Hypernymy Detection for Low-Resource Languages via Meta Learning
Changlong Yu, Jialong Han, Haisong Zhang, Wilfred Ng
Semantics: Lexical Short Paper
Session 6B: Jul 7
(06:00-07:00 GMT)
Session 8A: Jul 7
(12:00-13:00 GMT)
Abstract:
Hypernymy detection, a.k.a, lexical entailment, is a fundamental sub-task of many natural language understanding tasks. Previous explorations mostly focus on monolingual hypernymy detection on high-resource languages, e.g., English, but few investigate the low-resource scenarios. This paper addresses the problem of low-resource hypernymy detection by combining high-resource languages. We extensively compare three joint training paradigms and for the first time propose applying meta learning to relieve the low-resource issue. Experiments demonstrate the superiority of our method among the three settings, which substantially improves the performance of extremely low-resource languages by preventing over-fitting on small datasets.
You can open the
pre-recorded video
in a separate window.
NOTE: The SlidesLive video may display a random order of the authors.
The correct author list is shown at the top of this webpage.
Similar Papers
Improving Candidate Generation for Low-resource Cross-lingual Entity Linking
Shuyan Zhou, Shruti Rijhwani, John Wieting, Jaime Carbonell, Graham Neubig,
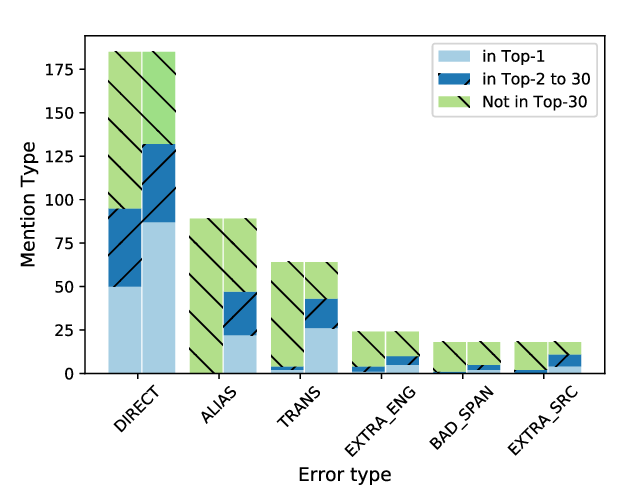
Leveraging Monolingual Data with Self-Supervision for Multilingual Neural Machine Translation
Aditya Siddhant, Ankur Bapna, Yuan Cao, Orhan Firat, Mia Chen, Sneha Kudugunta, Naveen Arivazhagan, Yonghui Wu,
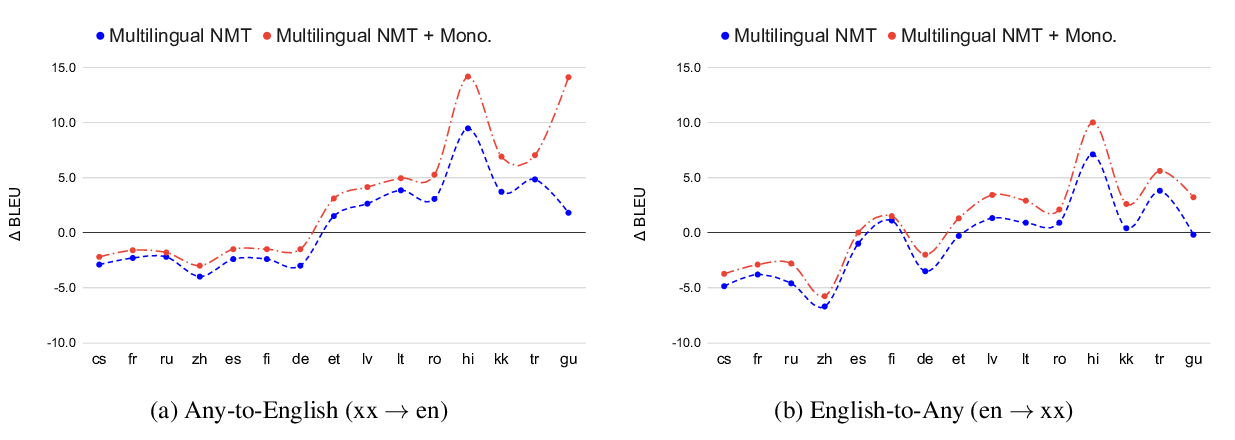
Improving Low-Resource Named Entity Recognition using Joint Sentence and Token Labeling
Canasai Kruengkrai, Thien Hai Nguyen, Sharifah Mahani Aljunied, Lidong Bing,
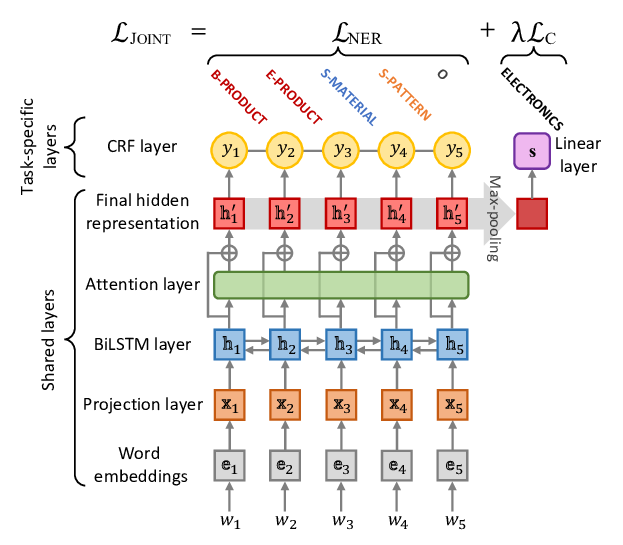
Soft Gazetteers for Low-Resource Named Entity Recognition
Shruti Rijhwani, Shuyan Zhou, Graham Neubig, Jaime Carbonell,
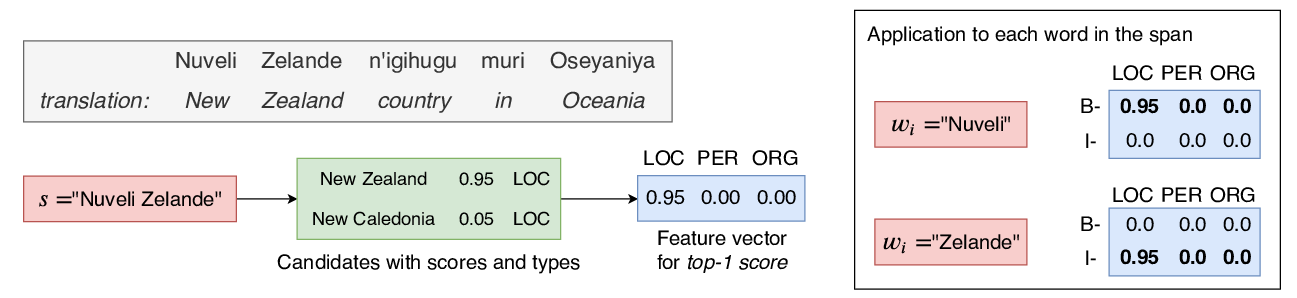