Paraphrase Generation by Learning How to Edit from Samples
Amirhossein Kazemnejad, Mohammadreza Salehi, Mahdieh Soleymani Baghshah
NLP Applications Long Paper
Session 11A: Jul 8
(05:00-06:00 GMT)
Session 13A: Jul 8
(12:00-13:00 GMT)
Abstract:
Neural sequence to sequence text generation has been proved to be a viable approach to paraphrase generation. Despite promising results, paraphrases generated by these models mostly suffer from lack of quality and diversity. To address these problems, we propose a novel retrieval-based method for paraphrase generation. Our model first retrieves a paraphrase pair similar to the input sentence from a pre-defined index. With its novel editor module, the model then paraphrases the input sequence by editing it using the extracted relations between the retrieved pair of sentences. In order to have fine-grained control over the editing process, our model uses the newly introduced concept of Micro Edit Vectors. It both extracts and exploits these vectors using the attention mechanism in the Transformer architecture. Experimental results show the superiority of our paraphrase generation method in terms of both automatic metrics, and human evaluation of relevance, grammaticality, and diversity of generated paraphrases.
You can open the
pre-recorded video
in a separate window.
NOTE: The SlidesLive video may display a random order of the authors.
The correct author list is shown at the top of this webpage.
Similar Papers
Unsupervised Paraphrasing by Simulated Annealing
Xianggen Liu, Lili Mou, Fandong Meng, Hao Zhou, Jie Zhou, Sen Song,
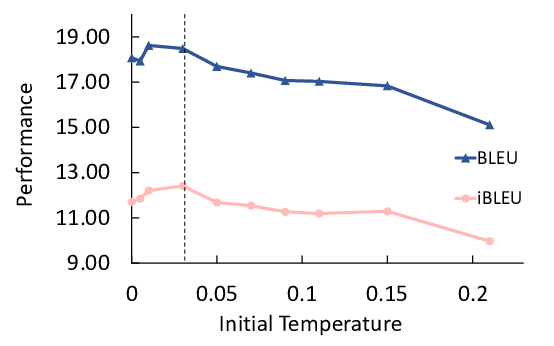