Benefits of Intermediate Annotations in Reading Comprehension
Dheeru Dua, Sameer Singh, Matt Gardner
Question Answering Short Paper
Session 9B: Jul 7
(18:00-19:00 GMT)
Session 10A: Jul 7
(20:00-21:00 GMT)
Abstract:
Complex compositional reading comprehension datasets require performing latent sequential decisions that are learned via supervision from the final answer. A large combinatorial space of possible decision paths that result in the same answer, compounded by the lack of intermediate supervision to help choose the right path, makes the learning particularly hard for this task. In this work, we study the benefits of collecting intermediate reasoning supervision along with the answer during data collection. We find that these intermediate annotations can provide two-fold benefits. First, we observe that for any collection budget, spending a fraction of it on intermediate annotations results in improved model performance, for two complex compositional datasets: DROP and Quoref. Second, these annotations encourage the model to learn the correct latent reasoning steps, helping combat some of the biases introduced during the data collection process.
You can open the
pre-recorded video
in a separate window.
NOTE: The SlidesLive video may display a random order of the authors.
The correct author list is shown at the top of this webpage.
Similar Papers
Obtaining Faithful Interpretations from Compositional Neural Networks
Sanjay Subramanian, Ben Bogin, Nitish Gupta, Tomer Wolfson, Sameer Singh, Jonathan Berant, Matt Gardner,
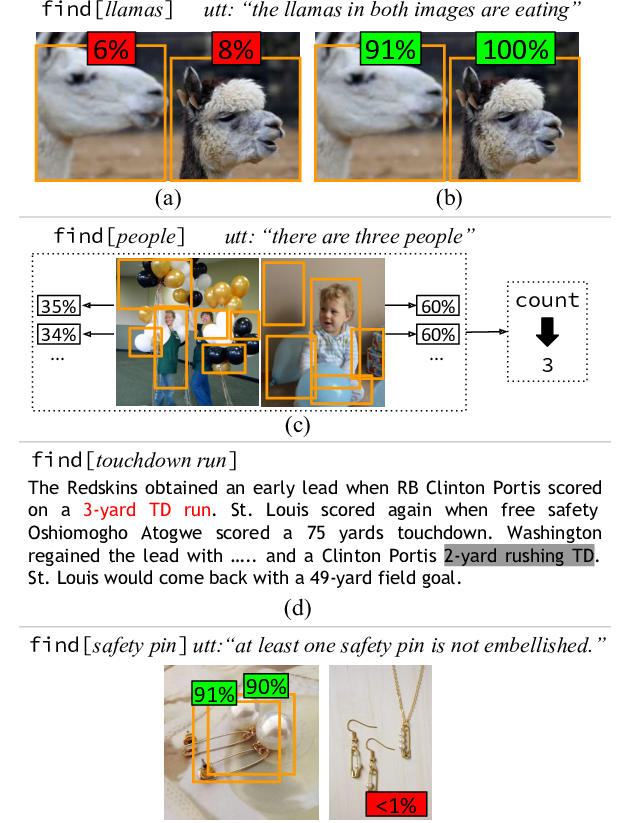
Intermediate-Task Transfer Learning with Pretrained Language Models: When and Why Does It Work?
Yada Pruksachatkun, Jason Phang, Haokun Liu, Phu Mon Htut, Xiaoyi Zhang, Richard Yuanzhe Pang, Clara Vania, Katharina Kann, Samuel R. Bowman,
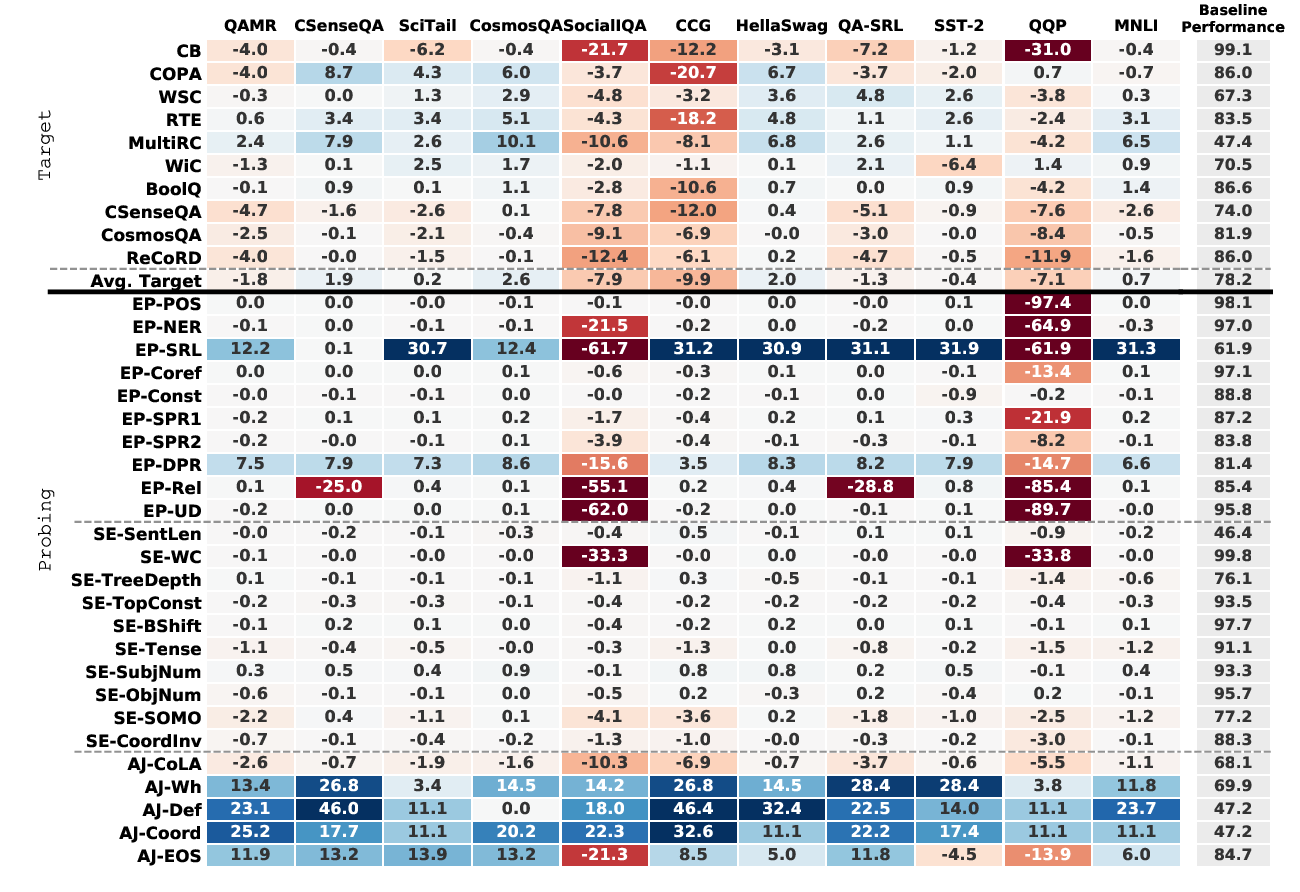
STARC: Structured Annotations for Reading Comprehension
Yevgeni Berzak, Jonathan Malmaud, Roger Levy,
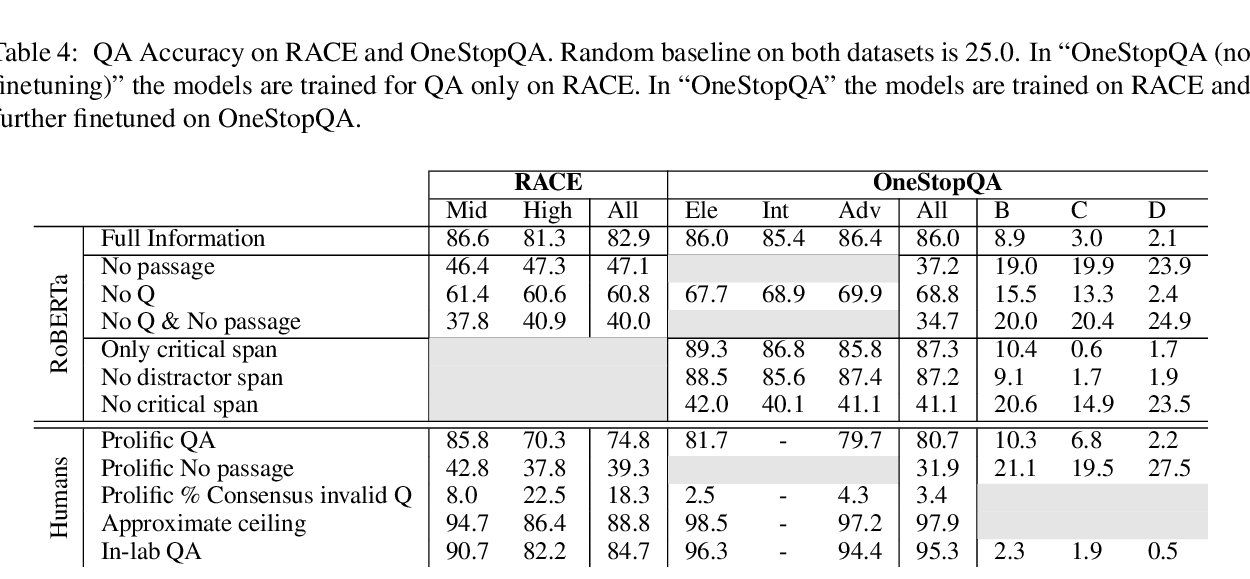
Compositional Generalization by Factorizing Alignment and Translation
Jacob Russin, Jason Jo, Randall O'Reilly, Yoshua Bengio,
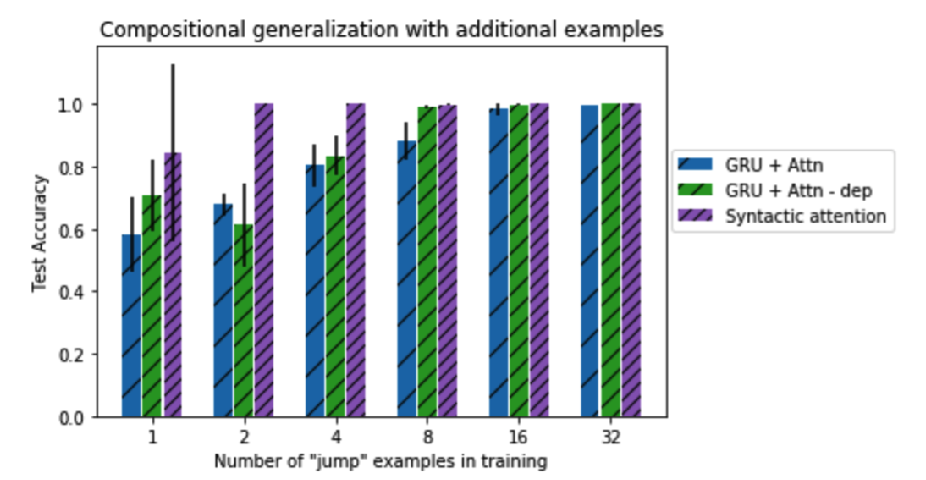