Topic Balancing with Additive Regularization of Topic Models
Eugeniia Veselova, Konstantin Vorontsov
Student Research Workshop SRW Paper
Session 2A: Jul 6
(08:00-09:00 GMT)
Session 14A: Jul 8
(17:00-18:00 GMT)
Abstract:
This article proposes a new approach for building topic models on unbalanced collections in topic modelling, based on the existing methods and our experiments with such methods. Real-world data collections contain topics in various proportions, and often documents of the relatively small theme become distributed all over the larger topics instead of being grouped into one topic. To address this issue, we design a new regularizer for Theta and Phi matrices in probabilistic Latent Semantic Analysis (pLSA) model. We make sure this regularizer increases the quality of topic models, trained on unbalanced collections. Besides, we conceptually support this regularizer by our experiments.
You can open the
pre-recorded video
in a separate window.
NOTE: The SlidesLive video may display a random order of the authors.
The correct author list is shown at the top of this webpage.
Similar Papers
Neural Topic Modeling with Bidirectional Adversarial Training
Rui Wang, Xuemeng Hu, Deyu Zhou, Yulan He, Yuxuan Xiong, Chenchen Ye, Haiyang Xu,
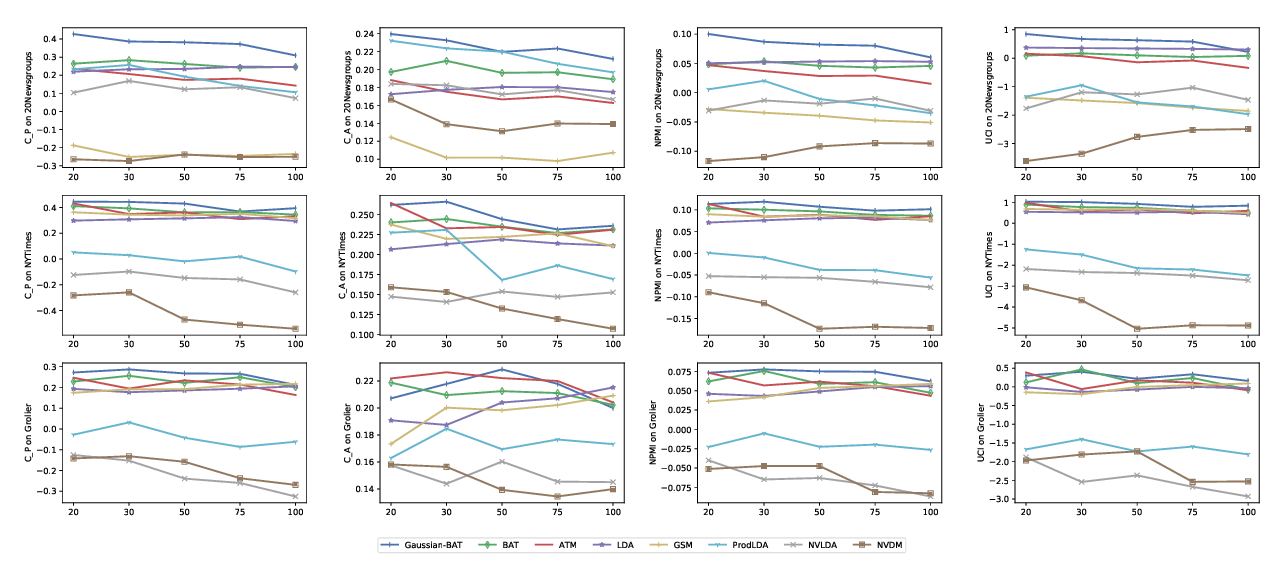
Neural Mixed Counting Models for Dispersed Topic Discovery
Jiemin Wu, Yanghui Rao, Zusheng Zhang, Haoran Xie, Qing Li, Fu Lee Wang, Ziye Chen,
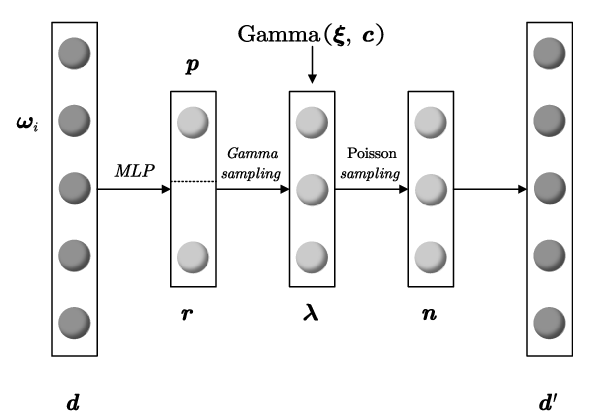
Integrating Semantic and Structural Information with Graph Convolutional Network for Controversy Detection
Lei Zhong, Juan Cao, Qiang Sheng, Junbo Guo, Ziang Wang,
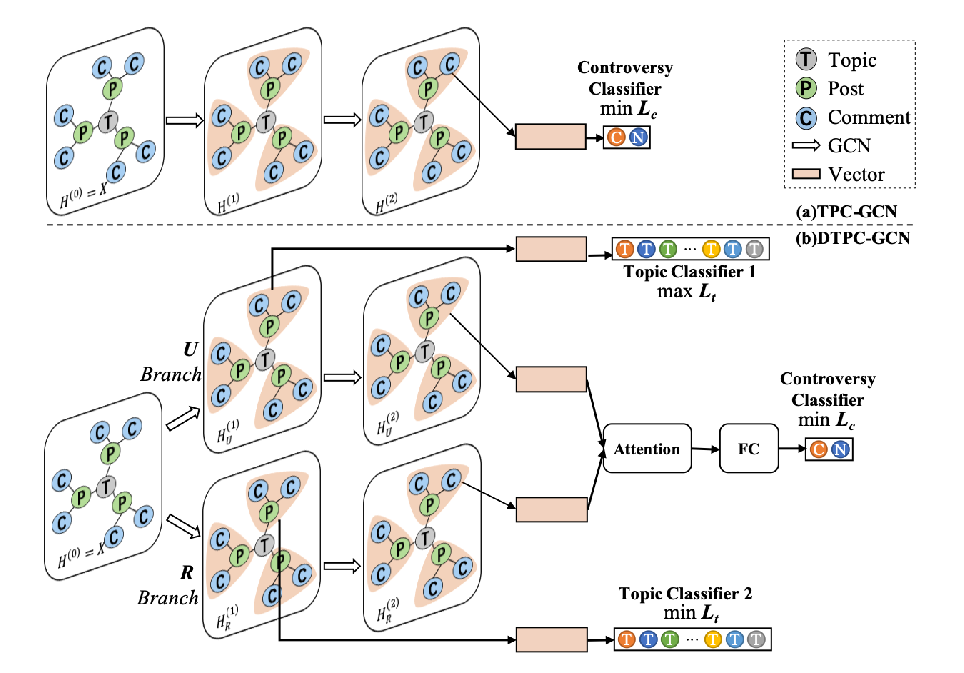
Tree-Structured Neural Topic Model
Masaru Isonuma, Junichiro Mori, Danushka Bollegala, Ichiro Sakata,
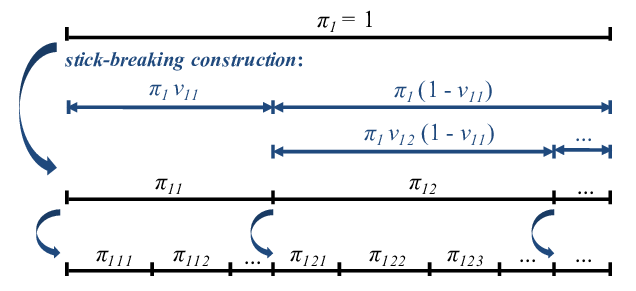