Social Biases in NLP Models as Barriers for Persons with Disabilities
Ben Hutchinson, Vinodkumar Prabhakaran, Emily Denton, Kellie Webster, Yu Zhong, Stephen Denuyl
Ethics and NLP Short Paper
Session 9B: Jul 7
(18:00-19:00 GMT)
Session 10B: Jul 7
(21:00-22:00 GMT)
Abstract:
Building equitable and inclusive NLP technologies demands consideration of whether and how social attitudes are represented in ML models. In particular, representations encoded in models often inadvertently perpetuate undesirable social biases from the data on which they are trained. In this paper, we present evidence of such undesirable biases towards mentions of disability in two different English language models: toxicity prediction and sentiment analysis. Next, we demonstrate that the neural embeddings that are the critical first step in most NLP pipelines similarly contain undesirable biases towards mentions of disability. We end by highlighting topical biases in the discourse about disability which may contribute to the observed model biases; for instance, gun violence, homelessness, and drug addiction are over-represented in texts discussing mental illness.
You can open the
pre-recorded video
in a separate window.
NOTE: The SlidesLive video may display a random order of the authors.
The correct author list is shown at the top of this webpage.
Similar Papers
When do Word Embeddings Accurately Reflect Surveys on our Beliefs About People?
Kenneth Joseph, Jonathan Morgan,
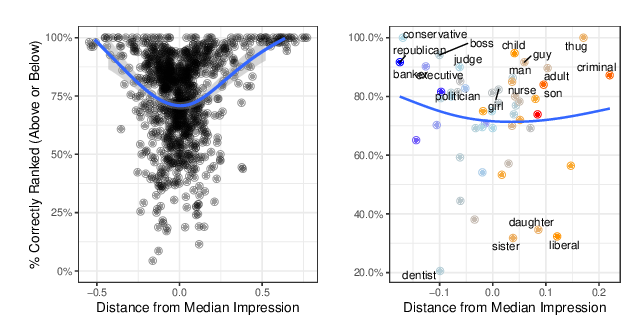
Towards Debiasing Sentence Representations
Paul Pu Liang, Irene Mengze Li, Emily Zheng, Yao Chong Lim, Ruslan Salakhutdinov, Louis-Philippe Morency,
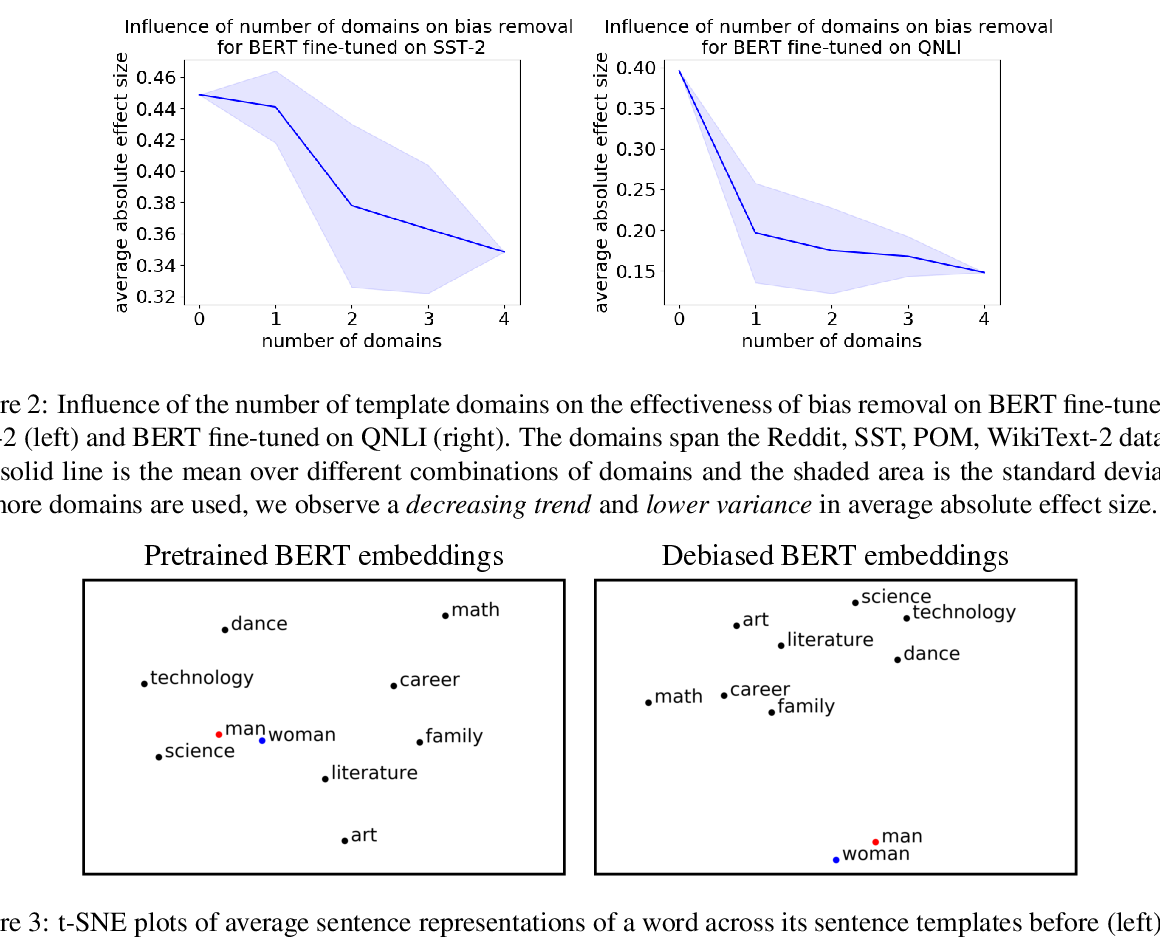
Social Bias Frames: Reasoning about Social and Power Implications of Language
Maarten Sap, Saadia Gabriel, Lianhui Qin, Dan Jurafsky, Noah A. Smith, Yejin Choi,
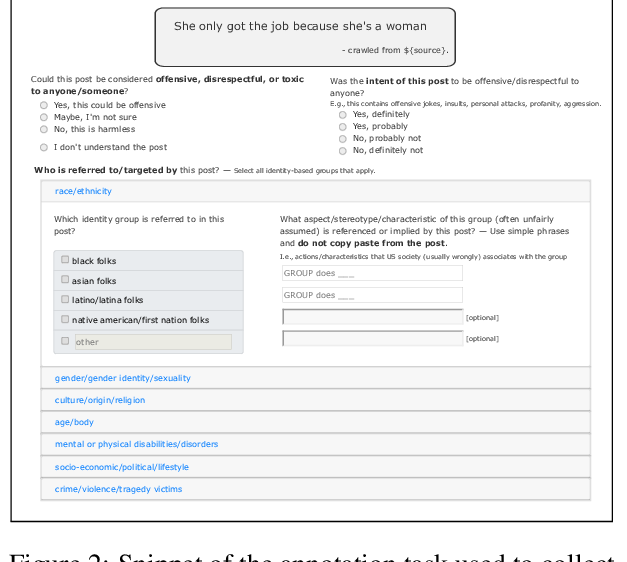