Improving Low-Resource Named Entity Recognition using Joint Sentence and Token Labeling
Canasai Kruengkrai, Thien Hai Nguyen, Sharifah Mahani Aljunied, Lidong Bing
Information Extraction Short Paper
Session 11A: Jul 8
(05:00-06:00 GMT)
Session 12A: Jul 8
(08:00-09:00 GMT)
Abstract:
Exploiting sentence-level labels, which are easy to obtain, is one of the plausible methods to improve low-resource named entity recognition (NER), where token-level labels are costly to annotate. Current models for jointly learning sentence and token labeling are limited to binary classification. We present a joint model that supports multi-class classification and introduce a simple variant of self-attention that allows the model to learn scaling factors. Our model produces 3.78%, 4.20%, 2.08% improvements in F1 over the BiLSTM-CRF baseline on e-commerce product titles in three different low-resource languages: Vietnamese, Thai, and Indonesian, respectively.
You can open the
pre-recorded video
in a separate window.
NOTE: The SlidesLive video may display a random order of the authors.
The correct author list is shown at the top of this webpage.
Similar Papers
A Unified MRC Framework for Named Entity Recognition
Xiaoya Li, Jingrong Feng, Yuxian Meng, Qinghong Han, Fei Wu, Jiwei Li,
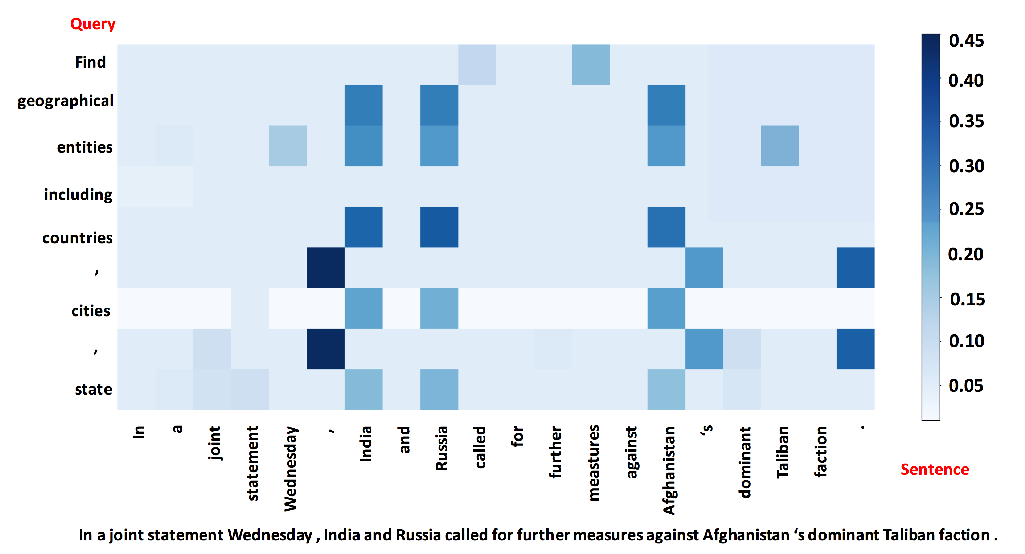
Hypernymy Detection for Low-Resource Languages via Meta Learning
Changlong Yu, Jialong Han, Haisong Zhang, Wilfred Ng,
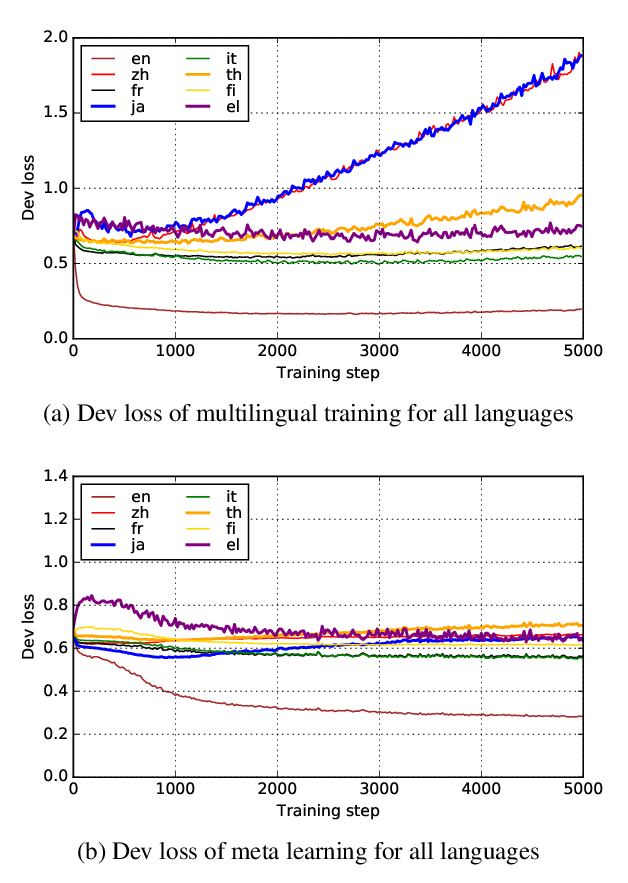
Embeddings of Label Components for Sequence Labeling: A Case Study of Fine-grained Named Entity Recognition
Takuma Kato, Kaori Abe, Hiroki Ouchi, Shumpei Miyawaki, Jun Suzuki, Kentaro Inui,
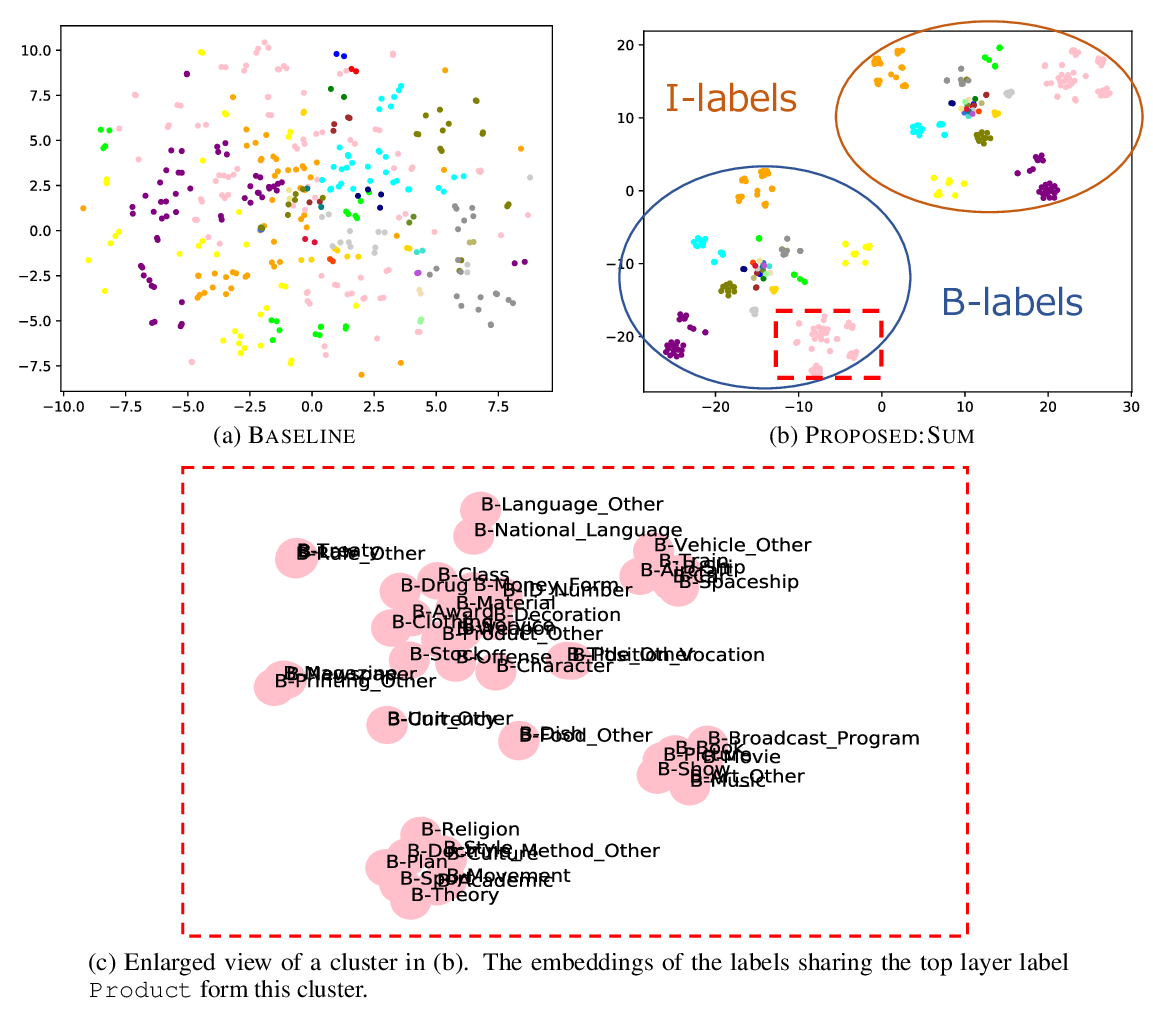
LEAN-LIFE: A Label-Efficient Annotation Framework Towards Learning from Explanation
Dong-Ho Lee, Rahul Khanna, Bill Yuchen Lin, Seyeon Lee, Qinyuan Ye, Elizabeth Boschee, Leonardo Neves, Xiang Ren,
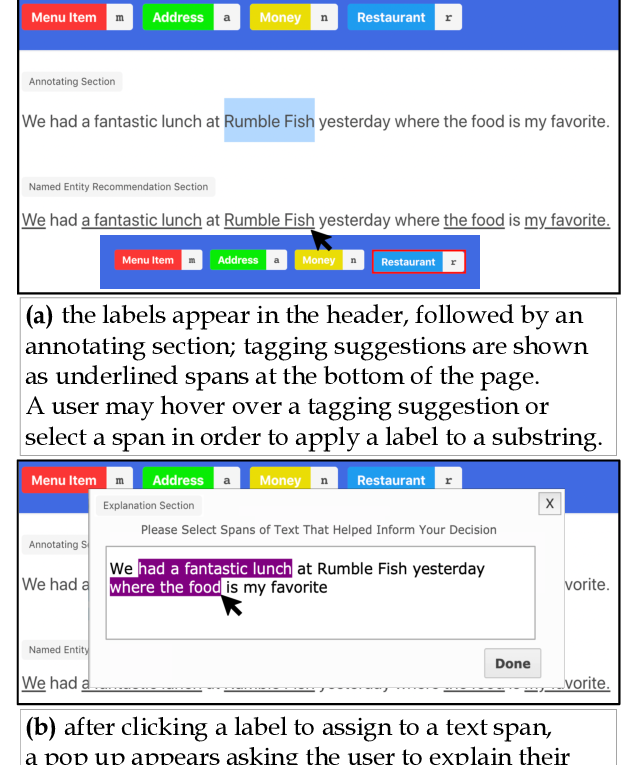