Masking Actor Information Leads to Fairer Political Claims Detection
Erenay Dayanik, Sebastian Padó
Computational Social Science and Social Media Short Paper
Session 8A: Jul 7
(12:00-13:00 GMT)
Session 10A: Jul 7
(20:00-21:00 GMT)
Abstract:
A central concern in Computational Social Sciences (CSS) is fairness: where the role of NLP is to scale up text analysis to large corpora, the quality of automatic analyses should be as independent as possible of textual properties. We analyze the performance of a state-of-the-art neural model on the task of political claims detection (i.e., the identification of forward-looking statements made by political actors) and identify a strong frequency bias: claims made by frequent actors are recognized better. We propose two simple debiasing methods which mask proper names and pronouns during training of the model, thus removing personal information bias. We find that (a) these methods significantly decrease frequency bias while keeping the overall performance stable; and (b) the resulting models improve when evaluated in an out-of-domain setting.
You can open the
pre-recorded video
in a separate window.
NOTE: The SlidesLive video may display a random order of the authors.
The correct author list is shown at the top of this webpage.
Similar Papers
On Exposure Bias, Hallucination and Domain Shift in Neural Machine Translation
Chaojun Wang, Rico Sennrich,
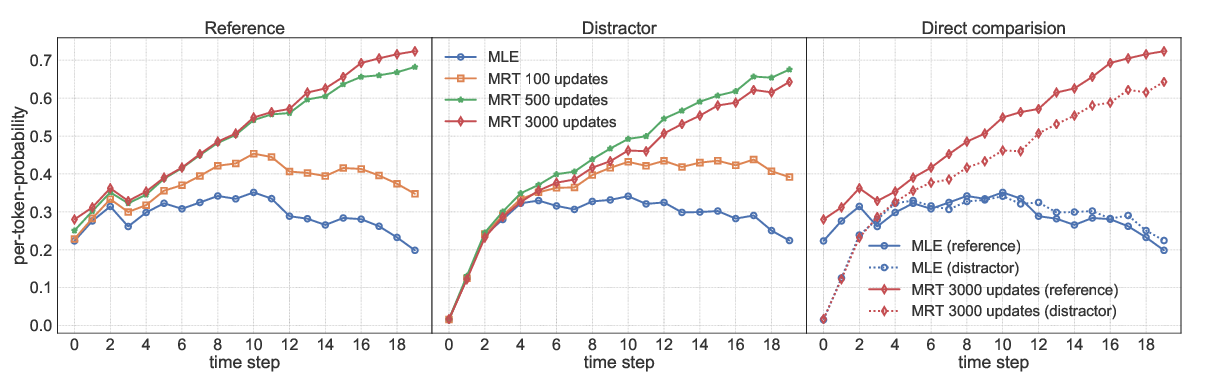
End-to-End Bias Mitigation by Modelling Biases in Corpora
Rabeeh Karimi Mahabadi, Yonatan Belinkov, James Henderson,
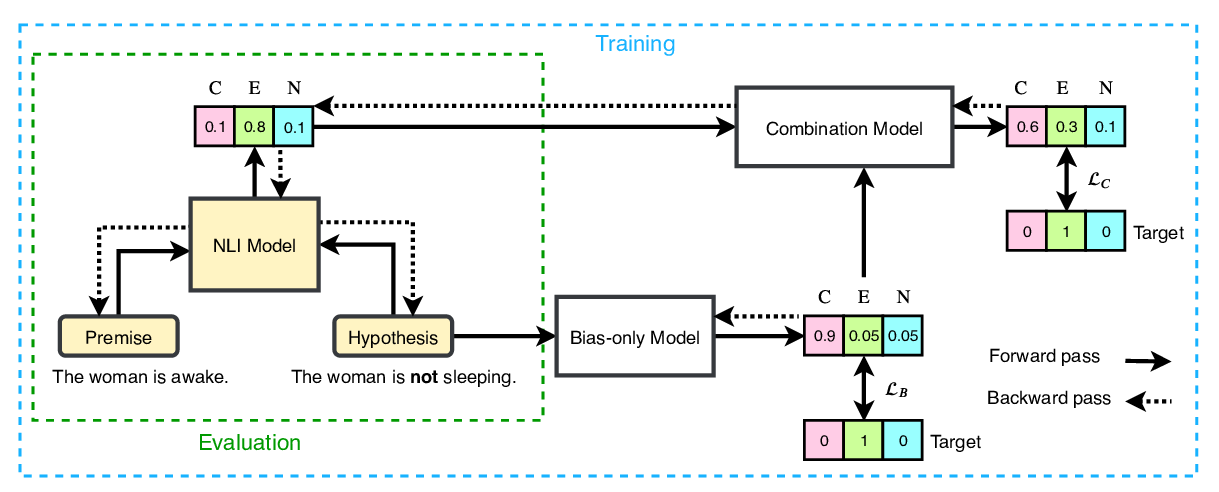
Predictive Biases in Natural Language Processing Models: A Conceptual Framework and Overview
Deven Santosh Shah, H. Andrew Schwartz, Dirk Hovy,
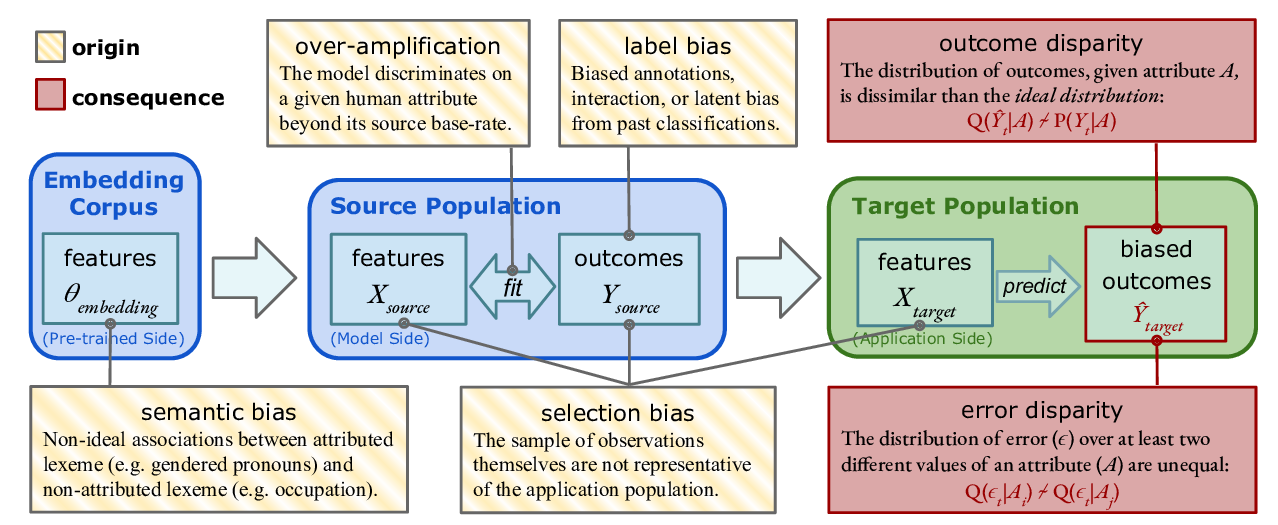