Pointwise Paraphrase Appraisal is Potentially Problematic
Hannah Chen, Yangfeng Ji, David Evans
Student Research Workshop SRW Paper
Session 3B: Jul 6
(13:00-14:00 GMT)
Session 14B: Jul 8
(18:00-19:00 GMT)
Abstract:
The prevailing approach for training and evaluating paraphrase identification models is constructed as a binary classification problem: the model is given a pair of sentences, and is judged by how accurately it classifies pairs as either paraphrases or non-paraphrases. This pointwise-based evaluation method does not match well the objective of most real world applications, so the goal of our work is to understand how models which perform well under pointwise evaluation may fail in practice and find better methods for evaluating paraphrase identification models. As a first step towards that goal, we show that although the standard way of fine-tuning BERT for paraphrase identification by pairing two sentences as one sequence results in a model with state-of-the-art performance, that model may perform poorly on simple tasks like identifying pairs with two identical sentences. Moreover, we show that these models may even predict a pair of randomly-selected sentences with higher paraphrase score than a pair of identical ones.
You can open the
pre-recorded video
in a separate window.
NOTE: The SlidesLive video may display a random order of the authors.
The correct author list is shown at the top of this webpage.
Similar Papers
Paraphrase Generation by Learning How to Edit from Samples
Amirhossein Kazemnejad, Mohammadreza Salehi, Mahdieh Soleymani Baghshah,
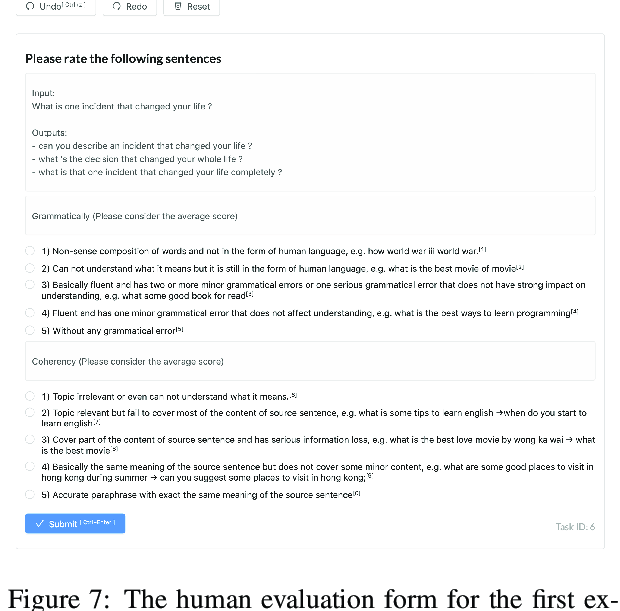
Unsupervised Paraphrasing by Simulated Annealing
Xianggen Liu, Lili Mou, Fandong Meng, Hao Zhou, Jie Zhou, Sen Song,
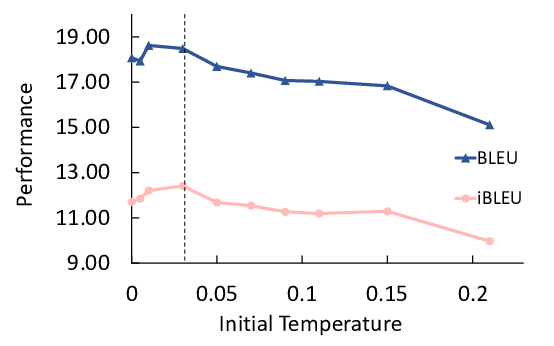
Facet-Aware Evaluation for Extractive Summarization
Yuning Mao, Liyuan Liu, Qi Zhu, Xiang Ren, Jiawei Han,
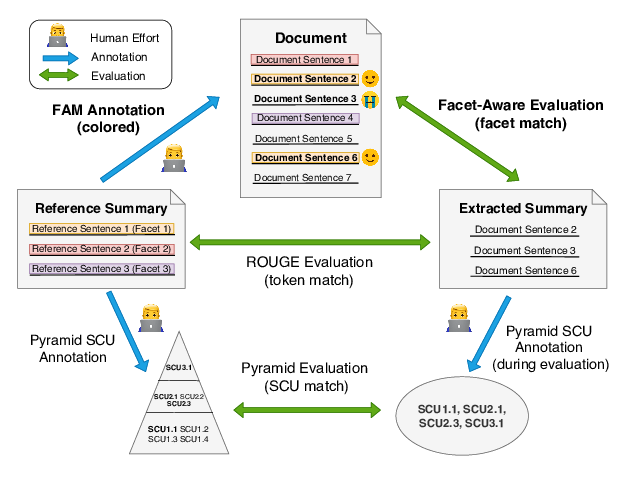