Probing for Referential Information in Language Models
Ionut-Teodor Sorodoc, Kristina Gulordava, Gemma Boleda
Interpretability and Analysis of Models for NLP Long Paper
Session 7B: Jul 7
(09:00-10:00 GMT)
Session 8A: Jul 7
(12:00-13:00 GMT)
Abstract:
Language models keep track of complex information about the preceding context -- including, e.g., syntactic relations in a sentence. We investigate whether they also capture information beneficial for resolving pronominal anaphora in English. We analyze two state of the art models with LSTM and Transformer architectures, via probe tasks and analysis on a coreference annotated corpus. The Transformer outperforms the LSTM in all analyses. Our results suggest that language models are more successful at learning grammatical constraints than they are at learning truly referential information, in the sense of capturing the fact that we use language to refer to entities in the world. However, we find traces of the latter aspect, too.
You can open the
pre-recorded video
in a separate window.
NOTE: The SlidesLive video may display a random order of the authors.
The correct author list is shown at the top of this webpage.
Similar Papers
Influence Paths for Characterizing Subject-Verb Number Agreement in LSTM Language Models
Kaiji Lu, Piotr Mardziel, Klas Leino, Matt Fredrikson, Anupam Datta,
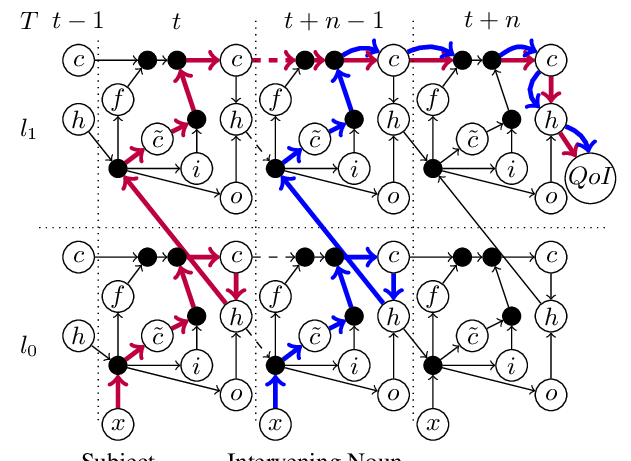
How much complexity does an RNN architecture need to learn syntax-sensitive dependencies?
Gantavya Bhatt, Hritik Bansal, Rishubh Singh, Sumeet Agarwal,
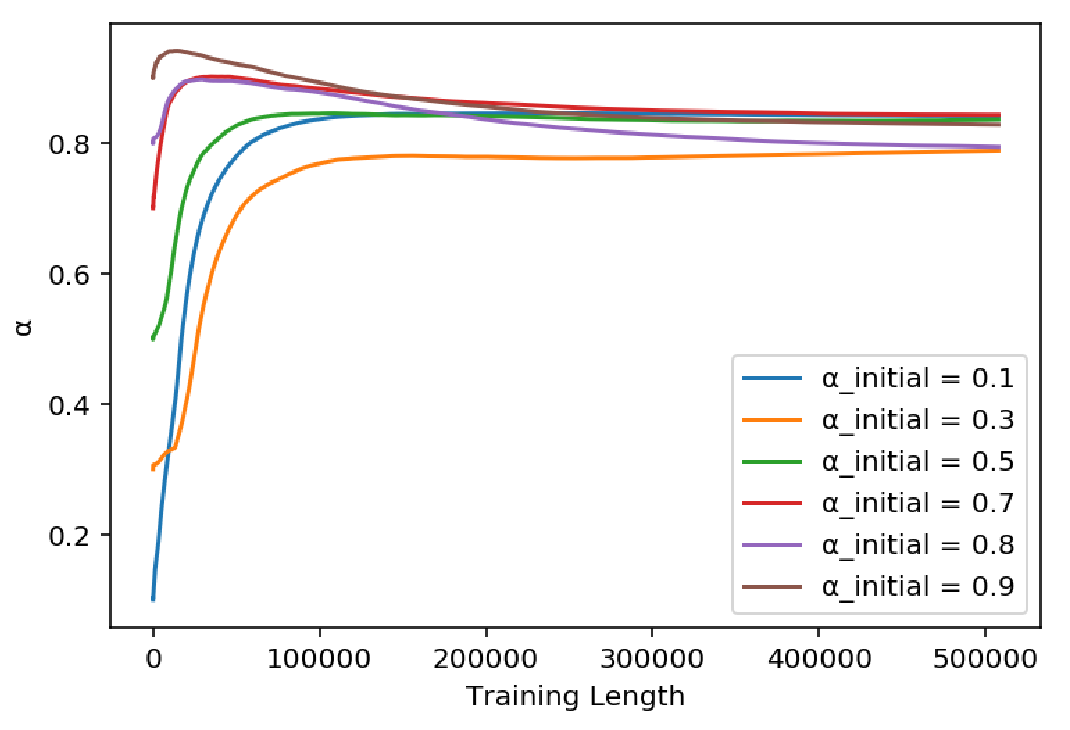
A Systematic Assessment of Syntactic Generalization in Neural Language Models
Jennifer Hu, Jon Gauthier, Peng Qian, Ethan Wilcox, Roger Levy,
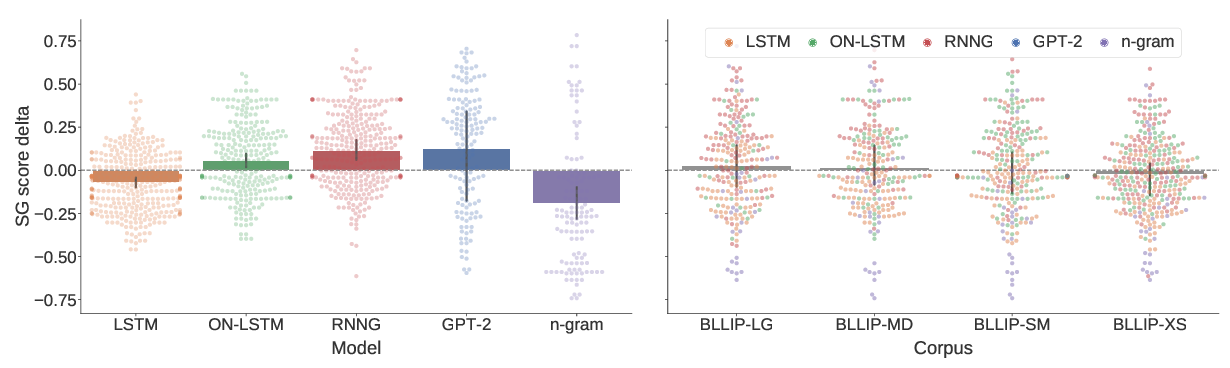
Do Neural Language Models Show Preferences for Syntactic Formalisms?
