The Unstoppable Rise of Computational Linguistics in Deep Learning
James Henderson
Theme Long Paper
Session 11A: Jul 8
(05:00-06:00 GMT)
Session 15B: Jul 8
(21:00-22:00 GMT)
Abstract:
In this paper, we trace the history of neural networks applied to natural language understanding tasks, and identify key contributions which the nature of language has made to the development of neural network architectures. We focus on the importance of variable binding and its instantiation in attention-based models, and argue that Transformer is not a sequence model but an induced-structure model. This perspective leads to predictions of the challenges facing research in deep learning architectures for natural language understanding.
You can open the
pre-recorded video
in a separate window.
NOTE: The SlidesLive video may display a random order of the authors.
The correct author list is shown at the top of this webpage.
Similar Papers
From SPMRL to NMRL: What Did We Learn (and Unlearn) in a Decade of Parsing Morphologically-Rich Languages (MRLs)?
Reut Tsarfaty, Dan Bareket, Stav Klein, Amit Seker,
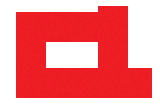
Probing for Referential Information in Language Models
Ionut-Teodor Sorodoc, Kristina Gulordava, Gemma Boleda,
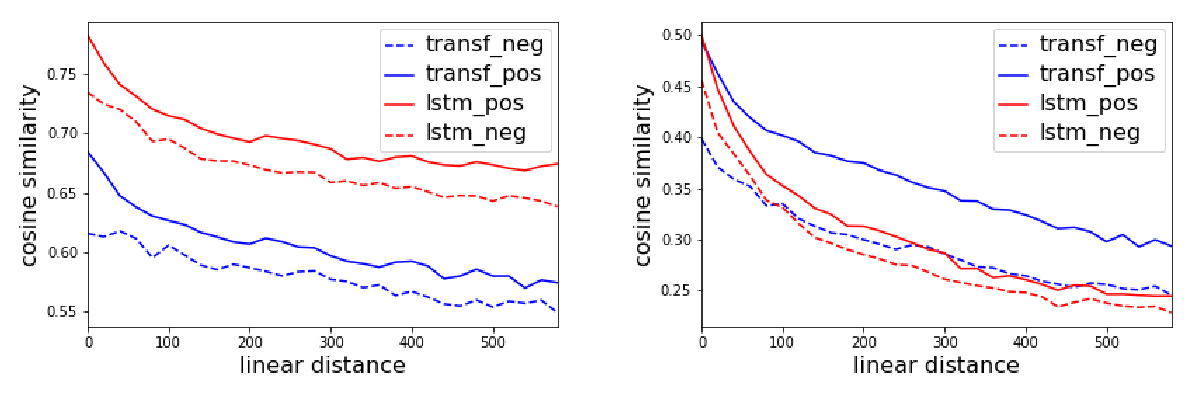
Climbing towards NLU: On Meaning, Form, and Understanding in the Age of Data
Emily M. Bender, Alexander Koller,
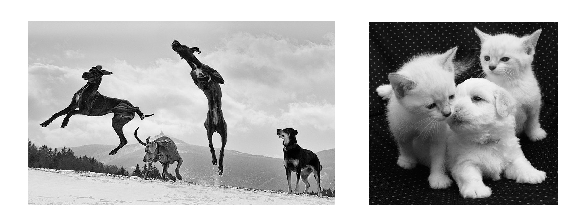