Integrating Semantic and Structural Information with Graph Convolutional Network for Controversy Detection
Lei Zhong, Juan Cao, Qiang Sheng, Junbo Guo, Ziang Wang
Computational Social Science and Social Media Long Paper
Session 1B: Jul 6
(06:00-07:00 GMT)
Session 2A: Jul 6
(08:00-09:00 GMT)
Abstract:
Identifying controversial posts on social media is a fundamental task for mining public sentiment, assessing the influence of events, and alleviating the polarized views. However, existing methods fail to 1) effectively incorporate the semantic information from content-related posts; 2) preserve the structural information for reply relationship modeling; 3) properly handle posts from topics dissimilar to those in the training set. To overcome the first two limitations, we propose Topic-Post-Comment Graph Convolutional Network (TPC-GCN), which integrates the information from the graph structure and content of topics, posts, and comments for post-level controversy detection. As to the third limitation, we extend our model to Disentangled TPC-GCN (DTPC-GCN), to disentangle topic-related and topic-unrelated features and then fuse dynamically. Extensive experiments on two real-world datasets demonstrate that our models outperform existing methods. Analysis of the results and cases proves that our models can integrate both semantic and structural information with significant generalizability.
You can open the
pre-recorded video
in a separate window.
NOTE: The SlidesLive video may display a random order of the authors.
The correct author list is shown at the top of this webpage.
Similar Papers
Neural Topic Modeling with Bidirectional Adversarial Training
Rui Wang, Xuemeng Hu, Deyu Zhou, Yulan He, Yuxuan Xiong, Chenchen Ye, Haiyang Xu,
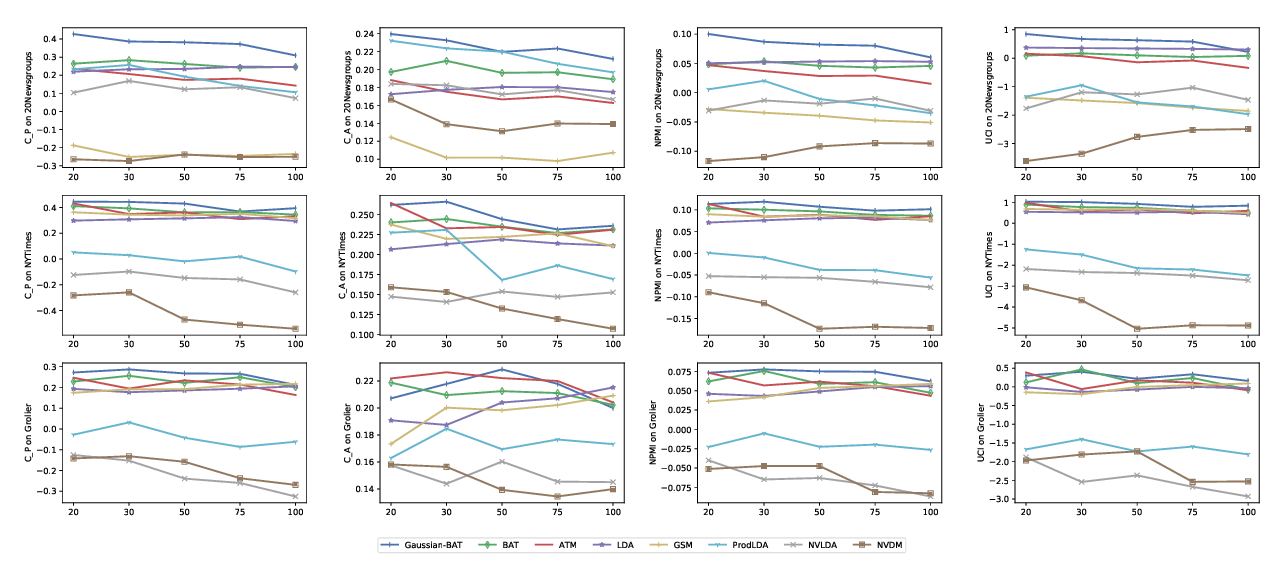
Topic Balancing with Additive Regularization of Topic Models
Eugeniia Veselova, Konstantin Vorontsov,
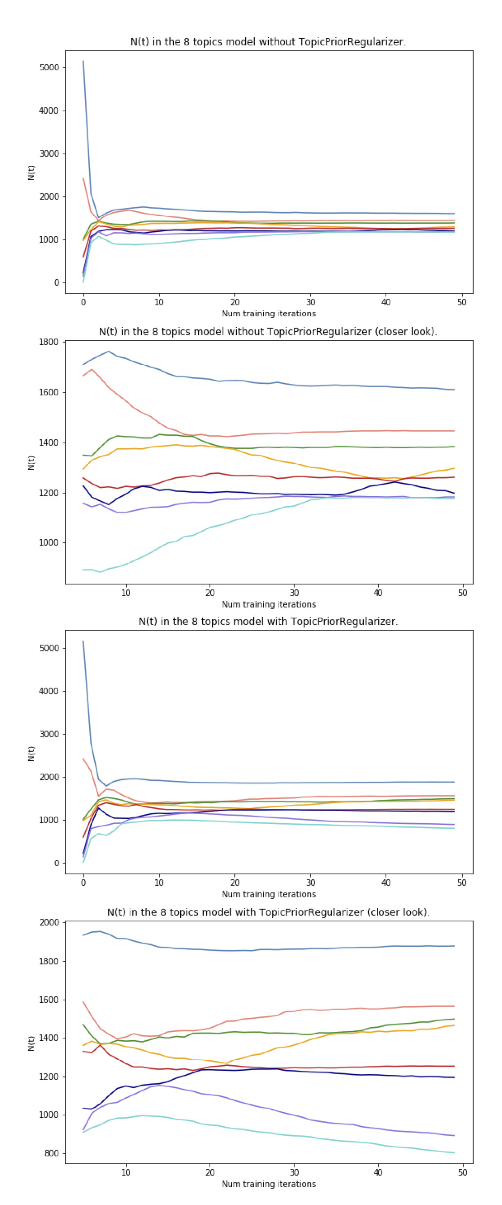
Aspect Sentiment Classification with Document-level Sentiment Preference Modeling
Xiao Chen, Changlong Sun, Jingjing Wang, Shoushan Li, Luo Si, Min Zhang, Guodong Zhou,
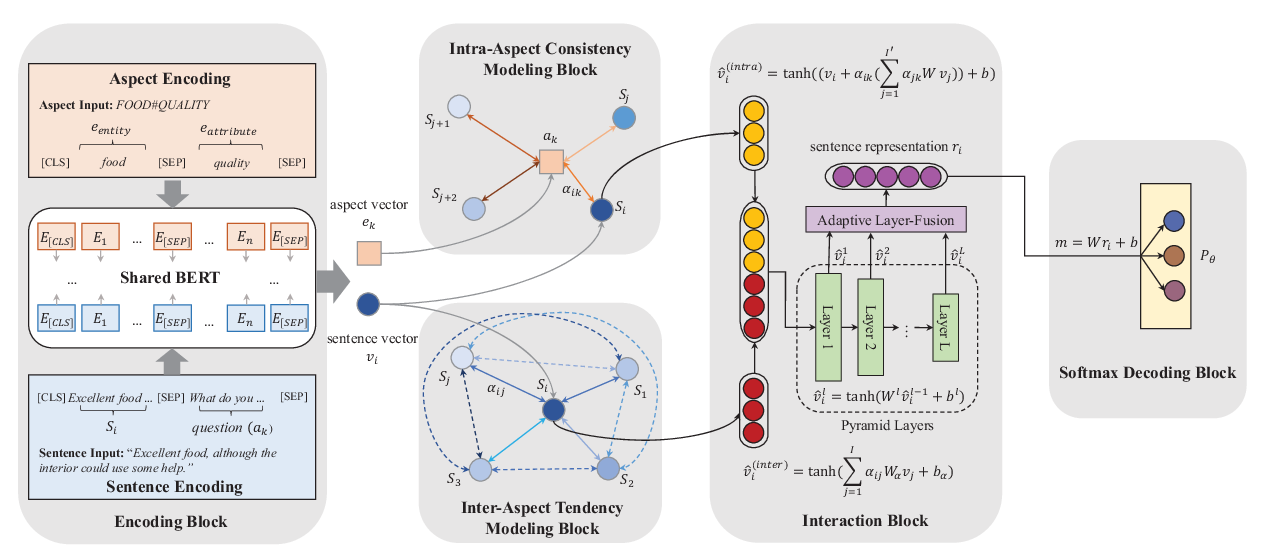
SEEK: Segmented Embedding of Knowledge Graphs
Wentao Xu, Shun Zheng, Liang He, Bin Shao, Jian Yin, Tie-Yan Liu,
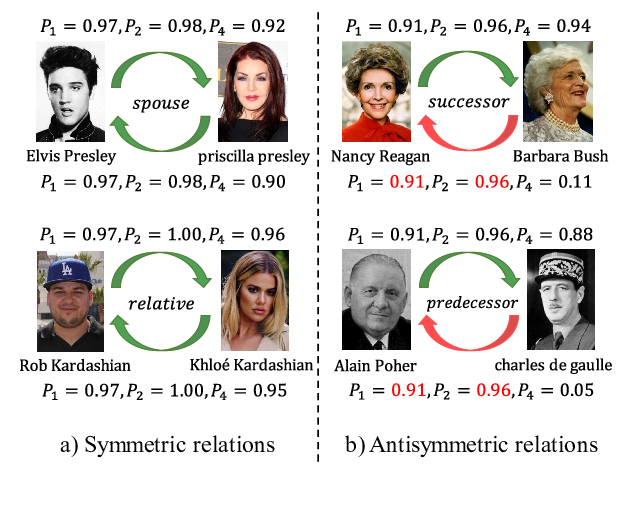