Amalgamation of protein sequence, structure and textual information for improving protein-protein interaction identification
Pratik Dutta, Sriparna Saha
Information Extraction Long Paper
Session 11B: Jul 8
(06:00-07:00 GMT)
Session 12A: Jul 8
(08:00-09:00 GMT)
Abstract:
An in-depth exploration of protein-protein interactions (PPI) is essential to understand the metabolism in addition to the regulations of biological entities like proteins, carbohydrates, and many more. Most of the recent PPI tasks in BioNLP domain have been carried out solely using textual data. In this paper, we argue that incorporating multimodal cues can improve the automatic identification of PPI. As a first step towards enabling the development of multimodal approaches for PPI identification, we have developed two multi-modal datasets which are extensions and multi-modal versions of two popular benchmark PPI corpora (BioInfer and HRPD50). Besides, existing textual modalities, two new modalities, 3D protein structure and underlying genomic sequence, are also added to each instance. Further, a novel deep multi-modal architecture is also implemented to efficiently predict the protein interactions from the developed datasets. A detailed experimental analysis reveals the superiority of the multi-modal approach in comparison to the strong baselines including unimodal approaches and state-of the-art methods over both the generated multi-modal datasets. The developed multi-modal datasets are available for use at https://github.com/sduttap16/MM_PPI_NLP.
You can open the
pre-recorded video
in a separate window.
NOTE: The SlidesLive video may display a random order of the authors.
The correct author list is shown at the top of this webpage.
Similar Papers
A Novel Graph-based Multi-modal Fusion Encoder for Neural Machine Translation
Yongjing Yin, Fandong Meng, Jinsong Su, Chulun Zhou, Zhengyuan Yang, Jie Zhou, Jiebo Luo,
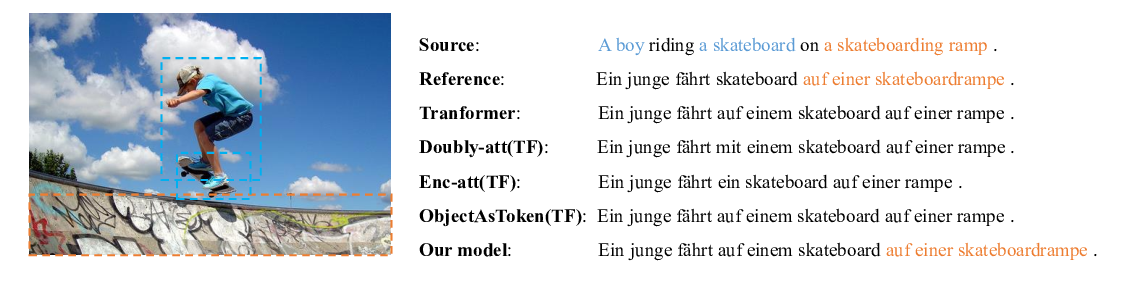
CH-SIMS: A Chinese Multimodal Sentiment Analysis Dataset with Fine-grained Annotation of Modality
Wenmeng Yu, Hua Xu, Fanyang Meng, Yilin Zhu, Yixiao Ma, Jiele Wu, Jiyun Zou, Kaicheng Yang,
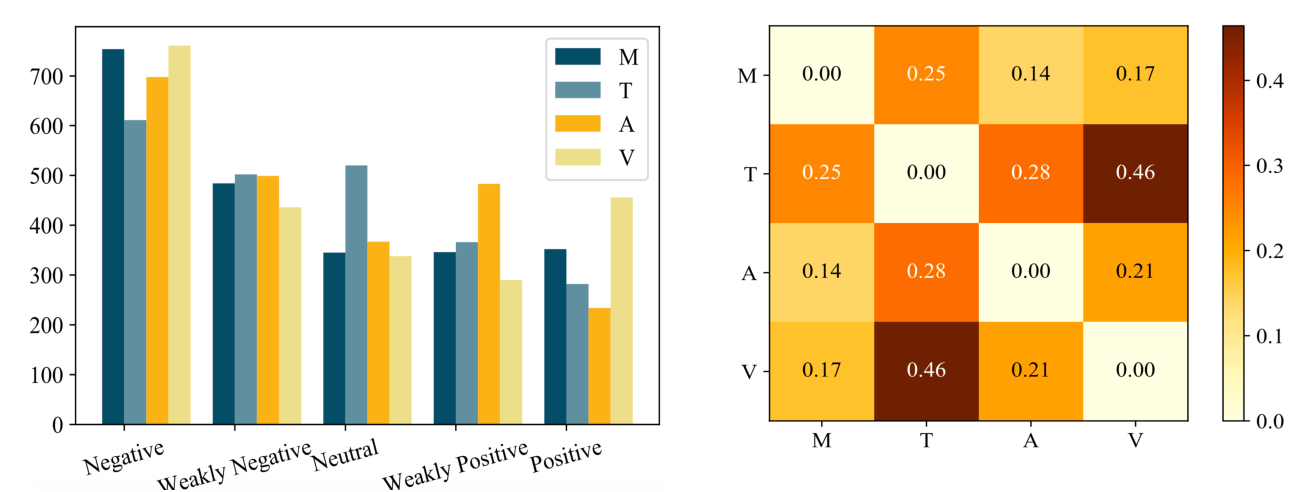
Towards Emotion-aided Multi-modal Dialogue Act Classification
Tulika Saha, Aditya Patra, Sriparna Saha, Pushpak Bhattacharyya,
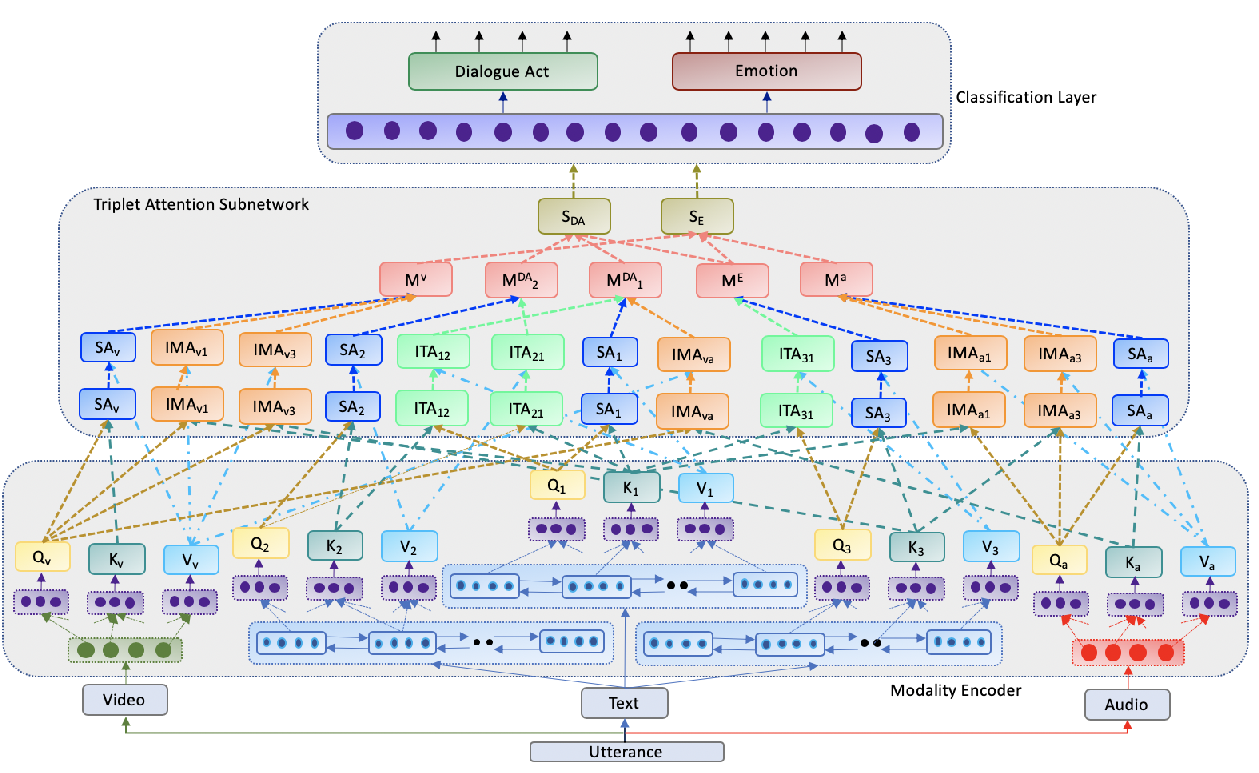
Sentiment and Emotion help Sarcasm? A Multi-task Learning Framework for Multi-Modal Sarcasm, Sentiment and Emotion Analysis
Dushyant Singh Chauhan, Dhanush S R, Asif Ekbal, Pushpak Bhattacharyya,
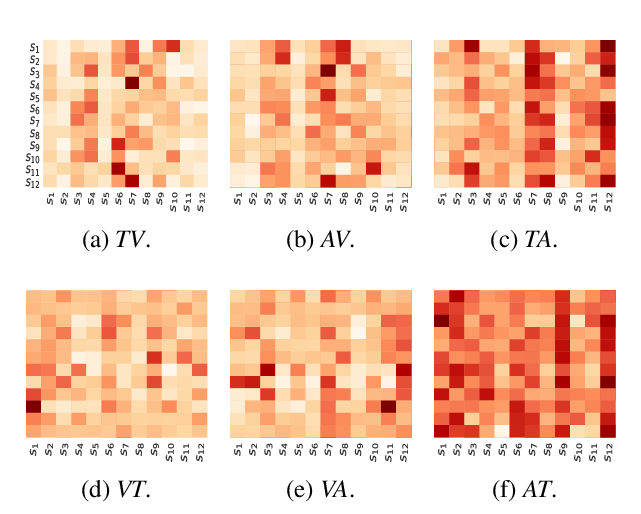