Cross-Linguistic Syntactic Evaluation of Word Prediction Models
Aaron Mueller, Garrett Nicolai, Panayiota Petrou-Zeniou, Natalia Talmina, Tal Linzen
Interpretability and Analysis of Models for NLP Long Paper
Session 9B: Jul 7
(18:00-19:00 GMT)
Session 10A: Jul 7
(20:00-21:00 GMT)
Abstract:
A range of studies have concluded that neural word prediction models can distinguish grammatical from ungrammatical sentences with high accuracy. However, these studies are based primarily on monolingual evidence from English. To investigate how these models' ability to learn syntax varies by language, we introduce CLAMS (Cross-Linguistic Assessment of Models on Syntax), a syntactic evaluation suite for monolingual and multilingual models. CLAMS includes subject-verb agreement challenge sets for English, French, German, Hebrew and Russian, generated from grammars we develop. We use CLAMS to evaluate LSTM language models as well as monolingual and multilingual BERT. Across languages, monolingual LSTMs achieved high accuracy on dependencies without attractors, and generally poor accuracy on agreement across object relative clauses. On other constructions, agreement accuracy was generally higher in languages with richer morphology. Multilingual models generally underperformed monolingual models. Multilingual BERT showed high syntactic accuracy on English, but noticeable deficiencies in other languages.
You can open the
pre-recorded video
in a separate window.
NOTE: The SlidesLive video may display a random order of the authors.