Artur Kulmizev, Vinit Ravishankar, Mostafa Abdou, Joakim Nivre,
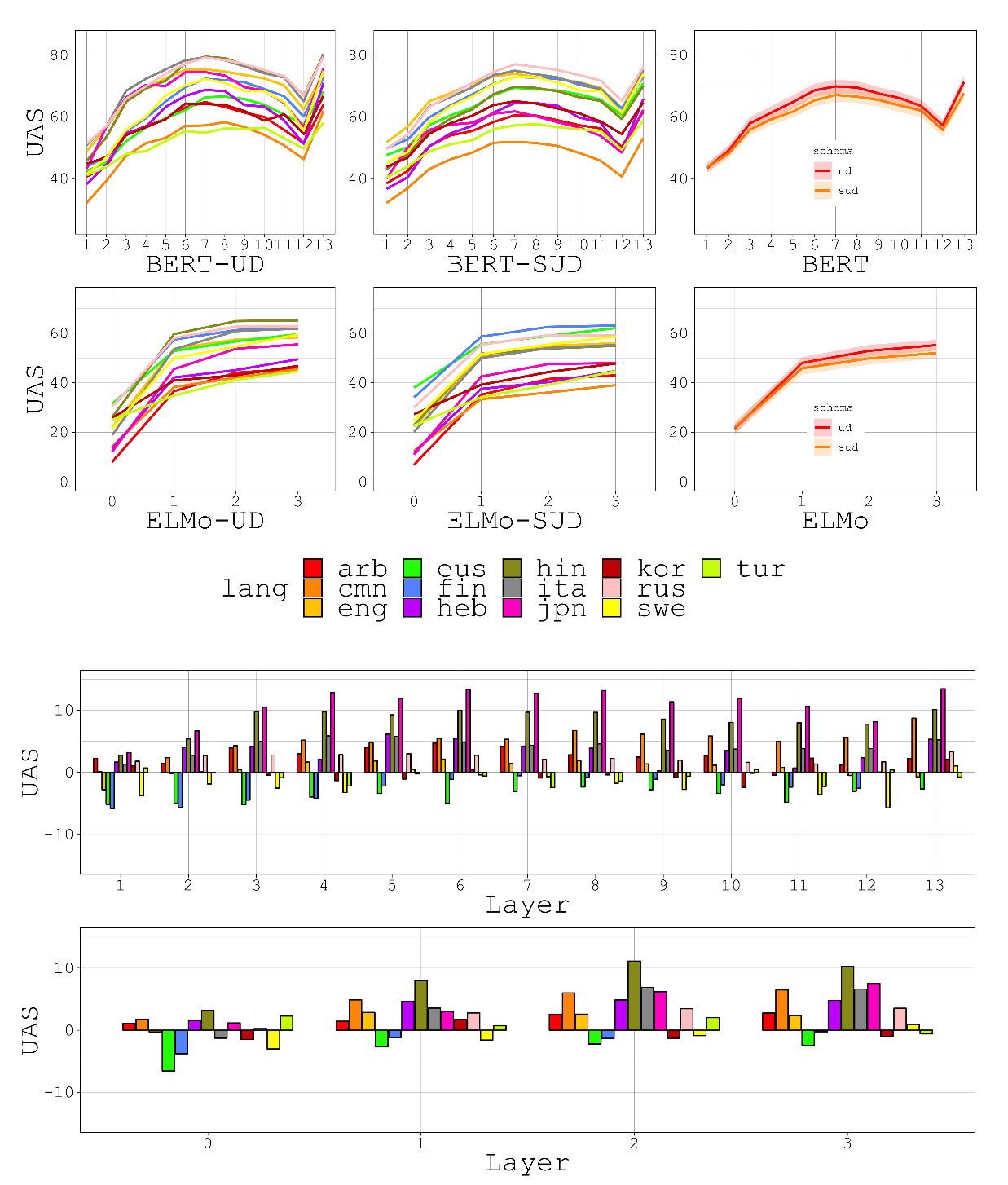