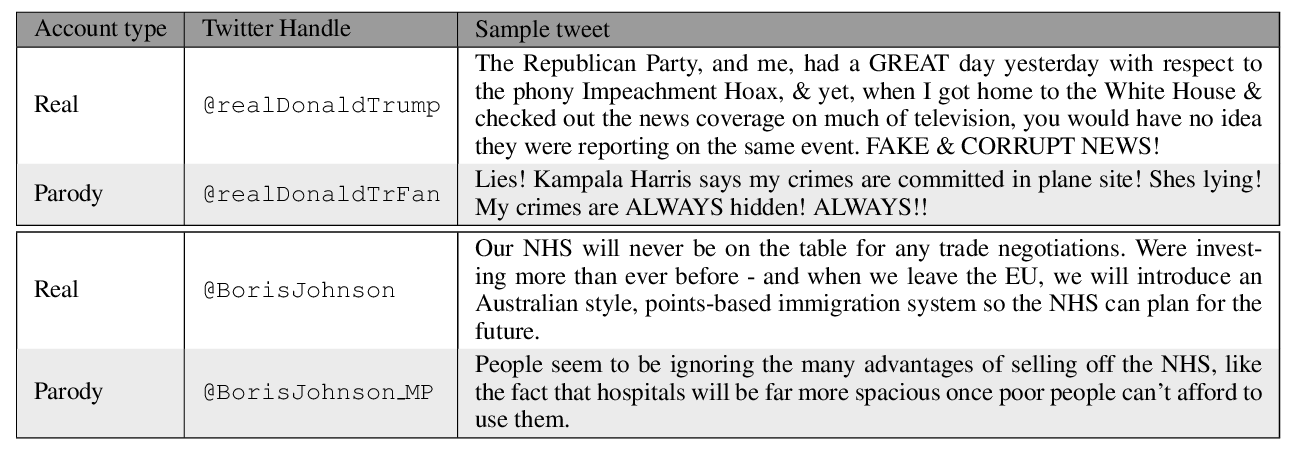
Neural Temporal Opinion Modelling for Opinion Prediction on Twitter
Lixing Zhu, Yulan He, Deyu Zhou,
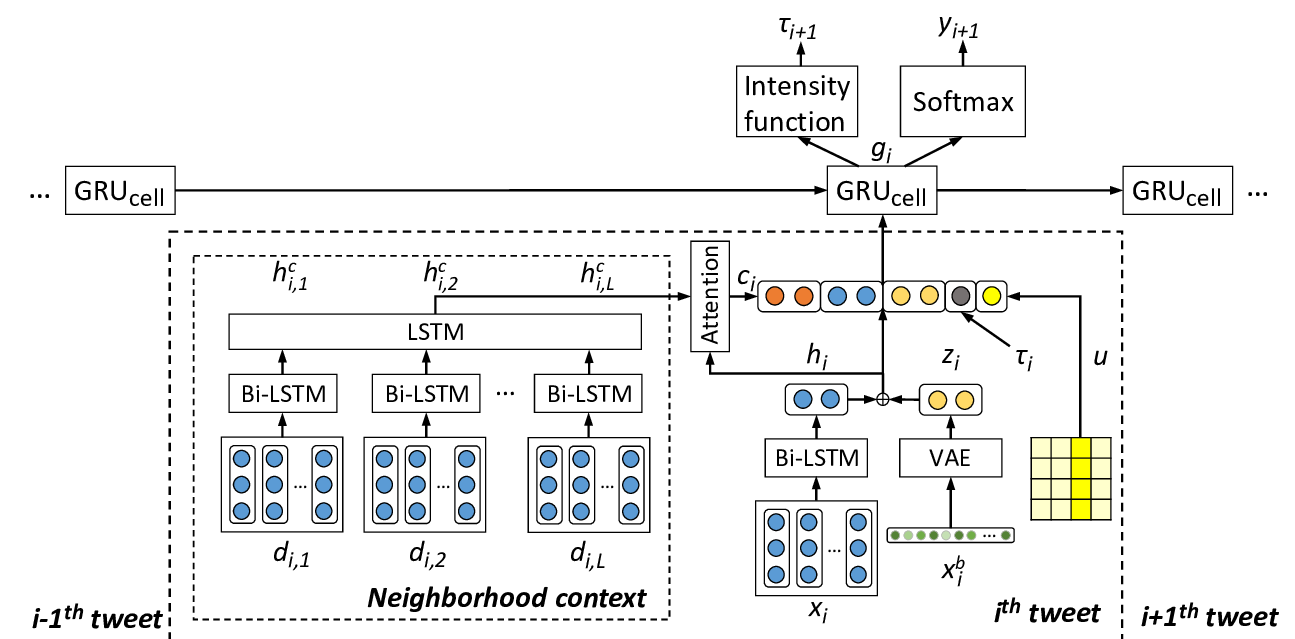
Masking Actor Information Leads to Fairer Political Claims Detection
Erenay Dayanik, Sebastian Padó,
