A Novel Graph-based Multi-modal Fusion Encoder for Neural Machine Translation
Yongjing Yin, Fandong Meng, Jinsong Su, Chulun Zhou, Zhengyuan Yang, Jie Zhou, Jiebo Luo
Machine Translation Long Paper
Session 6A: Jul 7
(05:00-06:00 GMT)
Session 7A: Jul 7
(08:00-09:00 GMT)
Abstract:
Multi-modal neural machine translation (NMT) aims to translate source sentences into a target language paired with images. However, dominant multi-modal NMT models do not fully exploit fine-grained semantic correspondences between semantic units of different modalities, which have potential to refine multi-modal representation learning. To deal with this issue, in this paper, we propose a novel graph-based multi-modal fusion encoder for NMT. Specifically, we first represent the input sentence and image using a unified multi-modal graph, which captures various semantic relationships between multi-modal semantic units (words and visual objects). We then stack multiple graph-based multi-modal fusion layers that iteratively perform semantic interactions to learn node representations. Finally, these representations provide an attention-based context vector for the decoder. We evaluate our proposed encoder on the Multi30K datasets. Experimental results and in-depth analysis show the superiority of our multi-modal NMT model.
You can open the
pre-recorded video
in a separate window.
NOTE: The SlidesLive video may display a random order of the authors.
The correct author list is shown at the top of this webpage.
Similar Papers
Sentiment and Emotion help Sarcasm? A Multi-task Learning Framework for Multi-Modal Sarcasm, Sentiment and Emotion Analysis
Dushyant Singh Chauhan, Dhanush S R, Asif Ekbal, Pushpak Bhattacharyya,
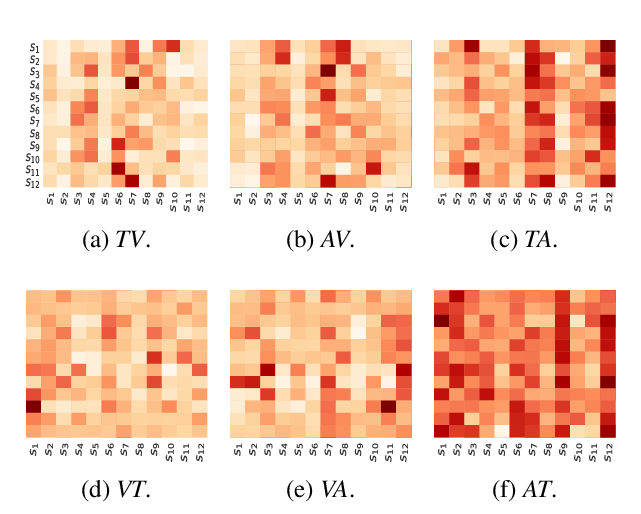
Towards Emotion-aided Multi-modal Dialogue Act Classification
Tulika Saha, Aditya Patra, Sriparna Saha, Pushpak Bhattacharyya,
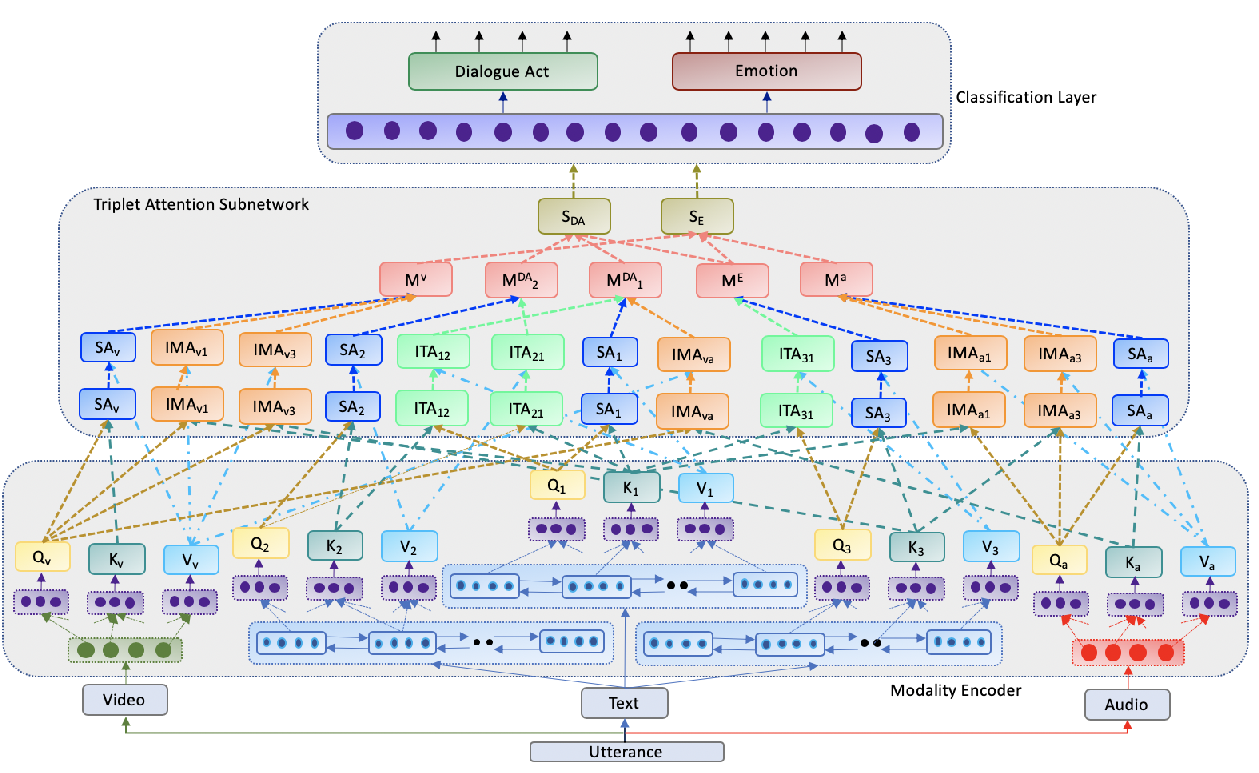
Multi-Granularity Interaction Network for Extractive and Abstractive Multi-Document Summarization
Hanqi Jin, Tianming Wang, Xiaojun Wan,
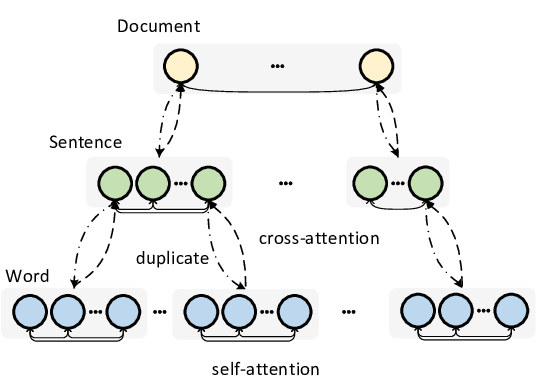