Learning Low-Resource End-To-End Goal-Oriented Dialog for Fast and Reliable System Deployment
Yinpei Dai, Hangyu Li, Chengguang Tang, Yongbin Li, Jian Sun, Xiaodan Zhu
Dialogue and Interactive Systems Short Paper
Session 1B: Jul 6
(06:00-07:00 GMT)
Session 2A: Jul 6
(08:00-09:00 GMT)
Abstract:
Existing end-to-end dialog systems perform less effectively when data is scarce. To obtain an acceptable success in real-life online services with only a handful of training examples, both fast adaptability and reliable performance are highly desirable for dialog systems. In this paper, we propose the Meta-Dialog System (MDS), which combines the advantages of both meta-learning approaches and human-machine collaboration. We evaluate our methods on a new extended-bAbI dataset and a transformed MultiWOZ dataset for low-resource goal-oriented dialog learning. Experimental results show that MDS significantly outperforms non-meta-learning baselines and can achieve more than 90% per-turn accuracies with only 10 dialogs on the extended-bAbI dataset.
You can open the
pre-recorded video
in a separate window.
NOTE: The SlidesLive video may display a random order of the authors.
The correct author list is shown at the top of this webpage.
Similar Papers
Conversation Learner - A Machine Teaching Tool for Building Dialog Managers for Task-Oriented Dialog Systems
Swadheen Shukla, Lars Liden, Shahin Shayandeh, Eslam Kamal, Jinchao Li, Matt Mazzola, Thomas Park, Baolin Peng, Jianfeng Gao,
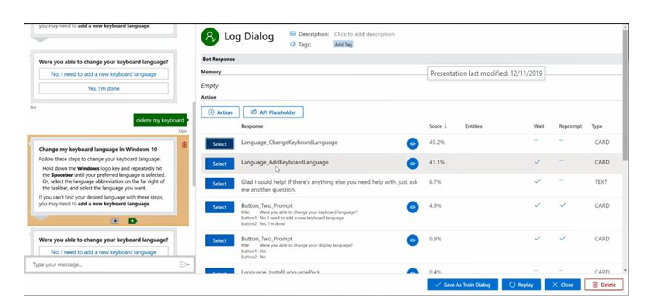
Towards Conversational Recommendation over Multi-Type Dialogs
Zeming Liu, Haifeng Wang, Zheng-Yu Niu, Hua Wu, Wanxiang Che, Ting Liu,
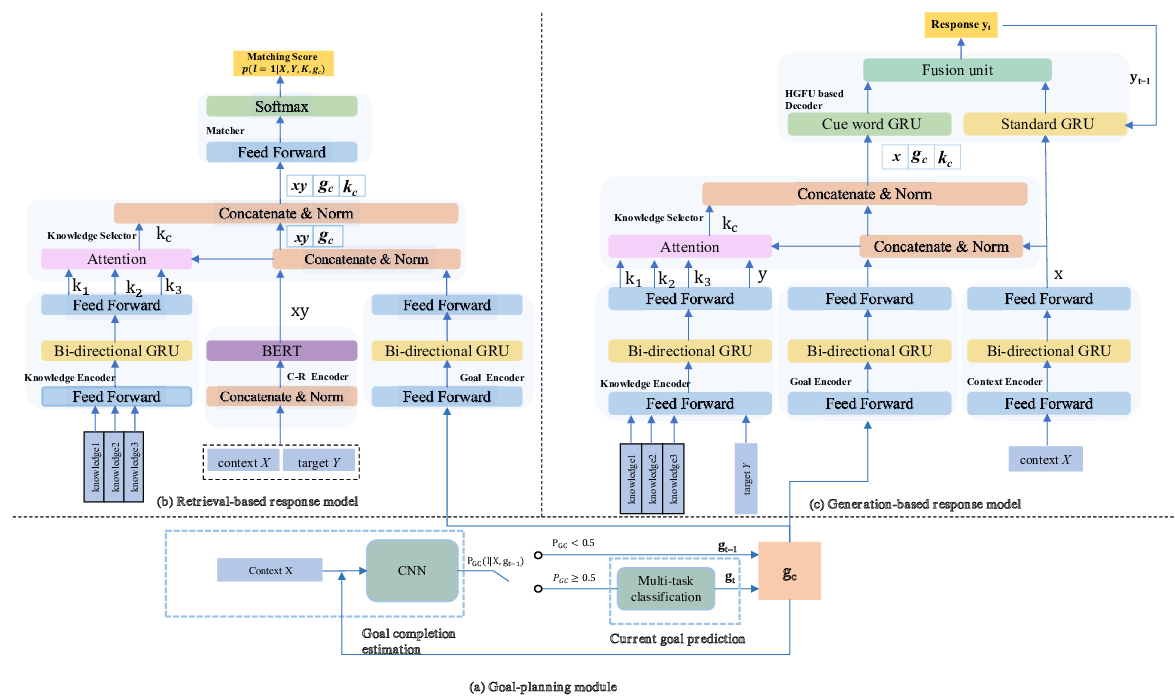
USR: An Unsupervised and Reference Free Evaluation Metric for Dialog Generation
Shikib Mehri, Maxine Eskenazi,
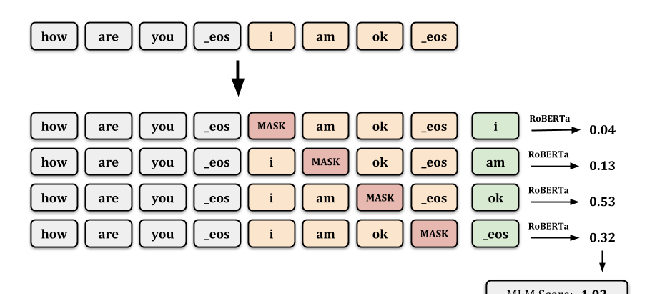