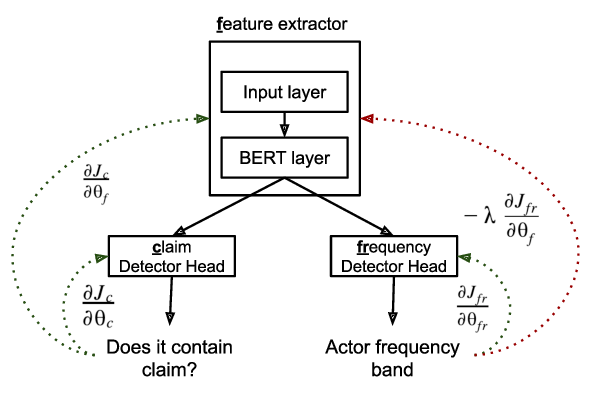
Prta: A System to Support the Analysis of Propaganda Techniques in the News
Giovanni Da San Martino, Shaden Shaar, Yifan Zhang, Seunghak Yu, Alberto Barrón-Cedeño, Preslav Nakov,
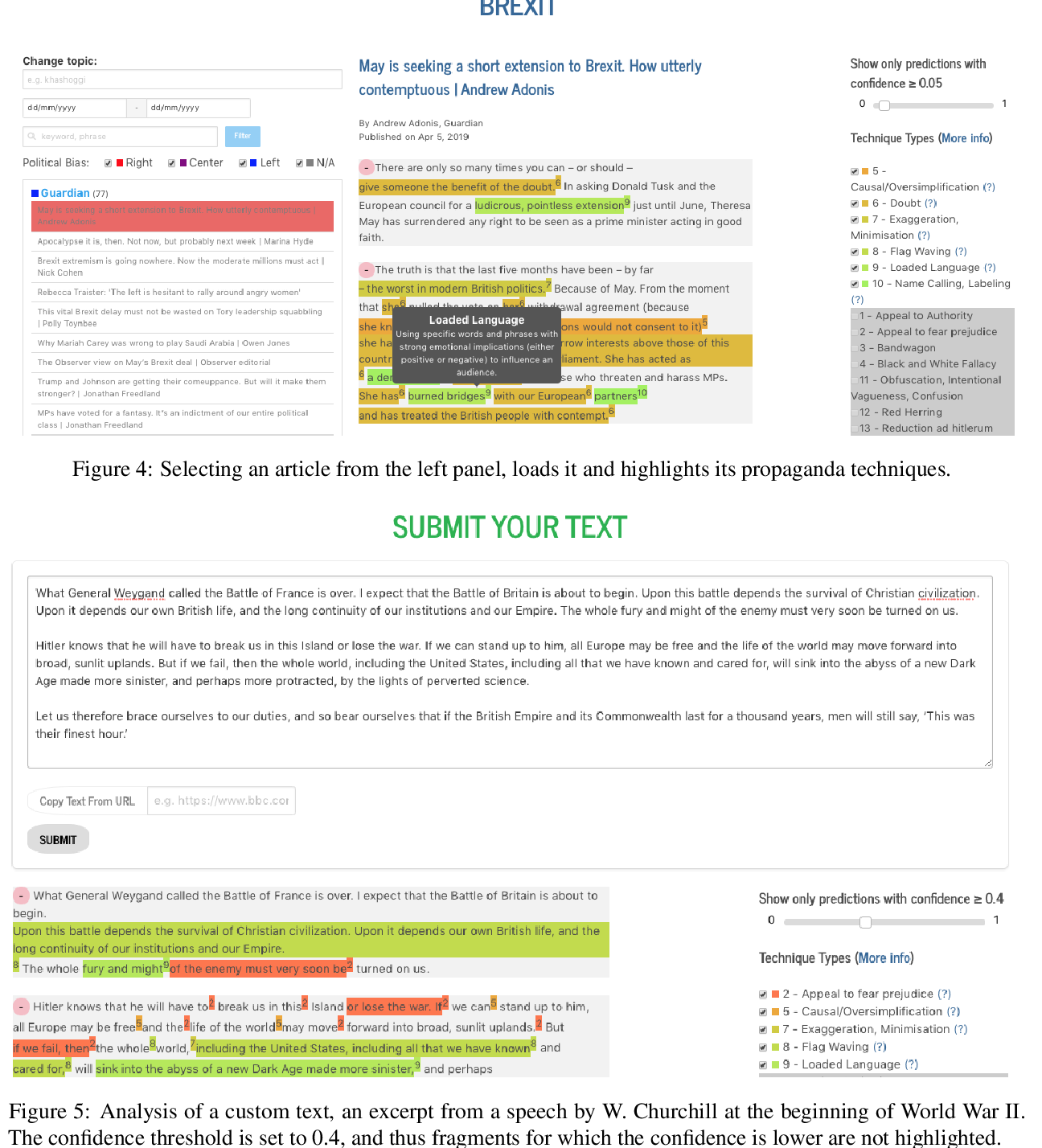