The correct author list is shown at the top of this webpage.
Similar Papers
Finding Universal Grammatical Relations in Multilingual BERT
Ethan A. Chi, John Hewitt, Christopher D. Manning,
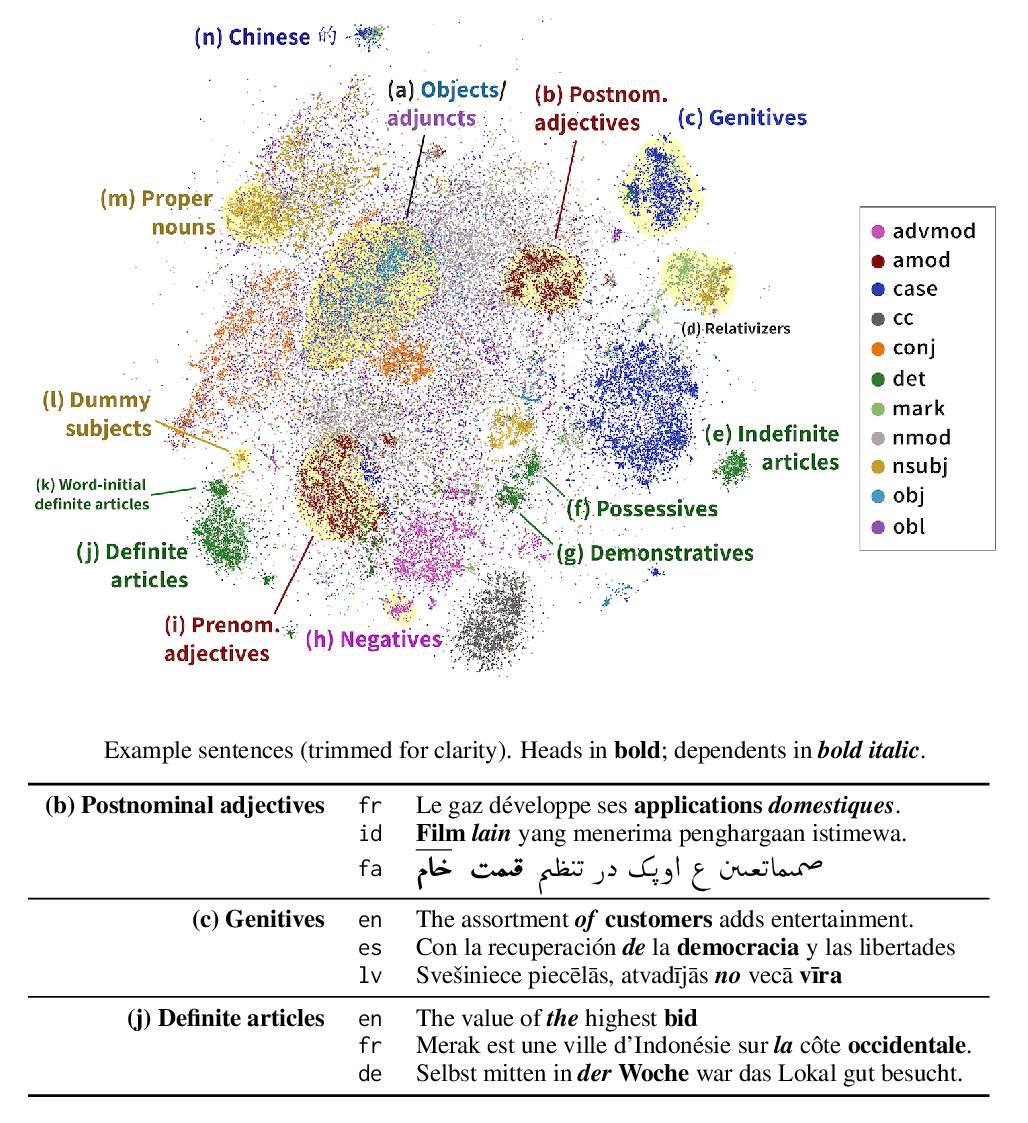
GLUECoS: An Evaluation Benchmark for Code-Switched NLP
Simran Khanuja, Sandipan Dandapat, Anirudh Srinivasan, Sunayana Sitaram, Monojit Choudhury,
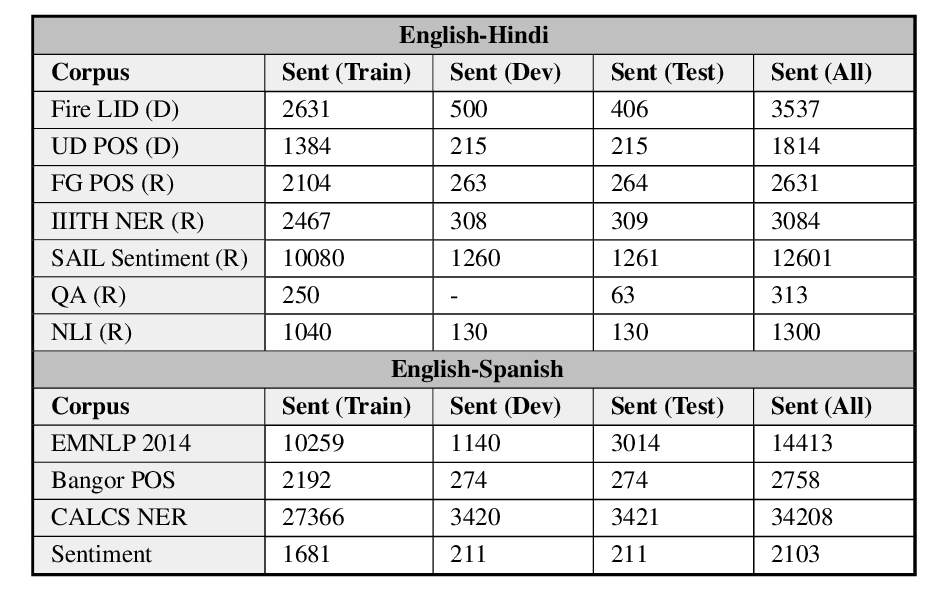
On the Cross-lingual Transferability of Monolingual Representations
Mikel Artetxe, Sebastian Ruder, Dani Yogatama,
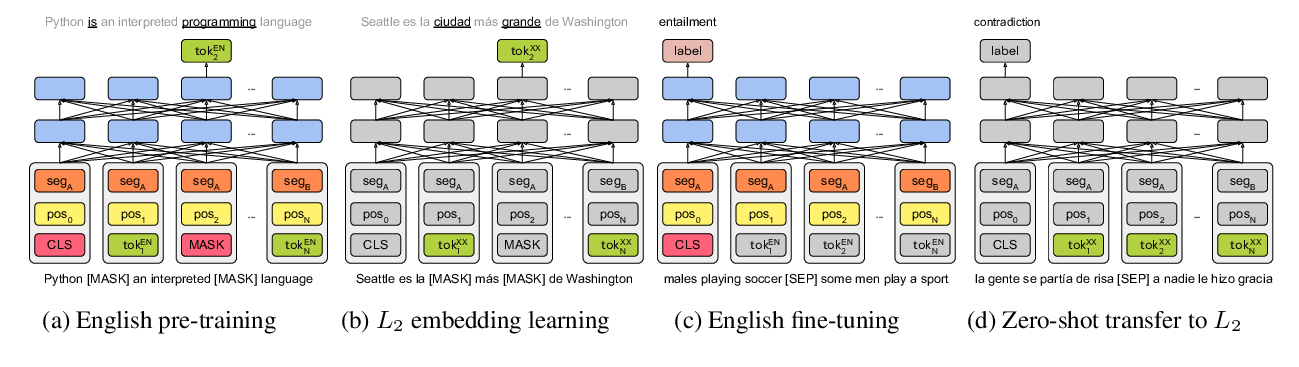
Structure-Level Knowledge Distillation For Multilingual Sequence Labeling
Xinyu Wang, Yong Jiang, Nguyen Bach, Tao Wang, Fei Huang, Kewei Tu,
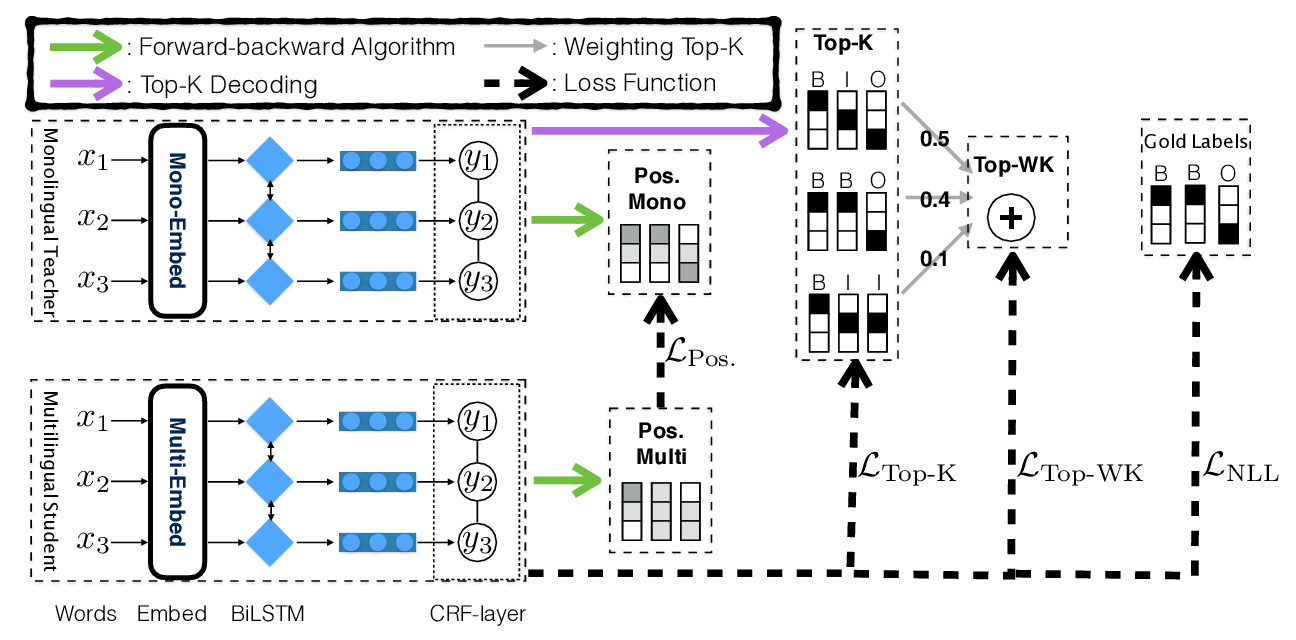