Learning to Tag OOV Tokens by Integrating Contextual Representation and Background Knowledge
Keqing He, Yuanmeng Yan, Weiran XU
Dialogue and Interactive Systems Short Paper
Session 1B: Jul 6
(06:00-07:00 GMT)
Session 3B: Jul 6
(13:00-14:00 GMT)
Abstract:
Neural-based context-aware models for slot tagging have achieved state-of-the-art performance. However, the presence of OOV(out-of-vocab) words significantly degrades the performance of neural-based models, especially in a few-shot scenario. In this paper, we propose a novel knowledge-enhanced slot tagging model to integrate contextual representation of input text and the large-scale lexical background knowledge. Besides, we use multi-level graph attention to explicitly model lexical relations. The experiments show that our proposed knowledge integration mechanism achieves consistent improvements across settings with different sizes of training data on two public benchmark datasets.
You can open the
pre-recorded video
in a separate window.
NOTE: The SlidesLive video may display a random order of the authors.
The correct author list is shown at the top of this webpage.
Similar Papers
Dialogue State Tracking with Explicit Slot Connection Modeling
Yawen Ouyang, Moxin Chen, Xinyu Dai, Yinggong Zhao, Shujian Huang, Jiajun Chen,
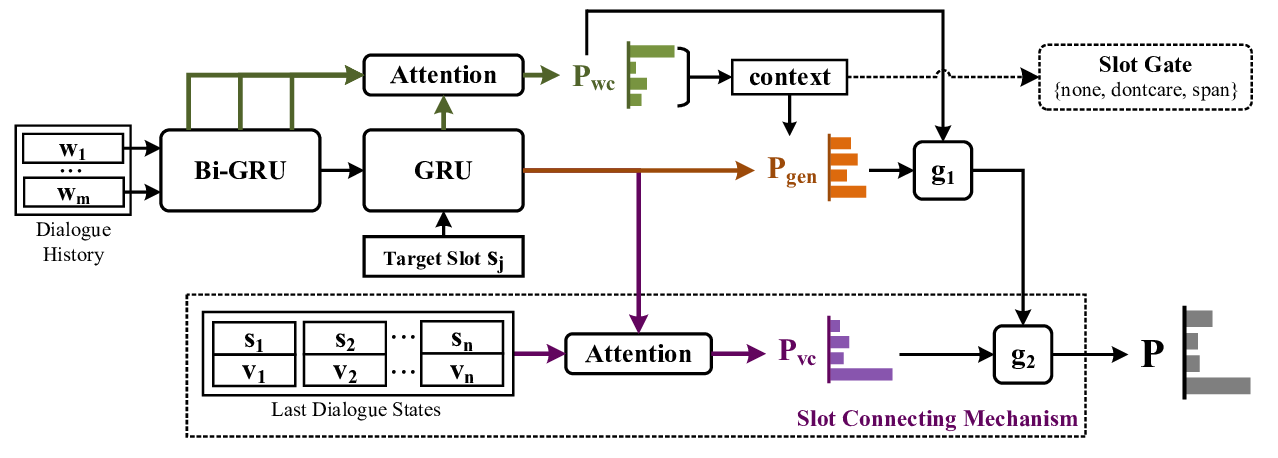
Improving Chinese Word Segmentation with Wordhood Memory Networks
Yuanhe Tian, Yan Song, Fei Xia, Tong Zhang, Yonggang Wang,
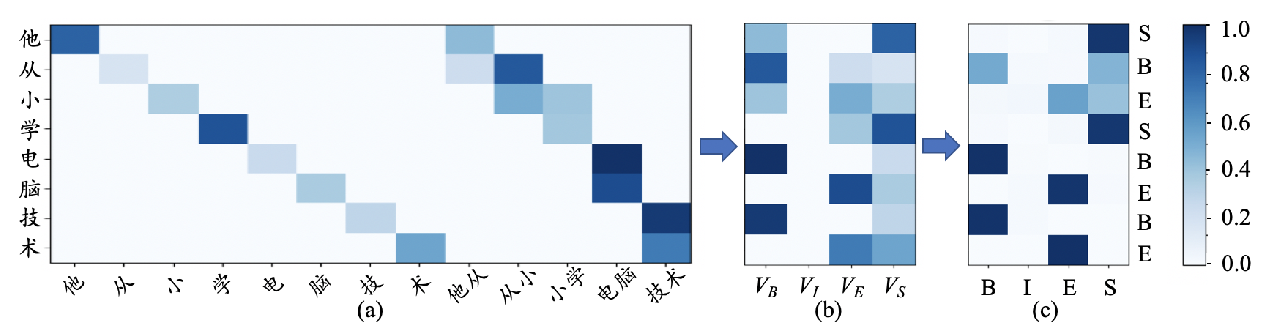
Joint Chinese Word Segmentation and Part-of-speech Tagging via Two-way Attentions of Auto-analyzed Knowledge
Yuanhe Tian, Yan Song, Xiang Ao, Fei Xia, Xiaojun Quan, Tong Zhang, Yonggang Wang,
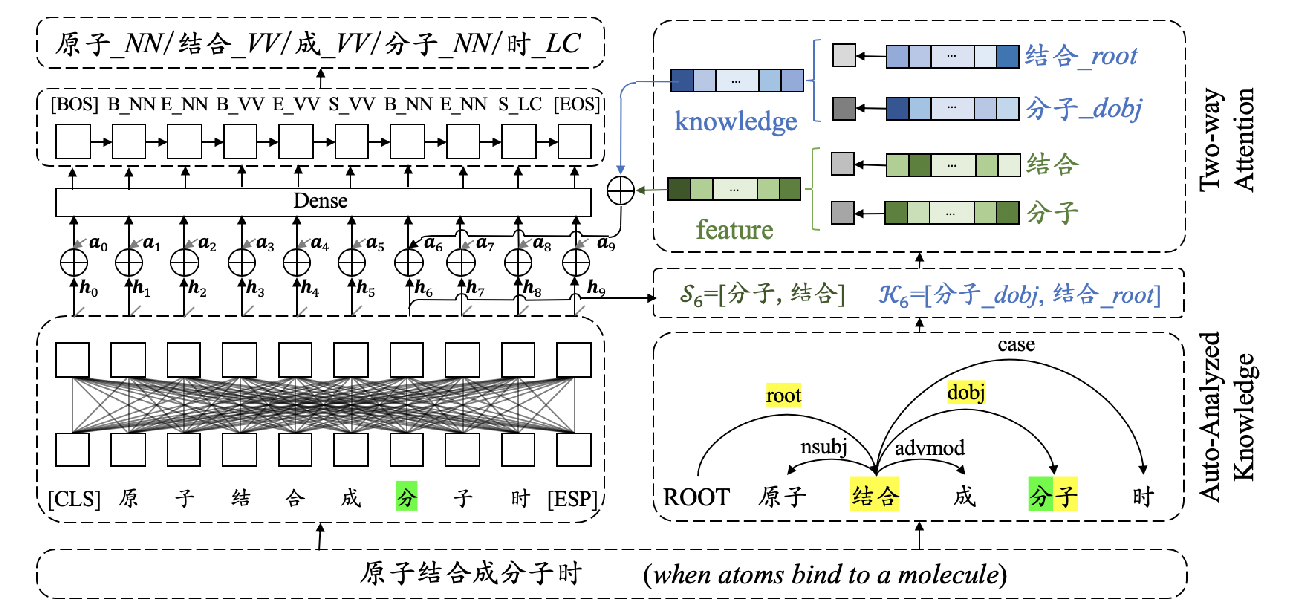